Understanding Cell Line Mutation Databases: Analysis
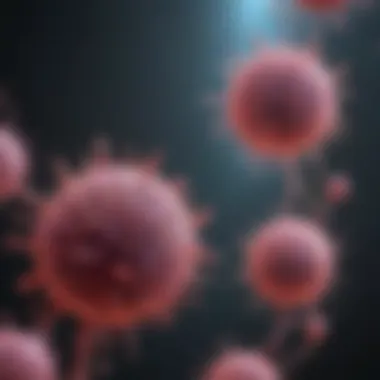
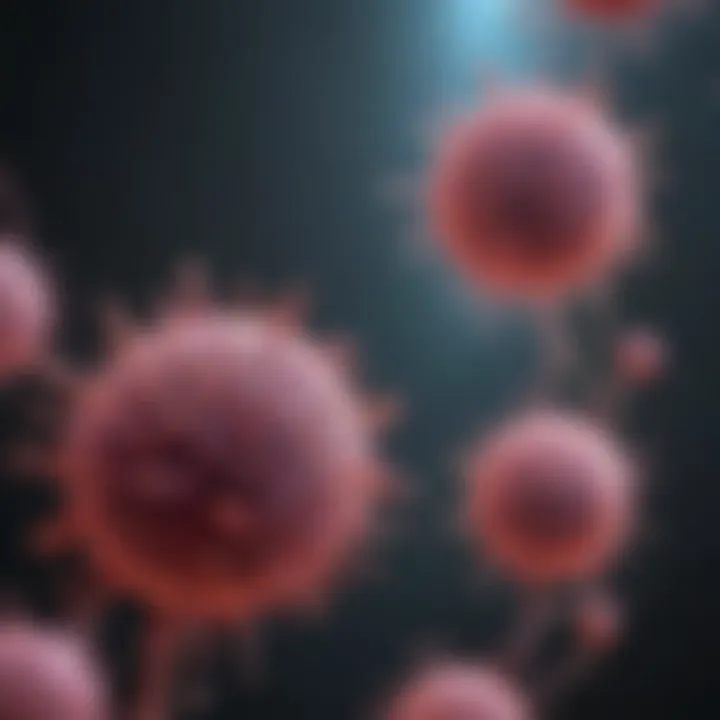
Intro
Cell line mutation databases play a pivotal role in the landscape of modern scientific research, particularly in oncology and personalized medicine. These resources consolidate genetic data from various cell lines, facilitating a deeper understanding of how mutations influence health outcomes and treatment response. With advancements in genomic technologies, the scope of these databases has expanded significantly. Researchers now have access to a wealth of information that can inform both theoretical studies and practical applications in clinical settings.
The relevance of this topic cannot be overstated. As cancers become increasingly complex, the need to personalize treatment strategies based on an individual's unique genetic profile grows. Cell line mutation databases provide a critical foundation for such personalized approaches. They serve not only as repositories of information but also as essential tools for hypothesis generation, drug discovery, and validation of research findings.
Within this article, we will explore key aspects of cell line mutation databases, examining their methodologies, the types of mutations recorded, and the implications of this data in cancer research. Furthermore, we will delve into challenges and ethical considerations associated with these databases, ensuring a well-rounded perspective for readers from various backgrounds in the scientific community.
Intro to Cell Line Mutation Databases
Cell line mutation databases serve a crucial role in the realm of scientific research, particularly in the fields of oncology and genetics. These databases aggregate vast amounts of genetic information from various cell lines, enabling researchers to analyze the complexities of genetic variations across diverse biological contexts. The importance of understanding cell line mutation databases lies not only in the insights they offer regarding cancer biology but also in their applicability to personalized medicine, drug development, and understanding disease mechanisms.
The analysis of cell line mutations allows for a greater understanding of how genetic alterations contribute to cancer progression. By studying these databases, researchers can identify specific mutations that may correlate with treatment responses or resistance patterns. Furthermore, the integration of these databases with emerging technologies, such as artificial intelligence, is fostering innovations that could enhance research capabilities and therapeutic strategies.
Definition and Purpose
Cell line mutation databases are repositories designed to store and categorize mutation information derived from various cultured cell lines. A cell line is a population of cells that have been adapted to grow continuously in vitro, often derived from tumors. The primary purpose of these databases is to provide an organized and accessible platform for researchers to access mutation data, facilitating studies that can lead to significant advancements in cancer research and treatment protocols.
These databases typically contain essential information such as the type of mutation, genetic context, and associated phenotypic traits. By systematically cataloging this data, researchers can identify patterns that dictate various cancer biology aspects, aiding the development of targeted therapies.
Historical Background
The concept of cell line mutation databases began to take shape with the advent of molecular biology techniques in the latter part of the 20th century. Early research focused primarily on individual mutations within specific genes. As molecular techniques advanced, the ability to analyze multiple mutations across different genes and pathways emerged.
The 1990s saw significant developments in genomic technologies, which allowed for high-throughput sequencing and more comprehensive genetic analyses. This period provided a foundation for the establishment of dedicated databases such as the Cancer Cell Line Encyclopedia (CCLE) and the Genomic Data Commons (GDC). These resources have been instrumental in assembling mutation data linked to various cancer types, thus paving the way for modern precision medicine research. As research methodologies improved, so did the scope and scale of information contained within these databases, giving rise to today’s complex and multifaceted resources.
Key Components of Cell Line Mutation Databases
Understanding the key components of cell line mutation databases is crucial for grasping their role and utility in research. These databases serve as repositories of genomic information, necessary for studies in cancer and personalized medicine. Knowledge of how data is formatted and sourced is essential.
Data Types and Formats
The types and formats of data stored in cell line mutation databases vary significantly, affecting how researchers utilize this information. The primary data types are genomic sequences, variant call formats, and annotations.
- Genomic Sequences: This includes DNA and RNA sequences collected from cell lines. These sequences capture genetic variations found within specific tumor samples or healthy controls.
- Variant Call Formats (VCF): VCF files are critical in documenting the mutations observed in the genomic sequences. They provide details about each variant, including its type, position, and impact on gene function.
- Annotations: Metadata that explains the biological significance of the variants, such as associated diseases or known interactions with treatments, is collected alongside genomic data. These annotations enhance the research potential of cell line mutation databases by contextualizing the data.
Using established formats ensures consistency. Commonly utilized resources for genomic data include to tools and software compatible with these formats, allowing researchers to perform analyses effectively.
Data Sources
Cell line mutation databases draw from a variety of data sources, maximizing their comprehensiveness. Some principal sources are:
- Biobanks: These facilities collect and store biological samples. They provide vital genomic information for cell lines derived from patient tissues.
- Public Repositories: Organizations such as The Cancer Genome Atlas (TCGA) make large datasets available to the research community. These repositories are invaluable for cross-comparison between independent studies.
- Peer-Reviewed Publications: Research studies that report novel findings often share their datasets openly. This helps create a larger body of knowledge on mutations within specific cancers.
- Collaborative Networks: Many databases are created through collaborations between universities, research institutes, and pharmaceutical companies. These partnerships help gather diverse and comprehensive datasets.
"Access to a diverse range of data sources enhances the reliability and applicability of cell line mutation databases in research."
By synthesizing information from these sources, cell line mutation databases improve the robustness of conclusions drawn from analyzed data. The integrity of the data stored is a vital part of ensuring that findings can be replicated and verified by other researchers.
Methodologies for Data Collection
Data collection is a cornerstone of understanding cell line mutation databases. The methods employed dictate the quality, reliability, and scope of the information generated. As scientists increasingly rely on these databases to inform research, the methodologies used in data collection must be rigorous and highly systematic. The underlying aim is to ensure that the data remains accurate and pertinent to ongoing studies in genetic variations and their implications for human health.
Genomic Sequencing Techniques
Genomic sequencing is a central methodology employed in the collection of mutation data. This technique provides a comprehensive view of the genetic makeup of cell lines. It includes several approaches, such as whole-genome sequencing, targeted sequencing, and exome sequencing, each contributing uniquely to the understanding of mutations.
- Whole-genome sequencing offers a complete snapshot of the organism's DNA, identifying all mutations present. This depth of data enables researchers to discern patterns that may be indicative of disease progression.
- Targeted sequencing focuses on specific regions of interest, often selected based on clinical relevance. This method is economical and efficient, allowing for rapid identification of known mutation hotspots associated with various cancers.
- Exome sequencing examines only the exons, or coding regions, of the genome. As these regions comprise a small percentage of the genome but contain the majority of mutations relevant to disease, this method is frequently utilized in mutation databases.
Each sequencing technique has its benefits and limitations. One key consideration is the coverage depth, which may impact the detection of low-frequency mutations. Therefore, adopting the most suitable genomic sequencing technique is crucial for obtaining robust data that can enhance the understanding of cellular mutations.
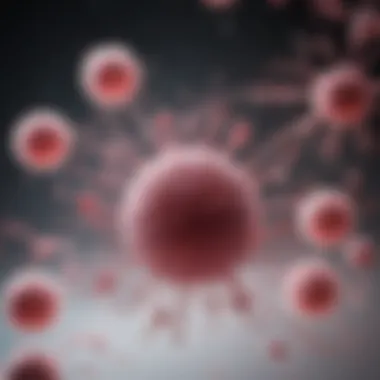
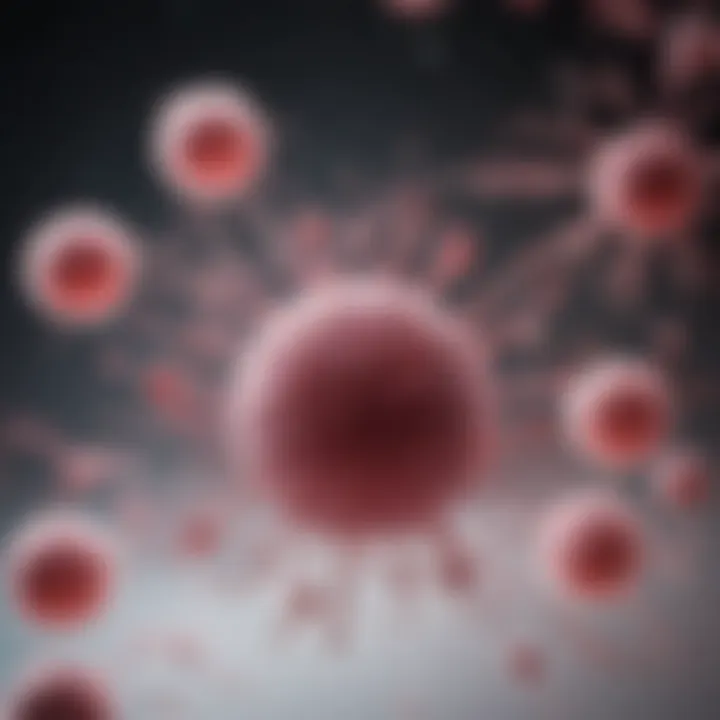
Data Integration and Annotation
Once data has been collected via genomic sequencing, the next step involves integration and annotation. This process is vital for creating a usable and informative database. Integration refers to the combination of data from various sources, while annotation provides context and meaning to the raw data.
- Data integration allows for the cross-referencing of mutation data with clinical outcomes, expression profiles, and treatment responses. By correlating information, researchers can draw more substantial conclusions and identify potential biomarkers for therapies.
- Annotation is equally important as it categorizes mutations based on their significance. This includes noting whether a mutation is known, novel, or benign, and linking it to relevant biological pathways or functions.
Accurate integration and comprehensive annotation not only enhance the quality of the cell line mutation databases but also facilitate their usage in research and clinical settings. Researchers can leverage this integrated and annotated data to formulate hypotheses, identify trends in mutation prevalence, and inform targeted therapeutic strategies.
"The quality of the data existing in mutation databases is directly related to the methodologies employed in data collection. Thus, innovative sequencing and robust integration processes are imperative for advancing cancer research."
The effectiveness of methodologies for data collection ultimately influences the capacity of cell line mutation databases to contribute meaningfully to cancer research and personalized medicine. Through meticulous genomic sequencing and thoughtful data integration and annotation, these databases can serve as invaluable resources for understanding genetic variations and their implications.
Types of Mutations Documented
Understanding the types of mutations documented in cell line mutation databases is essential for grasping their applications in medical research. Mutations are essentially changes in the genetic code that can affect cellular behavior and function. Focusing on somatic and germline mutations provides critical insight into how these genetic variations contribute to diseases like cancer.
Somatic Mutations
Somatic mutations are alterations in the DNA that occur after conception. These mutations are not inherited, which means they arise in non-germline tissues and cannot be passed to offspring. They can be caused by various factors, including environmental influences, lifestyle choices, and errors during cell division.
The importance of somatic mutations in cancer research cannot be overstated. Many cancers result from a series of somatic mutations that confer growth advantages to cells, enabling them to proliferate uncontrollably. Somatic mutations can affect several key genes, including oncogenes and tumor suppressor genes.
The characterization of somatic mutations allows scientists and oncologists to:
- Identify targets for treatment: Many targeted therapies, such as those for epidermal growth factor receptor (EGFR) mutations in non-small cell lung cancer, rely on knowledge of specific somatic mutations.
- Understand tumor evolution: Tracking somatic changes can reveal how tumors adapt to treatments over time.
- Predict patient outcomes: The presence of certain somatic mutations can give insights into prognosis and help in deciding on treatment strategies.
Germline Mutations
Germline mutations are genetic alterations that are present in the reproductive cells and can be passed from one generation to the next. These mutations are significant as they can predispose individuals to various diseases and conditions, including certain types of cancer. It is crucial to differentiate them from somatic mutations, as they represent inherited genetic risks rather than acquired changes.
Germline mutations may affect tumor suppressor genes like BRCA1 and BRCA2, which are linked to hereditary breast and ovarian cancers. Understanding these mutations can lead to important implications in cancer prevention and management strategies, particularly in personalized medicine.
Studying germline mutations enables researchers to:
- Identify hereditary cancer syndromes: Knowledge of germline mutations facilitates screening and genetic testing for at-risk individuals.
- Inform family planning: Genetic counseling can help affected families understand their risks of passing mutations to their children.
- Support research on population genetics: Comprehensive databases of germline mutations contribute to epidemiological studies, potentially guiding public health strategies.
Applications in Cancer Research
The application of cell line mutation databases is crucial to understanding cancer biology and improving therapeutic strategies. These databases provide a wealth of genomic and mutational information, which aids in elucidating the complex landscape of cancer. By analyzing these mutations, researchers can identify potential targets for intervention, ultimately leading to better patient outcomes.
Targeted Therapy Development
Targeted therapy is a treatment approach that utilizes medications designed to target specific molecular pathways involved in cancer progression. Information from cell line mutation databases enables researchers to identify mutations associated with specific cancer types.
This means:
- Researchers can examine how particular mutations influence tumor behavior.
- They can also predict which therapies will be effective based on the tumor’s genetic profile.
For instance, the identification of mutations in the EGFR gene in lung cancer has led to the development of inhibitors like Erlotinib and Gefitinib. These drugs target tumors with these specific mutations, showing higher efficacy compared to traditional chemotherapy.
Moreover, the use of data from cell line mutation databases can enhance the process of drug discovery. By integrating mutation data with clinical outcomes, researchers can evaluate how well a new drug performs in cell lines that harbor relevant mutations. This accelerates the pace of developing targeted therapies and potentially reduces costs.
Biomarker Identification
Biomarkers are biological molecules that indicate the presence or progression of a disease. In the context of cancer, they are critical for early detection, diagnosis, and monitoring of treatment response. The relevance of cell line mutation databases in biomarker identification cannot be overstated.
Through comprehensive mutation data, researchers can discover and validate new biomarkers that reflect the genetic makeup of tumors. For example:
- Mutations in the KRAS gene have been established as a biomarker for colorectal cancer.
- Data from cell line studies can support the examination of variants in this gene, correlating specific mutations with clinical outcomes.
The process is facilitated by the ability to compare mutations across various cancer cell lines, leading to the identification of consistent patterns that may serve as reliable biomarkers. This process not only aids in prognosis but also personalizes treatment plans based on individual biomarker profiles.
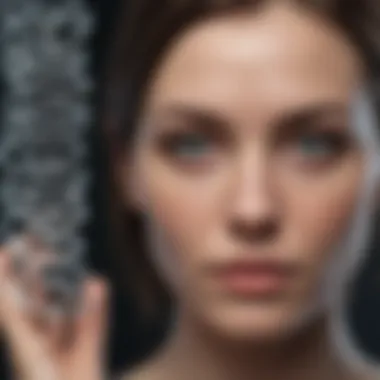
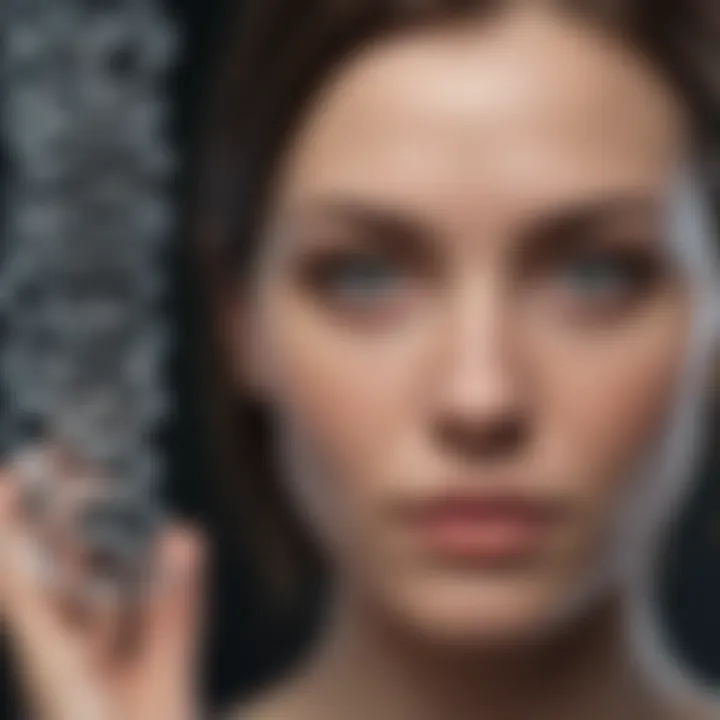
The detailed analysis of mutations through cell line databases plays a pivotal role in advancing both targeted therapies and biomarker identification, enhancing cancer research and treatment options.
In summary, the applications of cell line mutation databases in cancer research extend from targeted therapy development to the discovery of impactful biomarkers. The insights gained from these databases pave the way for more personalized and effective treatment approaches, which are essential in the evolving landscape of cancer therapy.
Role in Personalized Medicine
The role of cell line mutation databases in personalized medicine is crucial. Personalized medicine aims to customize healthcare, with medical decisions tailored to the individual patient. Cell line mutation databases contribute significantly to this approach by providing insight into genetic variations that influence treatment responses.
Tailored Treatment Strategies
In personalized medicine, understanding the genetic makeup of patients can guide treatment strategies. Cell line mutation databases provide data about how specific mutations affect the efficacy of therapies. For example, knowledge of a tumor's mutation profile can help oncologists choose targeted therapies that are more likely to succeed. This means that a patient with a specific mutation may receive a unique drug regimen designed for their genetic profile.
- Identification of Potential Therapies: These databases help in identifying existing drugs that target specific mutations. Researchers can quickly access which mutations correspond to sensitive or resistant responses to treatment.
- Development of New Treatments: Insights drawn from these databases can also inform the creation of new drugs. By understanding how mutations contribute to disease, pharmaceutical companies can develop innovative therapies that directly address these genetic alterations.
- Monitoring Treatment Response: The mutation data enables healthcare providers to monitor how well a patient responds to a treatment over time, facilitating adjustments as necessary. The ongoing assessment of mutation status can thus inform treatment decisions.
Patient Stratification
Patient stratification is another essential component of personalized medicine. This process involves dividing patients into subgroups based on their genetic attributes. Using cell line mutation databases, researchers can categorize patients more accurately according to their mutation profiles.
- Improved Clinical Trials: Patient stratification allows for more focused clinical trials. By selecting participants based on specific mutations, researchers can better assess the effectiveness of targeted treatments in these distinct groups.
- Enhancing Treatment Efficacy: By understanding which patients are most likely to benefit from a new treatment due to their genetic variations, healthcare providers can ensure a more efficient use of resources and improve patient outcomes.
- Reducing Adverse Effects: It is vital to recognize which patients are likely to experience adverse effects based on their genetic background. Cell line mutation databases can provide insights into risks associated with certain therapies.
"Personalized medicine is not just about individualizing treatments; it's about understanding the genetic landscape to improve outcomes and reduce risks."
The integration of cell line mutation databases into personalized medicine affirms the necessity for a deep understanding of genetic variability. It helps maximize the potential for success in treatment regimens while minimizing risks associated with ineffective therapies.
Challenges and Limitations
Understanding the challenges and limitations of cell line mutation databases is crucial for researchers in the fields of genetics and cancer biology. These databases offer extensive information that can propel research forward. However, they also present significant obstacles that need to be addressed to ensure that the data generated is not only reliable but also ethically sound. This section will dissect these challenges, with a focus on data quality and ethical considerations.
Data Quality and Reliability
The integrity of data in cell line mutation databases is paramount. High-quality data ensures that research findings are valid and reproducible. However, several factors can undermine data quality. First, mutations can vary based on the cell line used, leading to inconsistencies in results. Additionally, not all mutations that might be relevant for certain research objectives are documented.
- Sampling Bias: Often, certain cell lines are favored, which can introduce bias into the data. This bias can skew research outcomes and limit the understanding of mutation impacts across diverse population groups.
- Updates and Maintenance: Cell line databases need regular updates to reflect new research findings. Lack of timely updates can lead to misconceptions and outdated information being cited in new studies.
Ensuring data reliability involves rigorous validation processes. This includes cross-referencing mutations with clinical data and genomic studies. Tools and algorithms are also being developed to assess the quality of data within these databases to enhance confidence in their use.
"As we advance our understanding, the demand for high-quality data is more pressing than ever. Researchers must advocate for improved quality standards."
Ethical Considerations
The ethical landscape surrounding cell line mutation databases is complex. One significant concern is informed consent, especially when it comes to human-derived cell lines. Researchers must ensure that the individuals from whom the cells are derived have provided informed consent for their use in research.
- Privacy Issues: Protecting the identity and genetic data of individuals is critical. Mismanagement can lead to breaches of confidentiality, raising ethical flags in research.
- Ownership of Data: Questions about ownership and the implications of sharing this data persist. Researchers have to navigate the legal and ethical nuances of using genetic information obtained through cell lines.
To address these ethical concerns, guidelines and best practices are being established. Institutions are encouraged to create ethical review boards to oversee the use of human-derived cell lines in research. This kind of oversight can help mitigate potential ethical pitfalls.
Maintaining a balance between scientific advancement and ethical responsibility is essential for the continued use and development of cell line mutation databases. Researchers must stay vigilant and proactive in identifying and addressing these challenges to uphold the integrity of their work.
Recent Advances in Cell Line Mutation Research
Recent progress within the realm of cell line mutation research has significantly propelled the scientific dialogue around genetic variations and their implications for health. These advances are crucial for understanding how mutation databases can shape future studies and ultimately lead to better medical outcomes. Several specific elements underscore the importance of recent developments in this area.
First, innovative methodologies have emerged, allowing researchers to analyze mutations more comprehensively. As databases continue to grow, they incorporate new mutation types and reflect more diverse populations. This expansion allows for more nuanced research conclusions and prepares the ground for personalized medicine. Furthermore, the integration of diverse datasets fosters collaboration among institutions worldwide, ensuring a more inclusive exploration of genetic factors in diseases.
"The advancements in cell line mutation research are not just technical improvements; they shape the very foundation of how we understand genetic variability and its impact on human health."
Innovative Comparisons with Clinical Data
One of the standout trends in recent cell line mutation research is the emphasis on comparing cell line data with clinical findings. Such comparisons are essential because they bridge the gap between laboratory studies and real-world applications. Researchers have started employing advanced statistical models and bioinformatics tools to analyze how mutations found in cell lines correspond to those seen in clinical samples.
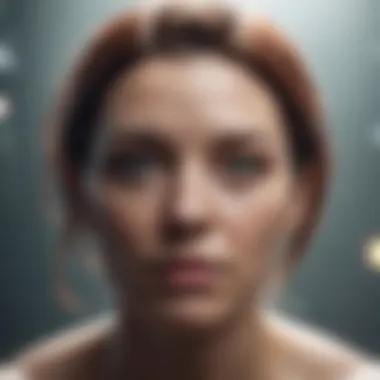
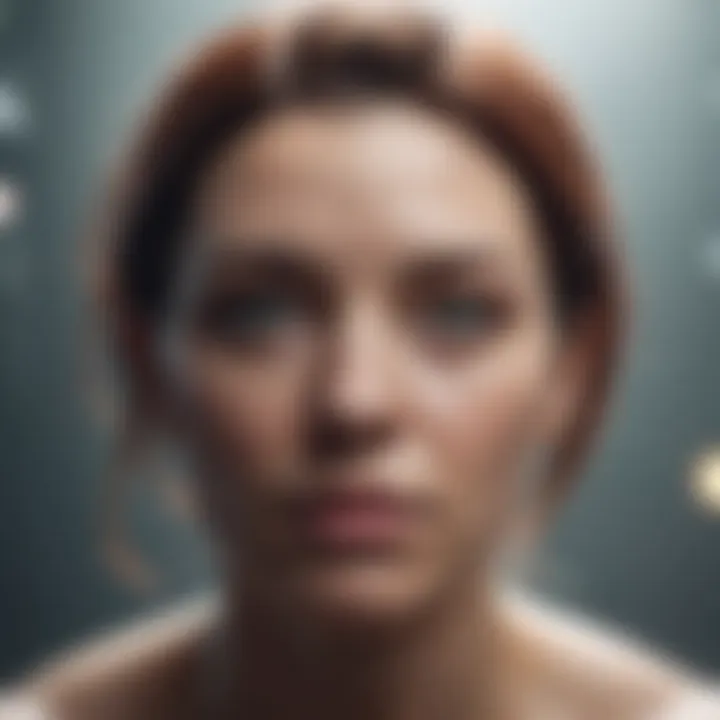
- Enhanced Validity: This approach ensures that findings from cell lines translate more directly into insights applicable to patients. By correlating cell line mutations with clinical outcomes, researchers can validate their hypotheses with far greater confidence.
- Targeted Analysis: Researchers can examine specific mutations that appear more frequently in clinical cases of certain cancers. This could lead to refined focus areas in therapeutic research, enhancing targeted therapies.
- Patient Cohorts: A comparative framework can also inform patient stratification. Understanding how different mutation profiles affect treatment responses allows medical professionals to tailor interventions.
Technological Innovations
Technological advancements play a pivotal role in the evolution of cell line mutation databases. Innovative tools and techniques have emerged that augment the data collection and analysis processes.
- Next-Generation Sequencing (NGS): This technology has transformed genomic research by allowing rapid sequencing of entire genomes. As a result, researchers can now identify mutations at an unprecedented scale and speed.
- CRISPR-Cas9: Applications of gene-editing tools, such as CRISPR-Cas9, enable scientists to create cell lines with specific mutations. This not only aids in understanding mutation impacts but also establishes ideal models for further exploration.
- Machine Learning: Algorithms and machine learning models analyze vast datasets, uncovering patterns that may not be visible to human researchers. These innovations can offer predictive insights, informing new hypotheses and optimizing research strategies.
Global Collaboration and Data Sharing
In the realm of cell line mutation databases, global collaboration and data sharing emerge as pivotal components that significantly enhance both the scope and impact of research. The fundamental tenet of this collaboration rests on the understanding that genetic research transcends geographical borders. By pooling resources, knowledge, and data, researchers can foster innovations that are otherwise unattainable in isolated environments.
Furthermore, collaboration encourages a diverse array of perspectives and methodologies. By involving international experts in the field, researchers can benefit from varied approaches to data curation, interpretation, and practical implementation. This amalgamation of knowledge fundamentally enriches the database, providing a more comprehensive resource for all users.
Benefits of Global Collaboration:
- Increased Access to Data: Collaborative platforms typically house a larger volume of data, facilitating more extensive research opportunities.
- Shared Best Practices: Research groups can exchange methodologies and technological advancements.
- Enhanced Validation: Multi-institutional efforts yield better validation of findings, reinforcing the credibility of results.
- Addressing Public Health Issues: With shared objectives, researchers can tackle global health challenges more effectively, as seen during recent pandemic responses.
Additionally, the ongoing advancements in data-sharing technologies enable seamless access to mutation databases across borders. This ensures that researchers, regardless of their location, can contribute to and benefit from shared knowledge.
International Databases and Platforms
International databases such as the Cancer Cell Line Encyclopedia (CCLE) and the Genomic Data Commons (GDC) play an instrumental role in promoting global data sharing. These platforms aggregate extensive datasets from numerous contributors worldwide. They offer a centralized repository where researchers can access mutation data across various cancer cell lines.
Data within these platforms often include:
- Genomic sequences
- Mutation annotations
- Phenotypic information
Such databases not only streamline the research process but also serve as validate resources for verifying findings from individual studies. Collaborating with these platforms enhances transparency, which is crucial for scientific integrity.
"The sharing of data is not just a matter of convenience; it is essential for pushing the boundaries of scientific understanding."
Impact on Research Collaboration
The impact of global collaboration on research efforts cannot be overstated. When multiple institutions work together, they can accelerate the pace of discovery. Collaborative research projects often yield more significant findings due to the combined expertise and resources of different teams. This synergy is vital in a field where technological advancements occur rapidly.
Factors Influencing Research Collaboration:
- Standardization of Data: A unified approach to data formatting and quality assurance significantly eases collaborative efforts.
- Funding Opportunities: Joint projects often have better access to funding, as grants are frequently awarded for collaborative initiatives.
- Increased Visibility: Findings from collaborative projects tend to gain more attention within the scientific community, leading to greater dissemination and discussion.
In summary, global collaboration and data sharing are not merely beneficial but essential for advancing our understanding of cell line mutations. They represent a collective effort to push research boundaries and tackle pressing health challenges through integrative approaches. By engaging in this collaborative spirit, the incremental knowledge gained from mutation databases can be maximized for the greater good.
Future Directions for Cell Line Mutation Databases
The future of cell line mutation databases holds great promise for transforming how researchers approach genetic studies. Integrating advanced technologies and methodologies can enhance the quality and accessibility of mutation data. This section examines two essential areas that are poised to shape the evolution of these databases: the integration with artificial intelligence and the exploration of emerging research areas.
Integration with Artificial Intelligence
Artificial intelligence (AI) stands to revolutionize cell line mutation databases by automating data analysis and improving predictive modeling. The sheer volume of data generated from genomic studies necessitates sophisticated techniques for analysis. AI can assist in identifying relevant patterns and correlations in mutation data that may not be apparent through traditional analytical methods.
Key benefits of integrating AI include:
- Enhanced Data Analysis: Machine learning algorithms can sift through massive datasets quickly and efficiently. This can lead to faster identification of significant mutations associated with various diseases, particularly cancers.
- Predictive Capabilities: AI can help predict how certain mutations may influence drug response. By training algorithms on historical data, researchers can develop models that estimate treatment outcomes based on specific cellular mutations.
- Improved Data Annotation: AI tools can automatically sort and classify data, making it easier for researchers to find specific mutations. This reduction in manual annotation time can lead to a more streamlined research process.
Despite its potential, challenges remain, such as the need for high-quality training datasets and the transparency of AI algorithms used in research. Collaboration between geneticists and data scientists will play a crucial role in realizing the full potential of AI in cell line mutation databases.
Emerging Areas of Research
As genomic research progresses, several emerging areas will likely impact cell line mutation databases significantly. These areas include:
- Single-Cell Genomics: Understanding mutations at the single-cell level allows for greater insights into heterogeneity within tumor populations. Emerging techniques in single-cell sequencing can help identify rare mutations that may drive cancer progression.
- CRISPR Technology: The use of CRISPR for genome editing provides opportunities to create more accurate cell line models. Researchers can intentionally introduce mutations and study their effects in controlled environments, leading to a deeper understanding of functional biology.
- Translational Research: Bridging the gap between laboratory findings and clinical application is essential. Research focusing on how data from mutation databases can be translated into effective therapies will enhance the practical utility of these databases.
- Database Interoperability: Connecting existing mutation databases could provide a broader overview of mutation landscapes across different conditions. This type of collaboration can facilitate more comprehensive analyses and enhance researchers' access to crucial data.
The continued evolution of cell line mutation databases will depend significantly on these emerging research themes. Addressing both technological advancements and scientific inquiries will ensure these resources remain relevant and driven by the needs of the scientific community.
"The synthesis of artificial intelligence with cutting-edge genomic research signifies a transformative period for cell line mutation databases, making them indispensable in the pursuit of personalized medicine."
By recognizing the potential of AI and new areas of research, stakeholders can optimize cell line mutation databases for future inquiries. A closer alignment between technology and biology will undoubtedly enhance our understanding of genetic variations and their implications for health outcomes.