Exploring the Synergy of AI and Biology
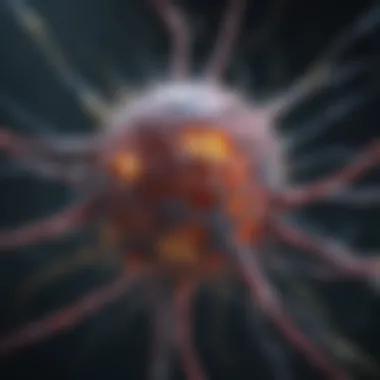
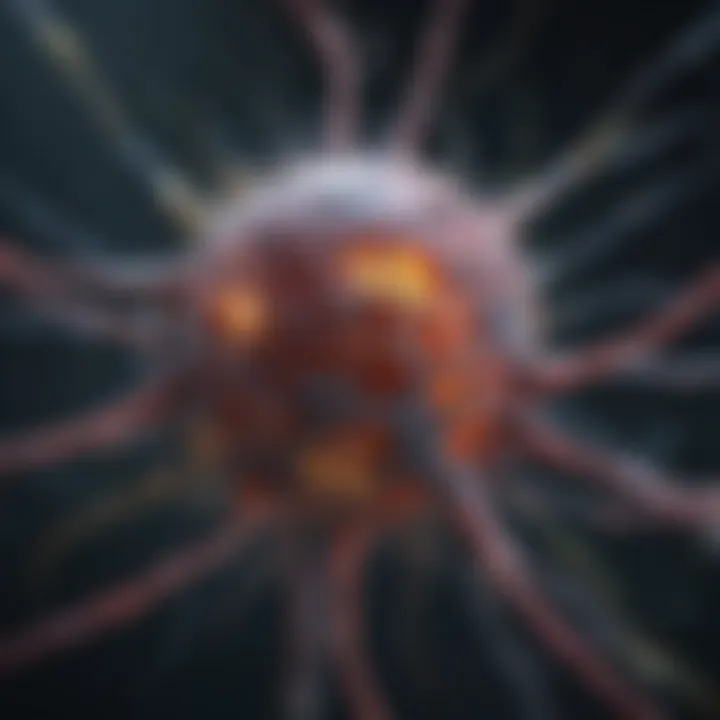
Intro
The convergence of artificial intelligence (AI) and biology is not just a passing trend; it is a profound shift in how we understand life itself. The symbiosis of these two fields paves the way for breakthroughs that are shaping modern medicine, environmental science, and even our understanding of genetics. As innovations march forward, we find ourselves in an age where computational methods are no longer just tools but crucial components in biological research. This article sets off on an exploratory journey, shedding light on the pivotal ways AI is transforming the biological landscape while also dipping into the ethical questions that arise from such powerful technologies.
Research Highlights
Overview of Key Findings
- AI in Genetics: In recent years, machine learning techniques, particularly deep learning, have made significant strides in genomic analysis. These algorithms can decipher complex genetic sequences faster and more accurately than traditional methods. For instance, researchers are using AI to identify mutations linked to hereditary conditions, enabling earlier diagnoses and tailored treatments.
- Drug Discovery: AI is proving essential in drug development. For example, companies like Atomwise are harnessing AI to predict how different compounds will interact with biological targets. This not only speeds up the discovery process but also reduces costs significantlyāa game changer for the pharmaceutical industry.
- Ecosystem Management: By utilizing AI to analyze vast datasets, ecologists can better monitor ecosystems. Predictive models can enhance conservation strategies, providing a clearer picture of how climate change is affecting biodiversity.
Significance of the Research
AIās integration into biology holds immense significance. Not only does it deepen our understanding of complex systems, but it also provides practical solutions to pressing global challenges such as healthcare accessibility and environmental sustainability. The promise of personalized medicine, informed by genomic insights analyzed through AI, may shift healthcare paradigms entirely. Likewise, enhancing ecological resilience through AI-driven insights could lead to more sustainable human practices in harmony with nature.
"Artificial intelligence serves as both the magnifying glass and the compass in biological exploration, illuminating pathways and guiding researchers through the intricacies of life processes."
Ethical Considerations
As we delve deeper into the relationship between AI and biology, it is crucial to navigate the ethical landscape. Questions arise about data privacy in genomic studies, the potential for bias in algorithms, and the overarching implications of AI-driven decisions in real-world applications. Striking a balance between innovation and ethics will be critical in ensuring that advancements serve humanity in a just manner.
Culmination
Preamble
The interplay between artificial intelligence (AI) and biology is a topic that cannot be overlooked in today's rapidly advancing technological landscape. As AI technologies evolve, they are increasingly being applied to biological systems, leading to significant breakthroughs that change our understanding of life itself. This melding of disciplines is crucial for several reasons, including its potential to enhance research efficiency, improve the accuracy of predictions, and accelerate the pace at which scientific discoveries are made.
In biological research, the sheer volume and complexity of data present challenges that often stymie traditional analytical methods. AI, with its ability to process massive datasets and identify patterns, offers tools that can unravel intricate biological processes. In applying concepts from AI, researchers are forging paths to tackle diseases, understand genetic makeups, and develop innovative drugs, making such synergy not just helpful, but vital.
"The future will belong to the curious. Those who are curious about AI and biology will drive the next wave of innovation."
Understanding how these technologies converge opens up avenues to push boundaries further, leading to discoveries that were previously mere speculation. As we explore the significance of AI in biology, it is essential to recognize that each step taken in this journey is a step toward revolutionizing our comprehension and treatment of various biological phenomena.
Understanding the Convergence
The convergence of AI and biology is not simply a trend; it reflects deep-rooted needs within research communities to leverage the rapid advancements in compute power and algorithmics. The computation abilities of AI enable the handling of biological data that is not just large in size but also diverse in types. From genomic sequences to protein structures, each category of data has unique characteristics, which AI methods can discern and analyze more effectively than conventional techniques.
Machine learning, a subset of AI, exemplifies this fit perfectly. It utilizes models that can learn from data and make decisions or predictions without being explicitly programmed for each task. In the realm of biology, this becomes invaluable. For instance, researchers can employ these models to predict disease progression based on genetic markers or understand how various environmental factors affect an organism's health.
As we dive deeper into specific applications, it becomes evident that the benefits are manifold. AI doesn't just optimize biological research; it transforms the very fabric of scientific exploration. This intersection challenges the status quo of research methodologies, urging scientists to adopt more holistic, interdisciplinary approaches.
Scope and Objectives
The scope of this exploration encompasses a variety of fields where AI influences biology, such as genomics, bioinformatics, drug discovery, and ecological management. Each application reveals distinct objectives that drive scientists and researchers toward integrating these technologies in their work.
- Genomic Sequencing: Harnessing AI algorithms to interpret sequence data swiftly and accurately, paving the way for personalized medicine.
- Drug Discovery: Streamlining pharmaceutical development through predictive modeling that reduces time and cost.
- Ecosystem Management: Utilizing AI to enhance conservation efforts and monitor biodiversity effectively.
- Ethics and Society: Understanding and addressing data privacy concerns and algorithmic biases that can arise in these intersections.
Ultimately, the objectives can be framed into two dominant strands: optimizing existing biological research workflows and exploring new frontiers that may bring about novel insights or therapies. As researchers hone in on these goals, the benefits wrought from this interplay might yield unprecedented advancements in curing diseases, conserving species, and understanding complex biological interactions.
By engaging with these challenges and exploring the relationship, one cultivates a fertile ground for both academic inquiry and practical applications that impact society.
Foundations of Artificial Intelligence
Understanding the foundations of artificial intelligence (AI) is paramount for anyone diving into the interconnected world of AI and biology. This exploration is not merely an academic exercise; itās a necessary foundation for future advancements that will redefine how we approach biological research, healthcare, and ecological management. The significance of foundational concepts in AI cannot be overstated, as they serve as the bedrock upon which all subsequent innovations are built.
AI isn't a monolithic entity. Rather, it consists of various branches and methodologies. By demystifying these core concepts, we can better appreciate how AI can revolutionize not just biology, but all scientific disciplines. The implications are as vast as they are profound: enhanced data analysis, predictive modeling, and automation of biological processes are just the tip of the iceberg.
Basic Concepts in AI
When we talk about the basic concepts of AI, we are looking at the fundamental principles that guide its operation. This includes understanding the distinction between various types of AI, such as narrow AI, which specializes in narrow tasks and is often seen in applications like image recognition, versus general AI, which aspires to perform any intellectual task a human can do.
- Narrow AI: This is what most current AI applications fall under. For instance, consider how Netflixās algorithm recommends shows based on your viewing history. It's tailored, effective, but specialized.
- General AI: While still theoretical, general AI would involve machines capable of understanding or learning any intellectual task that a human being can do. For now, it remains a tantalizing possibility on the horizon.
Understanding these types sets the stage for deeper discussions on how AI is applied to biological systems. As researchers grapple with increasingly complex biological data, their grip on AIās basic definitions and capabilities is essential for innovative solutions.
Machine Learning and Deep Learning
Diving deeper, we encounter machine learning and deep learning, both of which are subsets of AI that drive much of the current advancement in biological research.
Machine Learning (ML) is fundamentally about teaching machines to learn from data. For example, in genetics, ML can analyze vast datasets of genomic information to identify patterns or make predictions about genetic diseases. The key here is that the machine improves its performance over time as it ingests more data.
On the other hand, Deep Learning (DL) takes things a notch higher by utilizing artificial neural networks that mimic the human brainās architecture. This method excels at handling unstructured data, like images or sequences. Picture a laboratory using DL to identify cancerous cells in histopathology images. With thousands of images processed, the AI model learns the nuances of what makes a cell cancerous, drastically improving diagnostic accuracy.
As technology evolves, understanding the foundational principles of AI becomes increasingly crucial to solve complex biological challenges.
The synergy of Machine Learning and Deep Learning presents a double-edged sword that scientists can wield to enhance accuracy, efficiency, and innovative breakthroughs in the life sciences. These AI elements serve not only as tools but also as catalysts for a new era in biological research, eventually guiding the next generation of scientists toward unexplored territories.
Biological Data and AI
The integration of biological data with artificial intelligence is not just a trend; it's a revolution that's fundamentally reshaping how we understand life itself. As we venture into this increasingly data-driven era, recognizing the significance of biological data becomes paramount for students, researchers, and professionals alike. This is because biological data encompasses a wide range of information, from DNA sequences to protein structures, and even ecological data, all of which are pivotal for advancing our understanding of biology through AI.
The potential benefits of merging this data with AI are astounding. For instance, in genetics, AI algorithms can sift through immense genomic data sets, uncovering patterns and mutations that might escape human eyes. This meticulous interpretation lays the groundwork for precision medicine, where treatments can be tailored to individual genetic profiles, enhancing outcomes significantly.
Moreover, AI empowers bioinformatics, facilitating not just the storage but also the analysis of extensive biological databases. With sophisticated algorithms, researchers can perform complex analyses in mere seconds, a task that would otherwise take considerable time and human resources. This efficiency pushes the frontiers of discovery, accelerates research timelines, and opens up volumes of data that could lead to groundbreaking findings. In short, the symbiosis of biological data and AI stands as a beacon of innovation in scientific exploration.
Types of Biological Data
When we delve into biological data, it's crucial to categorize it to comprehend its vastness and applicability. Here are the primary types:
- Genomic Data: This includes sequences of DNA, RNA, and the mapping of entire genomes. Such data is foundational to genetic research and therapy development.
- Proteomic Data: Focused on the study of proteins, their structures, and functions, proteomic data is essential for understanding various biological processes and disease mechanisms.
- Metabolomic Data: Concentrating on metabolic profiles, this data type helps in understanding cellular processes and can signal specific states of health or disease.
- Ecological Data: This encompasses data related to species interactions, climate factors, and habitat specifics, contributing to biodiversity assessments and conservation efforts.
Each type plays a different role in advancing biological understanding, allowing researchers to tackle complex questions across disciplines. The interplay of these data types, especially when analyzed through AI techniques, can yield insights that are not only novel but vitally important for future developments.
Data Management Challenges
Yet, this integration isn't without its challenges. One significant issue at hand is the management of biological data itself. While we are swimming in data, the sea can be quite treacherous.
- Data Volume: As the quantity of biological data expands exponentially, the sheer size makes storage, retrieval, and processing daunting tasks. Traditional data management systems often find themselves grappling with this onslaught.
- Data Quality and Integrity: The reliability of analysis hinges on high-quality data. Missing values, errors in sequencing, and inconsistencies can impede research. Ensuring data integrity becomes an arduous task requiring constant vigilance.
- Interoperability: Biological data often comes from diverse sources and formats. Ensuring that these heterogeneous data types can work seamlessly together is an ongoing hurdle, as it hampers effective comprehensive analysis.
Faced with these challenges, researchers must adopt innovative data management solutions, ranging from advanced database systems to sophisticated AI-driven tools, to facilitate the proper handling of biological data. Without addressing these data management barriers, the potential benefits of integrating AI with biological research may remain tantalizingly out of reach.
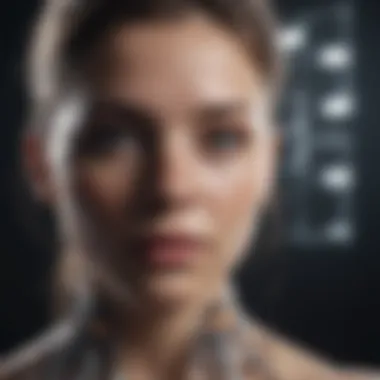
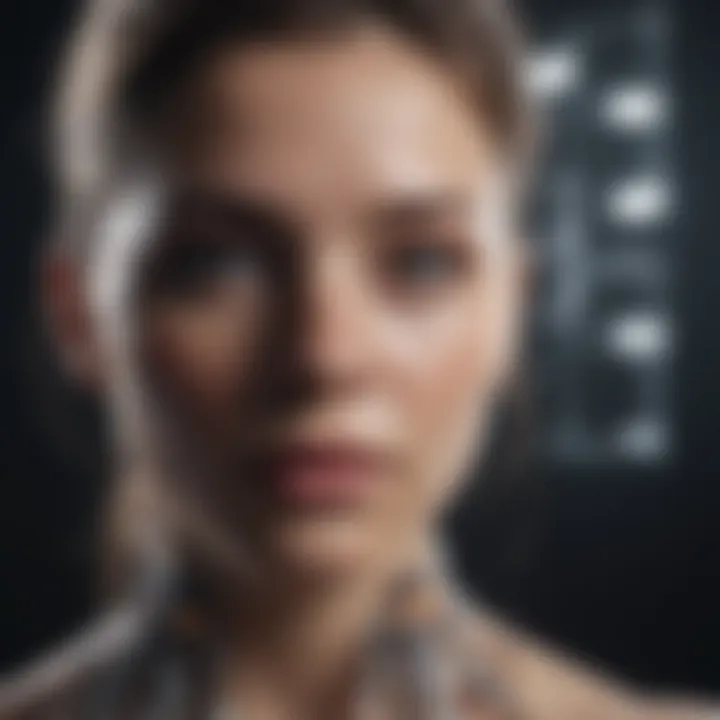
AI Applications in Genetics
Artificial intelligence is making waves in many fields, and genetics is no exception. The use of AI in genetics not only streamlines the research process but also unlocks insights that were once buried beneath layers of data. By analyzing vast quantities of genetic information, AI tools are enabling scientists to uncover relationships that might be invisible to traditional methods. This application is essential, especially as the complexity of genetic data continues to grow.
Importance of AI in Genetics:
- Increased Efficiency: AI, with its capacity to process and learn from large datasets, drastically reduces the time needed to analyze genetic sequences. Algorithms can sift through millions of data points quickly, turning what used to take months into mere weeks.
- Predictive Power: By employing machine learning techniques, AI can predict genetic predispositions to diseases. Such insights can lead to earlier interventions and more personalized healthcare strategies.
- Enhanced Accuracy: AI models can identify patterns in genetic information that may be too subtle for human researchers to catch. This capability helps in refining our understanding of gene functions and their implications in health and disease.
"AI not just aids in the quick scrutiny of data but ushers in a paradigm change in how we interpret biological information."
Genomic Sequencing
Genomic sequencing involves determining the complete DNA sequence of an organism's genome. AI significantly enhances this process through advanced algorithms that manage sequencing data; they improve accuracy and efficiency. When we sequence a genome, we generate enormous sets of data, the interpretation of which can be daunting. AI-driven algorithms can manage and analyze these datasets effectively, identifying variations and mutations that could be contributors to diseases.
- Applications in Genomic Sequencing:
- Variant Calling: AI models can precisely identify single nucleotide polymorphisms (SNPs) and structural variants from raw sequence data, which is crucial for understanding genetic disorders.
- Assembly Algorithms: Machine learning algorithms assist in piecing together fragmented genomic data, creating more accurate representations of the genome.
This significant leap in genomic technology is opening doors for personalized medicine, where treatments can be tailored more specifically to an individual's genetic makeup.
Gene Expression Analysis
Gene expression analysis is a critical field in molecular biology that focuses on the transcription of genes into RNA. AI plays an instrumental role in understanding gene expression and its variability across different conditions. By employing methods like natural language processing and machine learning, researchers can discern the mechanisms by which genes are regulated and expressed.
- Benefits of AI in Gene Expression Analysis:
- Large-scale Data Handling: AI tools are capable of processing vast amounts of gene expression data swiftly, analyzing how genes respond to environmental and biological changes.
- Expression Profiling: Using AI, scientists can compare expression profiles of various samples, which helps in identifying differential expression patterns associated with diseases.
The intersection of AI and genetics is creating a new landscape for research. As these technologies evolve, we can expect even more groundbreaking applications that continue to enrich our understanding of genetics.
AI in Drug Discovery
The fields of artificial intelligence and drug discovery have aligned in ways that were once mere dreams. The integration of AI in this area is a beacon of hope, brimming with potential to streamline the traditionally arduous and costly process of developing new medications. With the world grappling with rising health issues and the ongoing challenges posed by drug resistance, AI offers a transformative approach that raises efficiency while reducing the time required to bring new drugs to market.
AI-enhanced methodologies can significantly cut down on the chaos that typically accompanies drug development by spotlighting the most promising compounds and predicting their likelihood of success in clinical trials. This intersection also enables researchers to uncover hidden patterns within massive datasets that lay dormant, often unexamined. To put it plainly, it acts as a magnifying glass, revealing insights that would remain veiled without the impeccable diligence of computational analysis.
High-Throughput Screening
High-throughput screening stands as one of the critical techniques reshaping how drugs are discovered. This method entails the rapid testing of thousands to millions of compounds against targeted biological pathways or anomalies. Think of it like sifting through a mountain of pebbles, searching for that one sparkling gem. The brute force of AI can heighten this process, making it not just faster but also more accurate.
One benefit of using AI here is its ability to learn from previous data. Algorithms can pinpoint which compounds showed promise in earlier screenings and zero in on similar molecules in future tests. This feedback loop allows scientists to refine their searches continuously, effectively stacking the odds in favor of success. Coupled with robotics, it creates an assembly line of sorts, where compounds race through various tests at an unprecedented scale. With these advances, researchers are more likely to discover the needle in the haystack, thereby accelerating the overall drug discovery process.
"High-throughput screening, aided by AI, has the potential to transform pharma R&D into a data-driven venture that reduces lead times and enhances the quality of therapeutics."
Predictive Modeling of Drug Behavior
The landscape of drug behavior can be likened to navigating a complicated maze. Predictive modeling utilizes AI algorithms to simulate how drugs will react once they enter the human body. The intricate dance between a drug's molecular structure and its effect on various biological systems can be thoroughly analyzed, allowing researchers to anticipate efficacy, toxicity, and the drug's absorption rates.
By categorizing historical trial data and incorporating patient demographic variables, the predictive power of AI provides an edge. New models can generate insights into how specific populations might respond to drug treatments, fostering the development of personalized medicines that cater to individual needs. This adaptability adds a layer of precision that was largely absent in older methods.
The immediate benefits of these predictive models are myriad. They not only decrease the risk of late-stage clinical failures but also help prioritize which compounds should progress through the pipeline. Ultimately, the marriage of AI and predictive modeling in drug discovery is paving the way for a future filled with innovative solutions that could potentially address medical challenges with much greater accuracy and efficiency.
Bioinformatics Revolution
One of the key elements of the bioinformatics revolution is its ability to handle data at an unprecedented scale. With the advent of high-throughput technologies, such as next-generation sequencing, biologists are inundated with a deluge of data. This influx requires sophisticated data analysis techniques to extract meaningful insights. Bioinformatics provides a framework for managing, traversing, and interpreting these complex datasets.
Data Analysis Techniques
When discussing data analysis in bioinformatics, several techniques stand out:
- Sequence Alignment: This method involves comparing genetic sequences to identify similarities and differences. Algorithms like BLAST (Basic Local Alignment Search Tool) allow researchers to quickly assess sequence homologies, aiding in gene identification and evolutionary analysis.
- Gene Expression Profiling: Tools such as RNA-Seq enable scientists to quantify gene expression levels across various conditions. By examining differential expression patterns, researchers can gain insights into cellular processes and disease mechanisms.
- Structural Bioinformatics: This technique focuses on the 3D structure of biomolecules. Software such as PyMOL allows visualization of protein structures, facilitating the understanding of molecular interactions crucial for drug design.
- Machine Learning Models: Advanced machine learning approaches can be harnessed to predict biological outcomes based on historical data. This includes applications in disease prediction and treatment response assessment.
The application of these techniques can lead to several benefits. Firstly, they significantly streamline the research process, allowing scientists to focus on analysis rather than data curation. Additionally, more accurate predictions and classifications emerge from the use of AI-driven techniques, thereby enhancing the potential for significant discoveries.
Integration of Big Data in Biology
Big data integration is another cornerstone of the bioinformatics revolution. The ability to combine and analyze vast datasets from multiple sources ā such as genomics, proteomics, and metabolomics ā allows for a more holistic view of biological systems. Hereās how integration plays a crucial role:
- Comprehensive Analyses: By aggregating data from various biological disciplines, researchers can uncover relationships that would remain under the radar through isolated analyses.
- Collaboration: Integrating big data fosters a collaborative environment among researchers and institutions, as findings from one field can inform another. For example, genomics data may assist in understanding ecological impacts in environmental biology.
- Trend Identification: Patterns emerge more readily when large datasets are analyzed. AI can recognize subtle trends that might be overlooked in smaller datasets, providing critical insights for future research directions.
Moreover, significant challenges accompany big data integration, such as ensuring data consistency, managing privacy concerns, and developing interoperable platforms. Nonetheless, the potential rewards are immense.
"Bioinformatics is not just a tool; it's a compass steering biology into the era of data-driven discovery."
The infusion of AI into bioinformatics offers not only groundbreaking efficiency but also the promise of making previously untenable research questions approachable. The beginning of this revolution sees researchers harnessing data's power to decode life's complexities, shaping a future where biology and technology coalesce seamlessly.
AI in Ecosystem Management
The integration of artificial intelligence in ecosystem management marks a significant leap towards understanding and preserving the environments that we inhabit. As the global community faces pressing environmental changes, AI provides crucial tools and insights that help manage biodiversity, promote conservation efforts, and maintain ecological balance. With algorithms capable of analyzing vast data pools, AI streams new life into traditional ecosystem management approaches, enabling stakeholders to make informed and timely decisions.
Biodiversity Assessment
Biodiversity assessment, the process of evaluating the variety of life in a given ecosystem, has historically been a labor-intensive endeavor. AI excels in this arena by automating data collection and analysis. Machine learning models can sift through extensive datasets from sources like satellite imagery and field measurements. For instance, using machine vision, AI can distinguish between various species in dense forestsāa task that would take humans far longer and with less accuracy.
The benefits of incorporating AI into biodiversity assessments are manifold:
- Speed and Efficiency: Automated systems can rapidly process changes in ecosystems due to climate change or human impact.
- Predictive Analytics: Forecasting potential biodiversity loss allows conservationists to implement changes preemptively.
- Accessibility: A larger pool of data can be made available to the public, improving community engagement in local conservation efforts.
With these strengths in data handling, AI not only enhances our understanding of current biodiversity levels but also pinpoints areas that need urgent attention. AI methodologies lead to better resource allocation, ultimately creating a more sustainable environment.
Conservation Strategies
Conservation strategies are often reactive, responding to challenges as they arise. However, AI changes the game by enabling a more proactive approach to ecosystem protection. With tools such as data mining and machine learning, it allows for the identification of at-risk habitats and species before a crisis escalates. Data-driven conservation strategies provide an evidence-based framework that informs policy and on-the-ground actions.
Some key areas where AI is making inroads in conservation strategies include:
- Species Monitoring: Intelligent tracking systems utilize camera traps and drones to monitor wildlife populations continuously, allowing for real-time adjustments in conservation approaches.
- Habitat Restoration: AI algorithms can analyze soil health, weather patterns, and species interactions to develop precise intervention techniques for habitat recovery.
- Community Engagement: Platforms powered by AI can connect conservationists with local communities, providing them with essential tools and knowledge for better environmental stewardship.
Applying AI in conservation not only maximizes the impact of limited resources but also empowers everyday citizens and local organizations to play a vital role in ecosystem management. The capacity for AI to forge massive connections across disciplines and domains showcases its potential to drive meaningful environmental change.
"To nurture our natural world effectively, we must embrace AI innovations that guide us in understanding and maintaining our ecosystems."
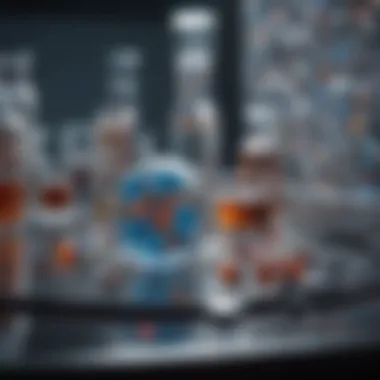
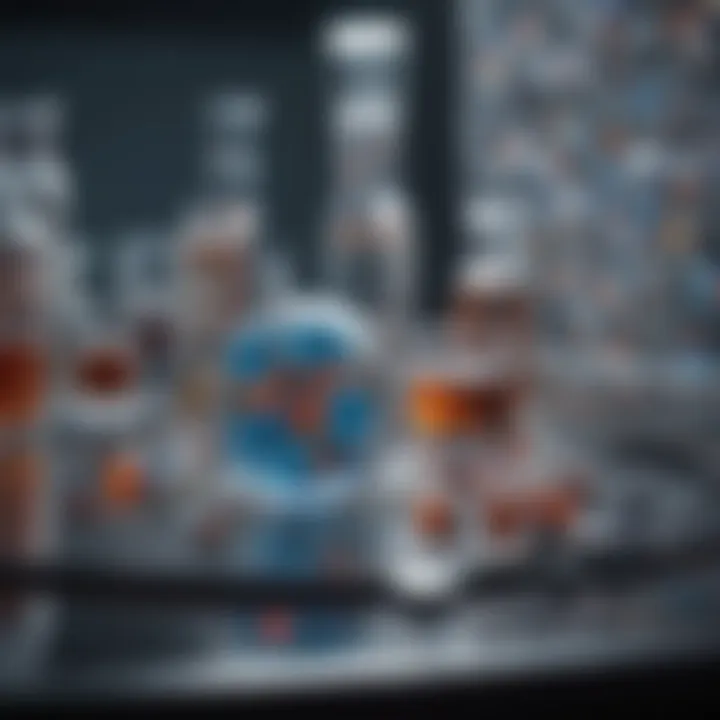
Ethical Considerations
In the rapidly evolving landscape of artificial intelligence and biology, ethical considerations form a critical backbone for discussions. In our quest to leverage AI's vast potential, we must not overlook the societal implications tied to data usage, algorithmic decision-making, and overall accountability. As these technologies advance, there are specific elements that weigh heavily on our moral compass, affecting both individuals and broader communities.
One pertinent aspect of ethical considerations is data privacy. With the enormous volume of biological data generated through research and healthcare practices, safeguarding personal information has never been more crucial. Individuals often provide intricate details about their health, familial histories, and lifestyle choices that could be misused or mishandled if not properly addressed. There needs to be robust policies to implement stringent measures to protect sensitive data, ensuring compliance with regulations while fostering trust in these systems.
Data Privacy Concerns
Data privacy concerns reflect not just a fear of breaches, but a broader anxiety about how information is collected, shared, and utilized. Many medical records, genetic information, and health-related datasets are digital now. Without proper precautions, this sensitive data may fall into the wrong hands.
"Privacy is not an option, and it shouldn't be the price we accept for just getting good service."
- Mark Zuckerberg
To address these concerns, institutions need to adopt practices that include:
- Informed Consent: Individuals should be fully aware of how their data will be used before giving consent, allowing them to make informed decisions.
- Anonymization Techniques: Data should be anonymized to protect the identities of individuals involved.
- Strict Access Controls: Implementing robust authentication processes can limit who can access or manipulate sensitive data.
By actively tackling these privacy issues, the biomedical sector can enhance the trustworthiness of AI applications.
Bias in AI Algorithms
Bias in AI algorithms is another significant ethical element demanding scrutiny. Algorithms that underpin AI systems can inadvertently perpetuate or even exacerbate existing biases found in our society. When trained on datasets that contain systematic prejudice, AI can yield warped results, affecting medical diagnoses, drug prescriptions, or resource allocations.
The implications of biased algorithms are far-reaching. They can lead to unequal treatment of diverse populations, perpetuating healthcare disparities. Itās essential for developers and researchers to understand that the underlying data source is as critical as the technology itself. This brings us to a couple of tactics to mitigate the effects of bias in algorithms:
- Diverse Training Datasets: Incorporating a variety of demographics in training datasets can improve algorithm fairness.
- Regular Audits: Continuous monitoring of the AIās outputs may unveil potential biases, allowing for timely corrections.
Addressing bias in AI isnāt merely a technical challenge. Itās a moral obligation to strive for systems that serve everyone equitably, ultimately leading to a more just society.
Challenges and Limitations
The advent of artificial intelligence in the realm of biology has sparked a plethora of innovations, yet it has also brought along a host of challenges and limitations that deserve careful consideration. The interplay between these two fields is rich with opportunity but also fraught with obstacles that can impede progress. Understanding these challenges is vital, not just for researchers but also for policymakers and educational institutions that aim to foster a conducive environment for future developments. Navigating this landscape requires insight into specific issues such as data quality and barriers between disciplines, as well as the implications they hold for the advancement of biological research.
Data Quality Issues
Data quality stands at the forefront of challenges in AI-driven biological research. The accuracy and integrity of data fundamentally influence every stage of research. If the data fed into AI systems is inadequate or flawed, the outcomes can lead to misleading interpretations. This concern can manifest in various forms:
- Incomplete Datasets: Biological data is inherently messy and often incomplete. This can arise from sampling errors, missing values, and unstandardized methodologies used in generating the data.
- Noise in Data: Biological data is frequently noisy, with many variables at play. This complexity can dilute signal strength, making it difficult for AI algorithms to learn effectively.
- Biases in Data Collection: The way data is collected may harbor biases that can skew research outcomes. For instance, if certain populations are underrepresented, AI models may fail to generalize across diverse biological contexts.
The impact of poor data quality can ripple through the research process, leading to faulty conclusions, wasted resources, and ultimately slowing down advancements in the field. Researchers and practitioners must embrace robust data management strategies to mitigate these risks.
"High-quality data is not merely a convenienceāitās the lifeblood of effective AI applications in biology."
Interdisciplinary Barriers
The fusion of AI and biology is not simply a matter of technology; it's also about people collaborating across disciplines. Interdisciplinary barriers can often stymie potential innovations. Various factors contribute to this limitation:
- Language and Terminology Differences: Each field possesses its own jargon, making communication between biologists and computer scientists challenging. This can create misunderstandings and hinder collaborative efforts.
- Varying Methodologies: The approaches and methodologies preferred in biology may clash with those employed in AI, creating friction that can stifle progress.
- Cultural Differences: The academic cultures of biology and artificial intelligence vary significantly. While biologists tend to focus on empirical data and experimentation, AI experts may prioritize theoretical constructs and algorithmic performance.
Overcoming these barriers requires intentional efforts to foster collaboration. Workshops, joint research projects, and cross-disciplinary training programs can enhance mutual understanding and pave the way for more integrated approaches. Institutions can play an instrumental role here by creating avenues for professionals from both domains to come together harmoniously.
Future Prospects
The exploration of artificial intelligence in biology holds countless possibilities for the future. As various technologies advance, the interplay between these fields is expected to grow stronger. Addressing the significance of this combination goes beyond mere academic curiosity; it has the power to reshape healthcare, agriculture, and environmental conservation.
AI's potential for driving efficient research can lead to new discoveries at an unprecedented pace. In genetics, for example, computational models can analyze vast datasets far quicker than traditional methods. Consequently, this promotes not only the speed of research but also enhances the accuracy of findings. Better algorithms could facilitate improved predictions about genetic variations and their impacts on health, thereby tailoring personalized medicine more effectively.
Moreover, the benefits extend into the realm of big data management. As our ability to collect biological data growsāthanks to technologies like next-generation sequencingāAI systems can handle, process, and derive insights from this surge of information, facilitating breakthroughs that previously seemed out of reach. But there are important considerations; ensuring that these technologies are developed responsibly will be vital.
Emerging Trends in AI and Biology
The convergence of AI and biology is already producing fascinating trends that point towards a groundbreaking future. One such trend is the development of predictive analytics, which allows researchers to forecast biological phenomena. For instance, AI can help predict how certain genetic modifications might influence traits in organisms, hence guiding selective breeding in agriculture.
Another trend gaining traction is the incorporation of machine learning models in drug discovery. By analyzing existing databases of chemical compounds, these models can identify promising candidates for further testing, significantly cutting down the time and cost associated with bringing new drugs to market. The application of computer vision in analyzing images from medical imaging adds yet another layer of innovation.
As we witness these trends evolve, itās pivotal to recognize their potential for real-world applications, especially across industries such as healthcare, agricultural science, and conservation.
Long-term Implications for Society
Looking ahead, the integration of AI and biology could usher in a new era for society. For one, enhanced healthcare solutions could emerge from the precise understanding of diseases and their genetic markers. This improved knowledge baseāenabled by AIācould lead to targeted therapies, reducing trial-and-error approaches that are often cumbersome and inefficient.
Environmental sustainability could also see a significant uplift. AI technologies can monitor ecosystems more accurately, allowing for better management of resources and informing conservation efforts. This means that societies will not only be better at healing themselves but also at protecting the planet.
However, there is a flip side to this coin. As these advancements unfold, society must remain vigilant about the ethical implications. The chance of increased inequality in access to these new technologies and data privacy issues is very real. Providing answers about who benefits from biological AI innovations will be underscored by the importance of balanced policymaking and robust ethical frameworks.
"The future is not something we enter. The future is something we create."
- Leonard I. Sweet
Case Studies
Incorporating case studies into the discussion of artificial intelligence and biology sheds light on practical applications and their influence in the field. These real-world examples serve as a bridge connecting theoretical concepts to actual practices, illustrating how AI technologies enhance biological research. The significance of case studies is profound, as they unveil both the triumphs and challenges researchers face when employing AI.
Successful Applications in Research
Case studies reveal a variety of successful applications where AI has distinctly impacted biological research. For instance, in genetics, researchers have employed deep learning algorithms to unravel intricate patterns within genomic data. An example of this is the utilization of convolutional neural networks to analyze images of cells. Such applications not only accelerate the identification of genetic variations but also refine the understanding of cellular behaviors.
Another illuminating case is the implementation of natural language processing in literature mining. This method allows scientists to sift through vast amounts of scientific publications to extract valuable insights that can inform experimental design. One study highlighted how researchers identified potential drug candidates by analyzing historical clinical trial data through AI.
These examples demonstrate that the integration of AI technologies can lead to breakthroughs that may otherwise take years to achieve manually.
Real-world Impacts of AI Integration
The integration of AI into biological research translates to significant real-world impacts that extend beyond academic curiosity. The application of AI-driven analytics has revolutionized the healthcare industry. Consider the advancements in predictive analytics that enable early diagnoses of diseases based on patient data. For instance, machine learning algorithms have successfully predicted the onset of diabetes by analyzing lifestyle factors and genetic predispositions, allowing for timely interventions.
Similarly, AI technologies have burgeoned in drug discovery. Companies like Atomwise utilize deep learning to screen vast libraries of compounds rapidly. This not only reduces the time it takes to develop new medications but also minimizes costs and increases the chances of identifying potent treatments.
āArtificial intelligence in biology is not just a tool; it's a catalyst for innovation that reshapes how we understand life itself.ā
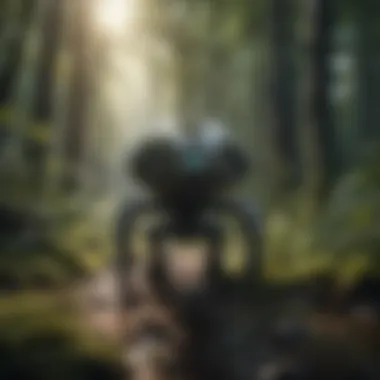
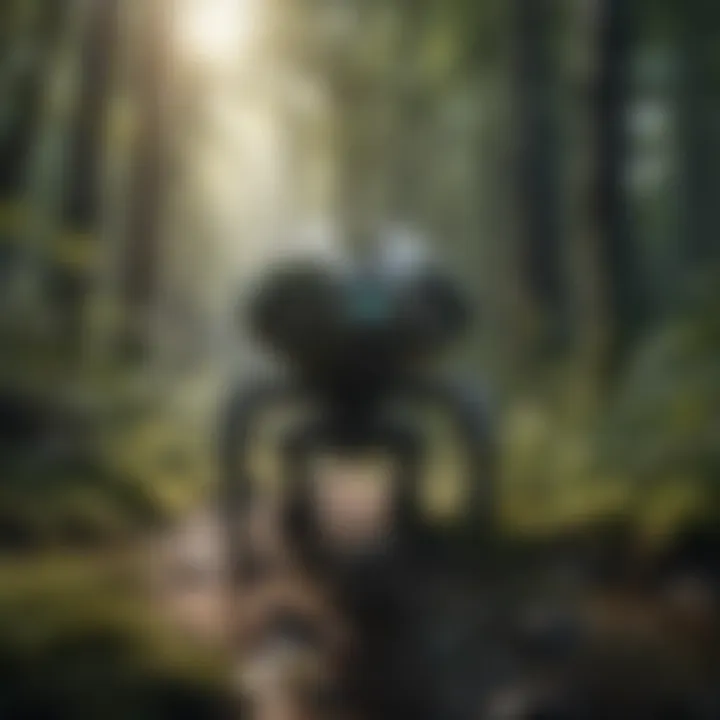
The ecological realm also benefits from AI. Tools such as satellite imagery analysis powered by AI are enhancing biodiversity assessments by tracking changes in habitats effectively. This, in turn, allows for more informed conservation strategies to protect endangered species.
In essence, the impact of integrating AI in biological research is multi-faceted, affecting everything from healthcare outcomes and drug development to ecological conservation efforts. These case studies serve not only as proof of concept but also as motivation for researchers to explore further collaborations between disciplines.
Interdisciplinary Collaborations
The confluence of artificial intelligence and biology is not merely an intersection of two distinct fields; it's a richly layered tapestry woven from various disciplines. Interdisciplinary collaborations serve as the backbone of this evolution, combining expertise from computer science, biology, statistics, and social sciences, among others. By fostering cooperation across various domains, researchers can harness a broader range of perspectives and methodologies. This not only enhances innovation but also leads to more robust solutions to complex biological challenges.
Working Between Fields
When talking about working between fields, itās essential to understand the importance of a shared language among disciplines. Those coming to the table from different backgrounds must find common ground. For instance, a biologist may be well-versed in the intricacies of genetic sequences, whereas a computer scientist may focus on algorithms and data structures. To truly move forward, both parties need to decode their jargon into accessible language.
This cross-pollination can lead to remarkable breakthroughs. A vivid example is the collaboration between molecular biologists and AI experts who developed predictive models to understand gene interactions. The model leverages machine learning algorithms to analyze large sets of genomic data, yielding insights that a single discipline alone might overlook.
Considerations about team dynamics also come into play. Academia is often siloed, with departments working in isolation. However, interdisciplinary projects can break down these barriers. By fostering teamwork through joint projects and regular workshops, professionals can create an atmosphere ripe for innovation. This collaboration doesnāt merely benefit the researchers; it opens up a wealth of opportunities for students and emerging scholars, as they learn to bridge gaps and navigate complexities.
Institutional Partnerships
Institutional partnerships where universities, research facilities, and private organizations come together are invaluable in this interdisciplinary landscape. Institutional backing can facilitate resource sharing, enabling teams to access advanced technologies, research funding, and extensive datasets that would be hard to come by independently.
For example, collaborative ventures such as the Broad Institute, which merges the power of AI with genetic research, exemplify how institutions can work together. These partnerships allow for a pooling of resources and expertise, fostering an environment where innovative ideas can flourish.
Furthermore, institutional partnerships often lead to the establishment of joint research programs aimed at specific global challenges. These might include tackling public health crises or enhancing agricultural practices through AI-driven insights into plant genetics. The synergy created can accelerate timelines, turning theoretical research into actionable solutions much quicker than could be achieved in isolation.
"Interdisciplinary efforts are not just beneficial; they are essential for groundbreaking discoveries that push the boundaries of what is possible in biological research."
Educational Implications
Curriculum Development
A robust curriculum development process must be undertaken to enhance educational opportunities in this interdisciplinary field. Hereās how that can happen:
- Integrative Approach: Curricula should seamlessly blend traditional biology with AI methods. A course might explore how algorithms can predict protein structures, merging computational techniques with biological understanding.
- Hands-On Experience: Laboratory courses should include practical sessions where students can engage with datasets using AI tools like TensorFlow or PyTorch. Real-world case studies can inspire students to apply theoretical knowledge practically.
- Cross-Disciplinary Courses: Offering courses such as "AI in Genomics" or "Computational Ecology" can draw students from both fields, fostering a collaborative learning environment that encourages diverse perspectives.
Educational programs can consider introducing a Capstone Project, where students work on real-life biological problems using AI tools. Such experiences not only deepen learning but also prepare students for the demands of the industry.
Training Programs for Researchers
As the landscape of biological research continues to evolve, so must the training provided to researchers. Here are key elements that should define these training programs:
- Foundational Skills: Researchers should gain a strong understanding of basic AI and machine learning principles, including data management and algorithm selection. This foundational knowledge will enable them to make informed decisions on the best AI techniques to apply in their research.
- Workshops and Seminars: Regularly scheduled workshops can keep researchers updated on the latest tools and methodologies in AI. These sessions should focus on practical applications, such as utilizing neural networks for gene expression studies.
- Collaborative Research Initiatives: Encourage partnerships between biologists and computer scientists through joint research projects. This collaborative approach can enhance skill sets and encourage innovative thinking.
Ultimately, providing a comprehensive education regarding AIās application in biology will empower a new generation of professionals able to navigate and excel in this evolving landscape.
"The fusion of AI and biology isn't just a trend; it's a new paradigm that shapes who we can become as researchers and innovators."
The implications for education touch every level, from undergraduate programs to advanced research training. By equipping individuals with the necessary tools and knowledge, the scientific community can look forward to robust advancements in both fields, ensuring sustained growth and innovation.
Policy Recommendations
In the rapidly evolving landscape where artificial intelligence intertwines with biology, creating effective policy recommendations becomes paramount. These policies not only guide the development of innovative applications but also establish a framework within which ethical considerations and safety concerns can be methodically addressed. Having comprehensive policies is crucial to ensuring that the integration of AI and biological research benefits society while minimizing risks.
One of the main components of these recommendations involves the establishment of regulatory frameworks. Such frameworks can provide a structured approach to oversee AI applications in biological contexts, ensuring that they are safe and effective for public use. By outlining specific guidelines for researchers and institutions, these policies aim to protect personal data, regulate experiments involving sensitive biological materials, and enforce transparency in AI algorithms.
Moreover, an emphasis on funding opportunities is essential to propel research that sits at the intersection of AI and biology. Policymakers have a role to play in directing financial resources towards initiatives that promise advancements in health, agriculture, and environmental management. This can foster innovation and inspire the next wave of interdisciplinary research.
"Policies aren't just about rules; they're about creating an environment where innovation and ethical standards flourish together."
Overall, policy recommendations guide the dialogue around the intersection of AI and biology, aiming to create a balanced system that promotes growth while keeping societal implications in check. It's about striking a professional but approachable balance, one where scientists and policymakers can work hand in hand toward shared goals.
Regulatory Frameworks for AI in Biology
The establishment of regulatory frameworks for AI in biology serves multiple functions. It ensures that the use of AI technologies in biological research and application remains both ethical and safe. With new data privacy norms and the potential for deep learning algorithms to identify patterns that human researchers may miss, regulators must stay ahead of the curve.
Some key elements include:
- Data Protection: Identifying and protecting genetically sensitive information is crucial. Regulatory frameworks should stipulate clear guidelines regarding the handling, storage, and sharing of biological data.
- Algorithm Transparency: As AI systems become more advanced, ensuring that algorithms can be interpreted and understood is vital for accountability. This includes clear reporting requirements on how decisions are made by AI systems used in biological contexts.
- Public Engagement: Gathering feedback from the public and stakeholders can enhance the frameworks. Open forums for discussion about the implications of AI in biology can help build trust and inform future regulations.
The aim is not to stifle innovation but to ensure that groundbreaking technologies are applied responsibly. By prioritizing transparency, public engagement, and data protection, these frameworks can strike a balance between innovation and responsibility. This will create a strong foundation for future developments at the intersection of AI and biology.
Funding Opportunities for Research
Funding plays a pivotal role in the advancement of research focused on the intersection of AI and biology. Properly allocated funds can turbocharge projects, allowing researchers to dive deeper into complex issues that require sophisticated technological solutions. Specifically, funding opportunities can be structured to support:
- Interdisciplinary Research Grants: Encouraging collaboration between AI experts and biologists will yield innovative solutions. By pooling resources and knowledge, these collaborative teams are better equipped to tackle multifaceted challenges.
- Public and Private Partnerships: Engaging both governmental bodies and private sector entities can leverage financial resources, knowledge, and technology. Increased interaction between public and private sectors could catalyze significant advancements through shared investments.
- Targeted Research Initiatives: Policies could define specific areas in need of exploration, such as genomics, drug discovery, or ecosystem management. Identifying high-impact areas helps to guide funding towards projects with the potential for substantial societal benefits.
Given these factors, it's essential for policymakers to create a nurturing environment that encourages funding for cutting-edge research. Through thoughtful financial investment, society can harness the full potential of AI in biological exploration, ensuring advancements that could address significant challenges in health, sustainability, and beyond.
Culmination
The conclusion of this article serves as a vital touchstone, summing up the intricate nexus of artificial intelligence and biology. Each thread explored throughout the narrative highlights the profound impact AI can wield in biological domains. From predictive modeling in drug discovery to enhanced genomic analyses, the converging paths of these fields tell a compelling story of innovation and possibility. The integration of AI into biology does not merely represent a passing trend; it indicates a fundamental shift in how biological research is conducted and applied.
Understanding this relationship sheds light on several key elements:
- Transformative Potential: AI can streamline processes that once took ages, cutting down research and development timelines significantly. Its ability to sift through vast datasets and extract meaningful trends marks a paradigm shift in scientific discovery.
- Enhanced Accuracy: With the power of machine learning, researchers can achieve outcomes that might have eluded them previously. Tools that analyze genetic patterns or predict drug interactions can lead to more effective treatments.
- Interdisciplinary Collaboration: The fusion of AI with biology calls for collaboration across fields. Understanding the language and principles of both domains allows professionals to make headway into uncharted territories of research.
"Integrating artificial intelligence in our biological explorations opens wide avenues for breakthroughs that seemed beyond reach, even a few years ago."
Moreover, as we learned in previous sections, the ethical implications of this technological merger canāt be ignored. Issues around data security, bias in algorithms, and accessibility remain critical considerations that need to be factored in as society embraces this evolution.
As we stand at this pivotal junction where biology meets artificial intelligence, it is clear that the implications of this integration are vast and multifaceted. The fields being explored and the innovations being fostered hold the promise of unlocking more profound insights into lifeās molecular mechanisms, ultimately paving the way for advancements that will benefit humanity as a whole.
Recap of Key Insights
Reflecting on the insights presented, itās essential to underscore several crucial points:
- AI's Application in Diverse Biological Fields: From genetic sequencing to ecosystem management, AI proves its worth across various biological concerns.
- Importance of Ethical Standards: Navigating the ethical landscape is paramount. The repercussions of AI's influence must be critically assessed to ensure unbiased applications.
- Collaboration and Interdisciplinary Approaches: The merging of expertise between biologists, data scientists, and ethicists is essential for harnessing the full potential of AI in biology.
This article effectively underscores how artificial intelligence is not merely a tool but a transformative partner, helping to confront some of the most challenging scientific questions today.
Looking Forward
The road ahead promises a future rich with potential as we explore the intersection of AI and biology further. Several emerging trends and technologies could shape this journey:
- Continued Advances in Machine Learning: As algorithms grow more sophisticated, their application in interpreting biological data will become deeper and broader. Expect to see more tailored solutions to specific biological problems.
- Greater Collaboration Between Fields: The ongoing fusion of diverse expertise will likely yield innovative solutions, enhancing research methodologies and findings.
- Focus on Sustainability: AI's role in managing biodiversity and ecological balance will increasingly come to the forefront. With pressing environmental issues at hand, these contributions are more critical than ever.
As we look to the future, a clear picture emerges: the partnership between AI and biology isnāt just beneficial; it's essential for tackling the increasingly complex challenges of our time. Through thoughtful and informed application of these technologies, there is hope for significant advancements in human health, ecological preservation, and the overall understanding of life itself.