Combating Bias in AI for Health Care Equity
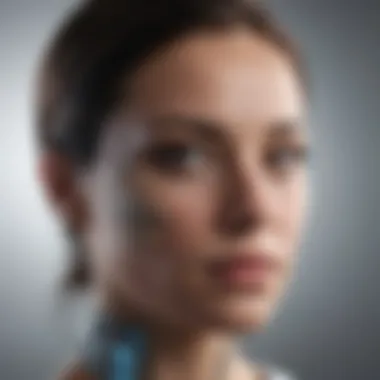
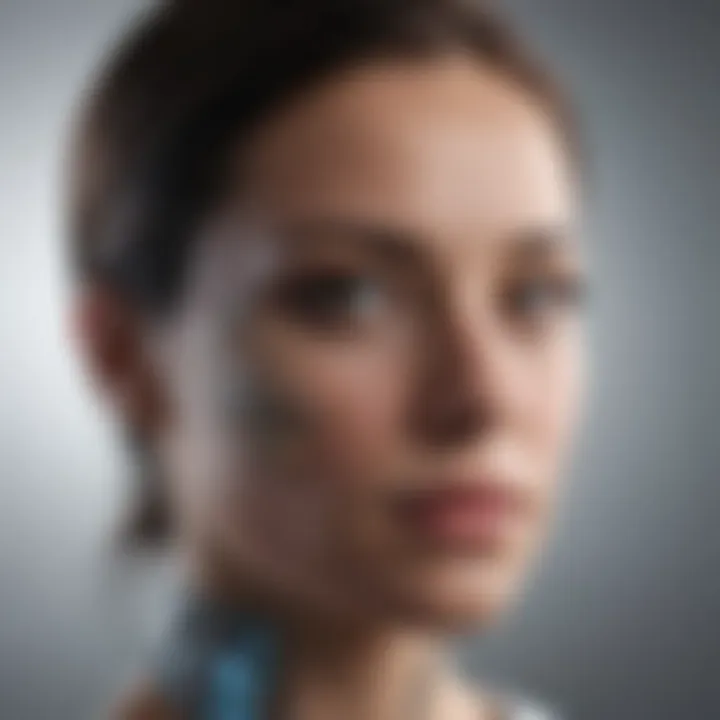
Intro
In the rapidly evolving field of health care, artificial intelligence (AI) is becoming an indispensable ally. However, lurking beneath its promise lies a significant challenge: bias. This isn't the same bias one might find in a casual conversation; it's a deep-seated issue that can lead to unequal treatment and poor health outcomes. The stakes are high, and recognizing the origins and implications of this bias is crucial for both practitioners and patients alike.
As we embark on this discussion, we must consider not only how bias manifests in AI systems but also how it influences the decisions made in healthcare settings. The algorithms designed to assist in diagnosing ailments, predicting patient outcomes, or recommending treatments can inadvertently perpetuate existing disparities if they are built on flawed data. In essence, AI reflects the world around it, including ingrained prejudices and structural inequalities, which can endanger lives when it matters most.
Understanding this complex landscape goes beyond a mere academic exercise; it necessitates a commitment to ethical responsibility. By exploring the origins of bias, its impact on patient care, and potential strategies for ensuring fairness, we can begin to chart a clearer path toward equitable healthcare AI.
Ultimately, addressing bias in AI isn't just about improving technology; it's about fostering a health care system where everyone, regardless of their background, has fair access to quality medical care.
Prolusion
In an age where technology is accelerating faster than a sports car on a straight highway, the role of artificial intelligence in healthcare cannot be overstated. This isn't just a collection of algorithms crunching numbers; it’s about improving patient outcomes, optimizing resources, and fundamentally reshaping how healthcare systems operate. However, as we delve deeper into the digital frontier, a pressing issue looms like a shadow: bias in AI systems. This article takes a close look at the multi-faceted problem of bias—how it sneaks into AI algorithms, what impacts it has on health outcomes, and the ethical quandaries it raises.
Understanding how bias seeps into the very fabric of AI systems is essential. It touches everyone from patients to practitioners, affecting diagnostics, treatment plans, and even the overarching trust in healthcare. In a world where the stakes are high, ensuring fairness and equity is not just idealistic; it is imperative. Addressing this issue is not merely about correcting data sets or tweaking algorithms. It’s about recognizing that the decisions made by AI can carry deep implications for individuals and communities alike.
The Rise of Artificial Intelligence in Health Care
The integration of artificial intelligence into healthcare settings has been nothing short of revolutionary. As hospitals and clinics adopt these advanced technologies, they face the challenge of harnessing AI’s potential while guarding against inherent biases that could compromise care. AI applications range from diagnosing diseases through imaging technologies to individualized patient treatment suggestions. For instance, tools like IBM Watson Health have showcased the transformative capabilities of AI in analyzing complex datasets to assist in cancer treatment planning. Yet with remarkable advancements come remarkable responsibilities.
One can't ignore the stark contrast between the promise of AI and the reality of its implementation. Notably, studies indicate that many AI systems have been trained on datasets that lack diversity, leaving vulnerable populations at a disadvantage. A clear example is how facial recognition images tend to misidentify individuals from racial or ethnic minorities compared to their white counterparts. Ultimately, when AI systems are trained on a narrow slice of the population, they create outcomes that may not only be inaccurate but downright harmful.
Understanding Bias in AI Systems
Bias in AI systems is often a cocktail of human, algorithmic, and data-driven influences. Take human bias, for instance; despite being well-intentioned, the biases of developers can infiltrate AI systems. This can manifest when programmers unknowingly encode their subjective beliefs into algorithms.
On the data front, if the training datasets predominantly feature data from a particular demographic, the resulting AI model will reflect these imbalances. Such skewed representations hinder effective treatments and recommendations for those who fall outside the represented group. Algorithmic bias additionally arises from the very nature of machine learning, where algorithms can self-learn and unintentionally amplify existing biases present in the training data—like adding fuel to a smoldering fire.
Understanding these nuances is the first step toward tragic consequences of biased AI systems in healthcare. As we continue to integrate AI into clinical practice, raising awareness and fostering scrutiny about bias is not just good practice; it is an absolute necessity for the future of equitable healthcare.
Types of Bias in AI
Bias in artificial intelligence isn't merely a technical issue; it’s a matter of ethical implications, particularly in health care where lives are on the line. Understanding the various types of bias that can arise in AI systems is crucial for developing solutions that foster equity in health outcomes. By dissecting the underlying elements, benefits, and considerations surrounding bias, we can pave the way for advancements that prioritize patient welfare and dignity.
Sampling Bias
Sampling bias refers to the distortion that occurs when certain groups are underrepresented or overrepresented in the data used to train AI models. This can lead to skewed results that do not accurately reflect the population. For instance, if a model is trained predominantly on data from a specific demographic—say, middle-aged white males—it might fail to perform effectively for women or racial minorities. In health care, this can have dire consequences, such as misdiagnosis or inadequate treatment recommendations for patients outside the training spectrum.
"A good sample makes all the difference; if you're not getting a full picture, you're bound to miss something crucial."
By recognizing the risks of sampling bias, developers can strive for a more inclusive data set that better represents the diversity of the population needing care. This move not only enhances the accuracy of AI diagnostics but also promotes trust among underrepresented groups, ultimately leading to improved health outcomes across the board.
Algorithmic Bias
Algorithmic bias is another layer of complexity, occurring when the algorithms themselves operate based on flawed assumptions or parameters that perpetuate existing disparities. For example, consider an AI system designed to predict patient risks for conditions like heart disease. If the algorithms weigh certain risk factors disproportionately—ignoring crucial variables that affect women or younger patients—it can unjustly categorize these individuals as low-risk, potentially leading to a lack of necessary interventions.
The significance of algorithmic bias calls for rigorous testing and refinement of models before deployment in real clinical settings. Continual learning from feedback loops can address flaws, ensuring algorithms are responsive to diverse patient needs.
Human Bias
Human bias is perhaps the most insidious of these biases, manifested during data collection, programming, and interpretation of results. The subjective nature of human decision-making can introduce unintentional biases in how data is handled. If those involved in developing AI systems possess their own biases—whether conscious or not—it can wrap itself into the algorithms, tainting the outputs.
An illustration of this can be found where clinicians trained on biased historical data unintentionally pass along these biases when interpreting AI outputs, thus perpetuating a cycle. To combat human bias, organizations must implement robust training programs focusing on awareness and mitigation strategies. Encouraging an environment of diversity not only among the algorithms but also within teams can be transformative in curbing biased outcomes.
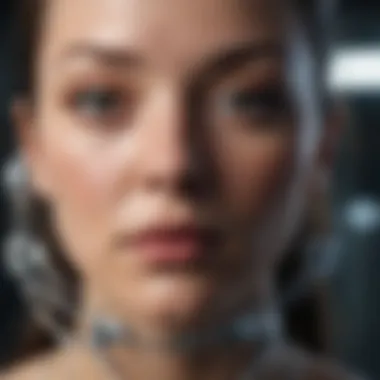
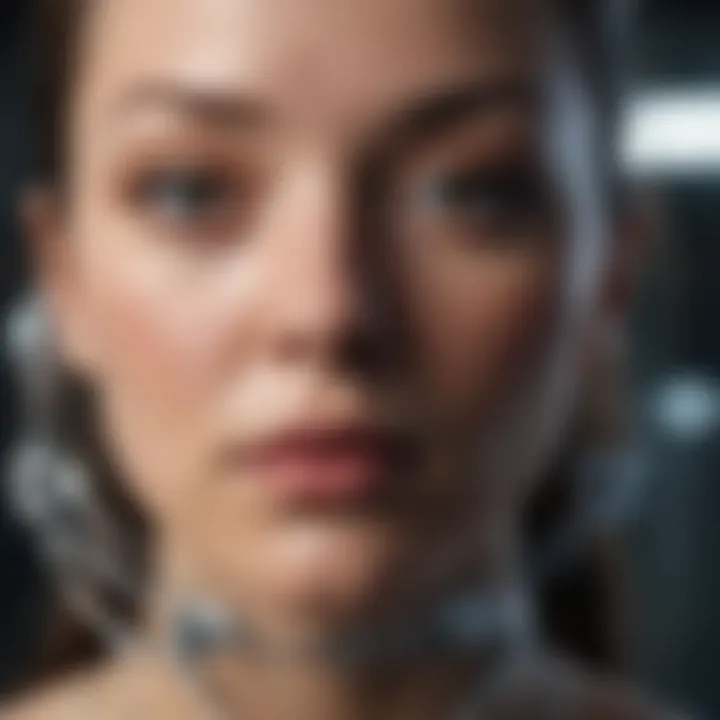
In essence, understanding types of bias in AI is pivotal in addressing potential pitfalls in health care applications. By improving data inclusiveness, scrutinizing algorithms, and fostering a culture of awareness regarding human bias, stakeholders can work collectively toward a more equitable healthcare system that truly reflects and serves the varied needs of all patients.
Sources of Bias in AI Health Care Applications
Understanding the sources of bias in AI applications within health care is crucial in ensuring that artificial intelligence contributes positively and equitably to medical practice. These sources play a significant role in how AI systems function, impacting everything from patient outcomes to public trust in technology and medical professionals. Identifying the biases embedded in these systems allows stakeholders to implement strategic actions toward enhancing fairness and transparency in health care.
Data Collection Practices
Data collection is the bedrock upon which AI algorithms are built. If the data gathered is skewed or unrepresentative, the outcomes will likely reflect that bias. For instance, if a healthcare system predominantly gathers data from a single demographic, the AI trained on this data may not adequately serve underrepresented groups. This uneven representation can lead to misdiagnoses or ineffective treatment recommendations.
Moreover, methods of data collection can introduce additional bias. Consider, for example, how health records are created. Older systems often rely on manual data entry, which is susceptible to human errors or biased interpretations of patient conditions. Inconsistent data collection methods, such as those using clinical intuition versus structured questionnaires, can further complicate the comprehensiveness of the data pool.
To mitigate these risks, medical professionals and researchers must prioritize rigorous data collection processes that encompass a broad range of populations and conditions. Striving for diversity in data not only enhances the model's accuracy but also builds a foundation of trust within different communities.
Model Training Processes
Once data is collected, the model training phase begins. This stage is where specific algorithms are taught to learn and replicate patterns based on the data given. If the training data reflects historical inequities or health disparities, the model will inherently learn to operate within those same biases. For instance, if a model is trained predominantly on data from one ethnic group, it may underperform when assessing conditions in others, potentially leading to serious health implications.
Furthermore, the selection of algorithms plays a large part as well. Some algorithms may inadvertently prioritize certain types of data, further amplifying existing biases. There is also the risk of overfitting, where an AI model becomes too familiar with its training data, resulting in skewed predictions when it encounters real-world cases that deviate from its narrow scope of training.
To address these issues, researchers can implement techniques to ensure more balanced learning—for example, by incorporating fairness metrics during the training phase, which can help monitor and correct for biases before they propagate into operational use.
Deployment and Use in Real Settings
The phase of deploying AI systems into real-world health care settings is where biases can manifest in the most harmful ways if not properly addressed. Even the most well-designed AI systems can falter in practical applications if the context in which they are implemented does not match the scenarios their training was based upon.
An example might include a diagnostic application that was tested in urban hospitals with diverse patient populations but is later used in a rural clinic serving a much homogenous group. Here, the assumptions made during the algorithm’s training may not hold, leading to misdiagnoses or suboptimal treatment recommendations. This discrepancy can exacerbate existing health inequities, further marginalizing certain populations.
Additionally, users of AI systems—the healthcare providers—play a pivotal role in bridging gaps. Their interpretations and trust in AI recommendations can shift based on their own biases and experiences. Training health care professionals on the limitations and strengths of these AI tools becomes essential in establishing a collaborative environment that prioritizes patient welfare over technology reliance.
Impact of Bias on Health Outcomes
The impact of bias in artificial intelligence within health care is a matter of profound significance. Bias can distort not only the functionality of AI systems but also the very essence of patient care. When certain populations are consistently misrepresented or overlooked, the medical decisions guided by AI can perpetuate health inequities, affecting outcomes across demographics. Understanding this impact is crucial. Improving diagnostic accuracy and treatment recommendations hinges on addressing these biases. By ensuring AI systems are unbiased, health outcomes can improve and become more equitable for all patients.
Disparities in Diagnostic Accuracy
Disparities in diagnostic accuracy highlight the stark reality of health care bias. Imagine two patients presenting similar symptoms — one a white male, the other an African American female. If AI tools trained on predominantly white data sets offer different predictive outputs, the result could lead to misdiagnosis. Misdiagnoses can have cascading effects, delaying necessary treatments for some while encouraging unnecessary interventions for others.
This phenomenon isn't just theoretical. In practical terms, studies have identified that certain AI-driven diagnostic tools are less accurate in detecting conditions like heart disease in women compared to men. The model lacks a comprehensive understanding because the data used in training was not inclusive enough. As a result, the diagnostic capabilities of these AI systems falter, leading to real-world implications for patient health.
"The true measure of an AI model's effectiveness lies not just in its algorithms, but its training data. Bias, if left unchecked, can lead to inadequate care."
Effects on Treatment Recommendations
Treatment recommendations also suffer from biased AI systems. When algorithms prioritize data from certain ethnic groups, it can lead to unequal treatment strategies. Consider the treatment protocol for diabetes management — if an AI system predominantly analyzes white populations, then the recommendations it provides may not consider the unique genetic factors influencing diabetes in other racial groups.
Such limitations can affect the effectiveness of prescribed treatments. One study showed that patients treated with recommendations based on biased AI had poorer health outcomes compared to those whose treatments were guided by more representative data sets. It emphasizes the critical need to refine algorithms and incorporate diverse datasets to promote effective treatment for everyone, regardless of their background.
Patient Trust and Engagement
Lastly, the concept of patient trust cannot be overlooked when discussing the impact of bias. If people feel that the technology guiding their health care is biased, their willingness to engage with the medical system diminishes. Imagine a patient who has observed repeated patterns of bias in their care — they may go elsewhere, seeking human judgment over algorithmic suggestions.
Research indicates that trust in AI systems is closely tied to transparency in how decisions are made. If patients believe that AI systems treat them unfairly, not only does it risk their health, but it also hampers the entire patient-care provider relationship. Trust is fundamental, as it encourages patients to share vital information, leading to more comprehensive care approaches.
Ethical Considerations
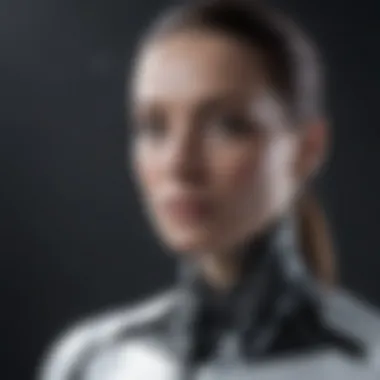
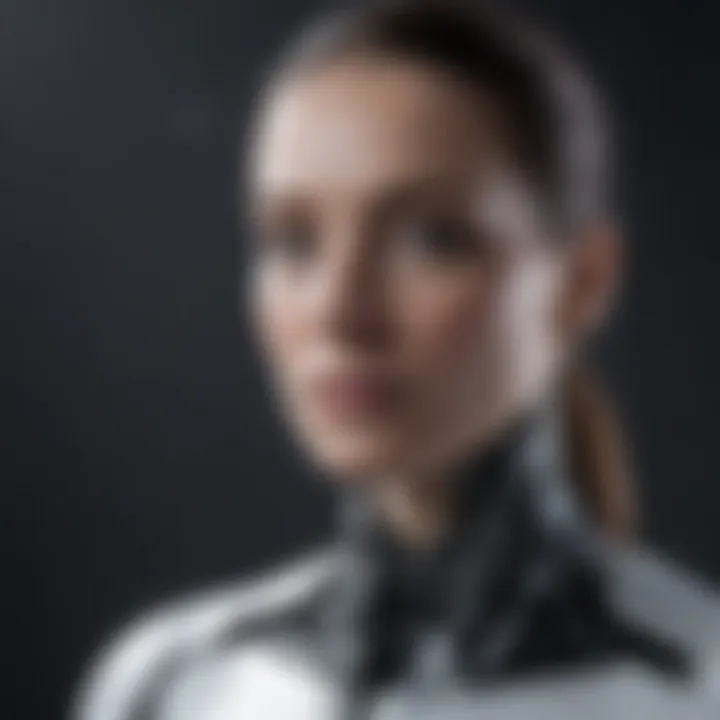
The evolution of artificial intelligence in health care has ignited critical discussions around ethical considerations. At its core, these discussions address the moral obligations we have toward patients and the potential consequences of using biased AI systems. Understanding ethical considerations isn’t merely academic; it’s essential for a trustworthy health care system. Ensuring that AI serves its purpose effectively and equitably means prioritizing ethics in every step of the design, deployment, and evaluation processes.
Informed Consent and Autonomy
Informed consent stands as a pillar of medical ethics. When AI systems are integrated into health care, the question arises: how much do patients understand about these technologies? Many patients might not be aware of how algorithms influence their treatment plans and decisions. They may simply trust the system, assuming it operates with their best interests in mind. Thus, it’s imperative to communicate clearly what the algorithms do and how their data is being used.
- Transparency is Key: Health care providers should disclose how AI systems function, what data is used, and what implications the algorithms may have for patient care. This transparency helps patients feel empowered, ensuring they can make informed choices about their treatment.
- Preserving Autonomy: Patients should retain the ability to question, modify, or withdraw consent without fear of losing out on quality care. Autonomy, a fundamental ethical principle, must be preserved even in an AI-enhanced care model.
By reframing the narrative around informed consent to emphasize patient understanding and autonomy, health care entities can foster trust and promote a more ethical approach to AI.
Accountability and Liability
As artificial intelligence becomes intertwined with clinical decision-making, the question of accountability emerges. Who is responsible if an AI system causes harm? Accountability structures are crucial. Yet, they remain murky. If an algorithm misdiagnoses a condition due to flawed data, the consequences can be dire. The patients, whose lives hinge on these diagnoses, often bear the brunt of these failings.
- Assigning Responsibility: Clear guidelines should outline whether liability lies with the developers of the AI, the health care institutions deploying it, or perhaps even the regulators overseeing its use. Without clarity on this front, patients may find it challenging to seek recourse when negligent algorithms lead to subpar care.
- Building Trust: Establishing systems of accountability also builds trust in AI systems. Patients need assurance that, should something go awry, they have pathways for redress. This obstacle isn’t just practical; it speaks to the ethical obligation we have toward individuals seeking care.
Equity and Justice
The ethical implications of AI go beyond transparency and accountability; they hinge on broader questions of equity and justice. Health disparities can be perpetuated through biased AI systems. If these systems are trained on data sets that lack diversity, they may fail to serve marginalized groups adequately.
- Addressing Disparities: Equity mandates that AI applications be developed with an inclusive mindset. Diverse datasets should reflect the populations served. Otherwise, the technology may simply reinforce existing biases within health care systems.
- Social Responsibility: It falls upon developers, health care providers, and policymakers to advocate for justice in health care AI. The moral obligation extends to ensuring that innovations in technology help bridge gaps rather than widen them. This commitment to equity must drive every decision made in the design and deployment of AI tools.
In summary, prioritizing ethical considerations in AI development and its application in health care is not optional; it is a necessity. From informed consent to accountability and equity, every facet deserves our rigorous attention. By making ethics a cornerstone, we can help ensure the technology serves everyone fairly.
Strategies for Mitigating Bias
Addressing bias in artificial intelligence is not just a suggestion; it is a necessity. In health care, where lives hang in the balance, ensuring that AI systems operate fairly and equitably is paramount. The strategies put forth here are not merely theoretical; they form the bedrock of creating systems that promote inclusivity and fairness. By focusing on these strategies, practitioners, developers, and policymakers can actively reduce bias in health-related AI applications, leading to better health outcomes for all.
Improving Data Inclusiveness
The foundation of any AI system is its data. If the input data is skewed or unrepresentative, the resulting algorithms may perpetuate or even amplify existing disparities. Therefore, improving data inclusiveness becomes crucial. This means gathering diverse data from various demographic groups to ensure that all voices are heard. To achieve this, organizations should look for a variety of data sources, such as:
- Surveys and health records from a range of facilities, including those in underserved communities.
- Collaborations with community organizations to understand localized health issues better.
Utilizing technologies like crowdsourcing can also help broaden data input.
Additionally, continually monitoring and updating data sets is essential to reflect current demographic changes.
Enhancing Algorithmic Transparency
Another critical aspect is enhancing transparency in AI algorithms. When stakeholders—including patients, health care providers, and regulatory bodies—understand how an algorithm makes its decisions, it builds trust and fosters collaboration. There are a few ways to enhance this transparency:
- Documentation: Clearly outline the data sources, model assumptions, and decision-making processes.
- Open-source software: Encourage the use of community-driven platforms where algorithms can be accessed and scrutinized by others.
By demystifying how AI works, we can better prevent biases that may arise from opaque processes.
"Transparency is the currency of trust in the digital age."
Implementing Regular Audits
Conducting regular audits of AI tools is akin to regular health check-ups; they help catch potential issues before they escalate. These audits should focus on assessing the performance of algorithms in various demographics. Steps to implement audits include:
- Establishing a bi-annual or quarterly audit schedule.
- Analyzing outcomes across demographics to identify discrepancies.
- Adjusting algorithms based on audit findings to continuously improve performance.
Regular audits will not only help detect deficiencies but also validate improvements over time. This proactive approach can significantly mitigate bias risks.
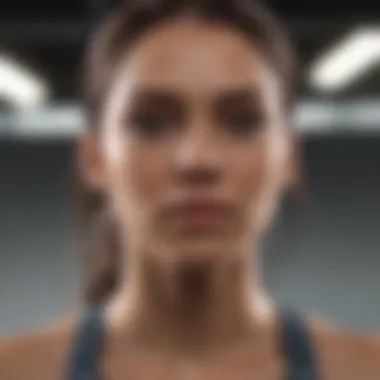
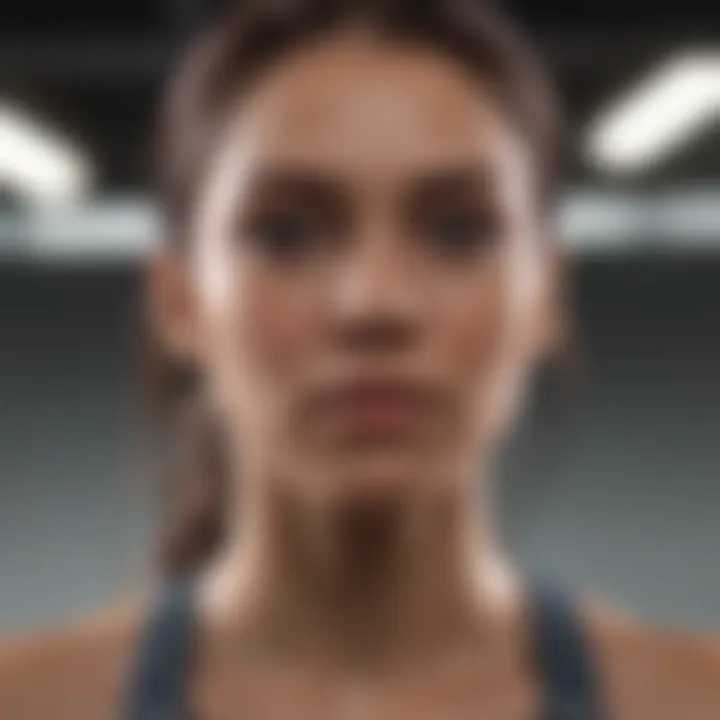
Promoting Ethical AI Development
Finally, fostering an environment that prioritizes ethical AI development is paramount. Developers should engage in ethical discussions at every phase of development, asking tough questions about the potential impacts of their work. Some strategies to promote an ethical culture in AI development include:
- Training and awareness programs: Educate developers on biases and their consequences in health care.
- Engagement with ethicists: Collaborate with ethicists to ensure that ethical standards are embedded into the design process.
This commitment to ethical guidance can serve as a compass, helping navigate the complex relationship between AI and health care.
Future Directions
The role of future directions in addressing bias in artificial intelligence within health care cannot be overstated. As the field of AI continues to evolve, understanding how to shape its trajectory is crucial for ensuring equity and effectiveness. Future directions point towards advancements that could not only ameliorate existing biases but also set a precedent for a more inclusive approach in health care applications.
Key considerations include an emphasis on innovative research initiatives, solid policy recommendations, and the importance of collaboration among stakeholders. Each of these elements brings its own benefits, fostering a more robust framework for mitigating bias in AI systems.
Research Initiatives
When discussing future directions, research initiatives stand at the forefront. There is a pressing need for comprehensive studies that delve deep into the mechanisms contributing to bias in AI. Research should focus not all on identifying what the biases are but also on how they emerge in different contexts. Exploring diverse datasets can help unearth nuanced patterns that conventional studies often overlook.
A few focused areas could include:
- Longitudinal Studies: Analyzing long-term effects of biased AI outcomes on various populations can reveal disparities over time.
- Interdisciplinary Approaches: Collaborating with social scientists, ethicists, and technologists can foster innovative solutions.
- User-Centered Research: Gathering input from affected communities can provide critical insights into real-world implications of AI decisions.
Investing time and resources into these initiatives will lay a solid foundation for developing more accurate, equitable AI models that prioritize patient care without bias.
Policy Recommendations
Adopting sound policy recommendations is essential for guiding the implementation of AI in health care. Policymakers need to craft regulations that do not just react to biases after they occur but proactively work to prevent them from surfacing. Some potential policies might involve:
- Data Governance Frameworks: Establishing clear guidelines on how data is collected, processed, and utilized.
- Mandated Bias Audits: Requiring routine evaluations of AI systems to assess their performance across demographic groups and ensure fairness.
- Funding for Inclusive Research: Allocating resources towards studies that aim to include underrepresented populations in health data sets.
These policies not only safeguard against potential misuse but also encourage a culture of accountability in AI development. An effective regulatory approach can build public confidence, ensuring that technology serves all patients equally.
Collaboration Among Stakeholders
Lastly, collaboration among stakeholders is paramount for fostering a more equitable AI environment in health care. Partnerships between tech companies, healthcare professionals, governmental bodies, and patient advocacy groups can drive significant progress. For instance, establishing multi-disciplinary task forces can facilitate knowledge-sharing and collective problem-solving.
Effective collaboration can result in:
- Shared Best Practices: Organizations can learn from each other's successes and failures, minimizing redundant efforts and streamlining processes.
- Enhanced Accountability: Cooperative efforts can introduce checks and balances, ensuring that all stakeholders are held responsible for bias mitigation.
- Cross-Sector Innovation: Insights from various fields can lead to groundbreaking advancements in AI that are reflective of diverse needs.
"The battle against bias in artificial intelligence can only be fought through a united front; it requires the collective effort of every player in the healthcare ecosystem."
Epilogue
In considering the intricate relationship between artificial intelligence and healthcare, it becomes evident that addressing bias is not merely an optional endeavor; it is a pressing necessity. The implications of biased AI systems can reverberate far beyond mere statistics; they touch the lives of individuals, shaping their healthcare experiences, and outcomes. By exploring various dimensions of bias throughout this article, including its origins and influences in data collection and model training, we have gained critical insights that illuminate the path toward a more equitable healthcare future.
Summarizing Key Insights
To encapsulate the findings and discussions in this article, several key insights emerge:
- Awareness of Bias: Recognizing the presence of bias in AI is the first step in tackling this pervasive issue. AI systems, although powerful, are only as good as the data they are trained on, thus acknowledging the potential sources of bias is crucial.
- Impact on Outcomes: We have seen how bias infiltrates diagnostic accuracy, treatment recommendations, and even the establishment of patient trust. Each facet of AI in healthcare is interconnected, and even minor biases can lead to significant disparities in care.
- Need for Ethical Practices: Emphasizing ethical considerations, such as informed consent and accountability, remains paramount as we move forward with AI. Stakeholders must prioritize these elements to foster an environment where healthcare technology serves all equitably.
- Strategies for Improvement: A variety of strategies, such as enhancing data inclusiveness and algorithmic transparency, can be employed to combat bias effectively. Ongoing auditing and research initiatives will also serve as significant tools in this fight.
Call to Action for Stakeholders
The need for action cannot be overstated. All stakeholders—healthcare providers, technology developers, regulatory bodies, and even patients—must join forces to advocate for change. Here are tangible steps they can take:
- Invest in Research: Prioritize funding in research initiatives that examine and combat biases in AI. Understanding how biases arise and persist is essential for developing effective solutions.
- Establish Policies: Create comprehensive policies that hold organizations accountable for their AI systems. Implement guidelines that ensure fairness and equity in all AI applications.
- Engage the Community: Foster discussions within the community about the ethical implications of AI. Awareness is key. Patient engagement in these dialogues can lead to better healthcare practices and outcomes.
"AI is not just a tool; it's a partner in care. We must ensure that partnership is grounded in fairness and respect for every individual."
- Promote Transparency: Encourage transparency in AI algorithms. Patients and health professionals deserve to know how decisions are made, fostering trust and understanding in AI applications.