Data and Measurement: Frameworks and Insights in Science
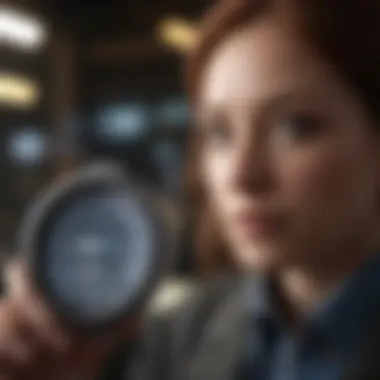
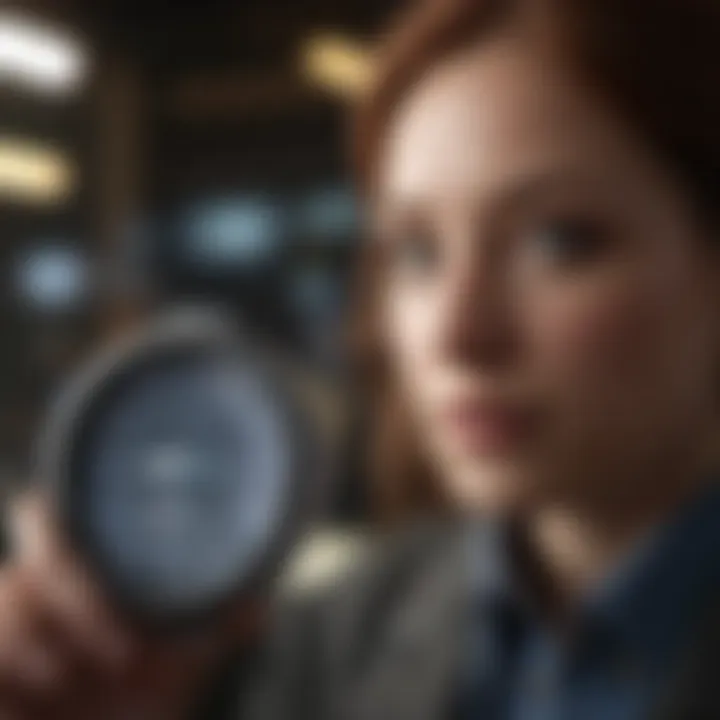
Intro
Data and measurement form the backbone of scientific inquiry, facilitating a deeper understanding of complex phenomena. In various fields, from biology to physics, the accurate collection and interpretation of data can mean the difference between valid conclusions and misguided theories. This exploration will highlight how rigorous methodologies and ethical considerations in measurement can foster scientific integrity and innovation.
Researchers often grapple with the challenge of diverse datasets, each requiring tailored strategies for analysis. The interplay between these data points can determine the success of any scientific endeavor, revealing patterns that advance knowledge and inform decision-making.
Furthermore, the implications of measurement extend beyond academia. Industries rely on data-driven insights to propel advancements in technology, healthcare, and environmental science. Therefore, understanding the frameworks and rigorous practices underpinning data collection is paramount for students, researchers, educators, and professionals.
In this context, we will delve into essential themes surrounding data and measurement.
Research Highlights
Overview of Key Findings
Through the examination of data collection methodologies and measurement techniques, several key findings emerge:
- Importance of Methodology: Different scientific disciplines employ unique data collection methods tailored to specific needs, emphasizing that no one-size-fits-all approach exists.
- Statistical Calibration: Accurate measurement necessitates careful statistical calibration to maintain reliability and validity.
- Ethical Dimensions: The ethics surrounding data collection and usage are increasingly relevant, where transparency and accountability are critical to maintaining public trust.
Significance of the Research
The significance of this research cannot be overstated. In an age where information is abundant, the ability to discern reliable data from the noise is vital. Understanding the nuances of data measurement supports the advancement of scientific knowledge across various domains, including biology, chemistry, physics, and earth sciences. Moreover, this knowledge fosters informed decision-making, shapes policies, and paves the way for technological innovations.
Original Research Articles
Summary of the Article
This article seeks to illuminate the framework of data and measurement, focusing on methodologies used to gather and analyze scientific data. It highlights the pressures many researchers face to produce significant results without sufficient understanding of appropriate measurement practices. The discussion integrates case studies and recent advancements in measurement techniques, providing a comprehensive look into the landscape.
Author Contributions
The contributions of the authors enrich the narrative by incorporating personal insights and empirical research. Each author brings specific expertise, helping to create a balanced perspective on how data and measurement shape scientific inquiry and its applications.
"Understanding measurement in science isn't just a technical skill; it's the foundation of credible and reproducible research."
Prolusion to Data and Measurement
Understanding data and measurement is crucial for anyone engaged in scientific research. This article addresses fundamental aspects of these concepts, providing insights that are essential for effective inquiry across various disciplines. Data serves as the backbone of scientific exploration, while measurement assures that this data is accurate and robust.
Data is not merely a collection of raw numbers or facts; it holds the potential to reveal patterns, trends, and relationships that are significant in decision-making processes. Measurement, on the other hand, is the method by which we attain quantifiable insights from this data. Together, they form an interdependent framework that underpins the validity of scientific findings.
By exploring the definitions and scopes of data and measurement, we can appreciate their roles within both traditional and modern scientific paradigms. Each component contributes uniquely to the overall integrity of research studies—be it in hypothesis testing, evidence collection, or broader scientific discourse. As we navigate this discussion, it becomes clear that the precision of measurement and the quality of data are paramount in achieving meaningful insights.
Importance of Data in Scientific Research
Data plays a pivotal role in scientific research, forming the backbone of hypothesis-driven inquiry. Without concrete data, the process of validating or refuting scientific claims becomes tenuous. The fundamental objective of scientific investigation is to obtain accurate and reliable insights, which are achieved through systematic data collection and analysis.
Understanding data's significance in research includes acknowledging its evidential value. Data acts as the foundation for testing hypotheses. Each hypothesis arises from a proposed relationship or observation which needs to be rigorously assessed. Here, quantitative data can reveal patterns that qualitative data might miss. The primary benefit of incorporating data in research is its potential to substantiate claims with empirical evidence. This supports a sound scientific discourse where findings contribute to a larger corpus of knowledge.
Moreover, the interaction of data with scientific methodology cannot be overstated. Methodical data collection allows researchers to maintain consistency and repeatability in experiments. This leads to greater credibility of results, as reproducible findings are a hallmark of robust scientific practice. The integrity of data also affects the broader scientific community, where shared datasets can facilitate collaborative efforts across various fields.
"Data is not just numbers; it’s the evidence that supports scientific revelation."
Another consideration in the importance of data is its influence on decision-making. Data-informed choices, rather than instinctual or anecdotal decisions, tend to yield better outcomes in research. This is particularly crucial in fields like healthcare and environmental science, where data can guide significant policy changes and innovations. In essence, effective and accurate data measurement is not merely a procedural step but a vital component that profoundly shapes the trajectory of scientific inquiry.
In exploring the importance of data, it becomes evident that achieving accuracy and precision is paramount. This involves understanding potential biases, selecting appropriate methodologies for gathering data, and adhering to ethical considerations on data usage. All of these factors interweave to establish a comprehensive framework that underpins successful scientific research.
Role of Data in Hypothesis Testing
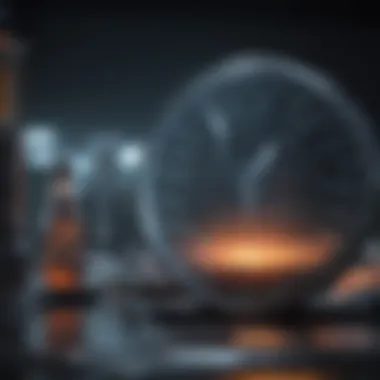
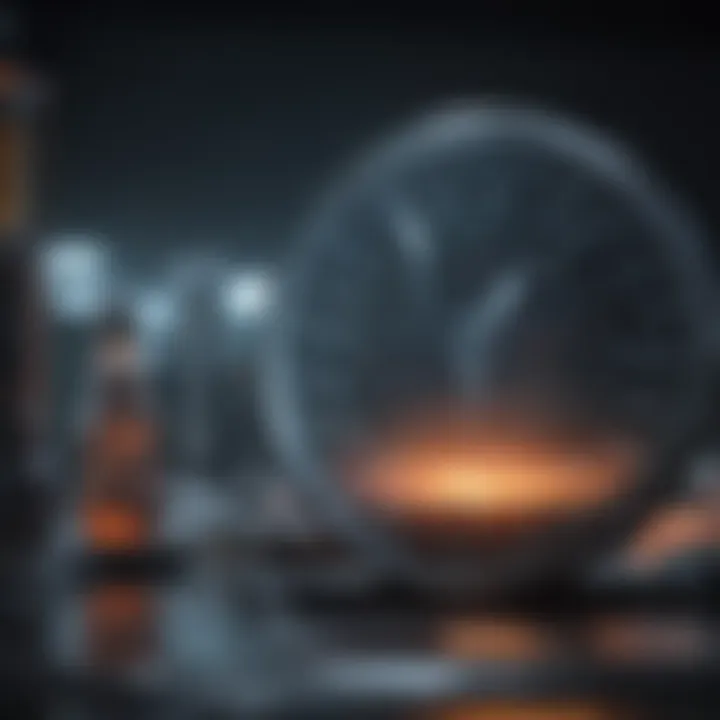
Hypothesis testing is one of the fundamental tasks in scientific research and hinges heavily on the quality of data. It involves formulating a hypothesis based on observations and then collecting data to test its validity. The role of data in this context cannot be understated. It provides the means through which researchers can apply statistical methods to either support or refute their initial claims.
The null hypothesis serves as a critical point of reference, and the collection of data through controlled experiments allows for systematic scrutiny of this hypothesis. For instance, if a researcher posits that a new drug improves recovery rates, the gathered data can be analyzed to determine the statistical significance of the results. In this way, data acts as the decisive element in determining whether the hypothesis holds value.
Furthermore, it is imperative to distinguish between types of data—nominal, ordinal, interval, and ratio—as they each serve different purposes in hypothesis testing. For example, using quantitative data can reveal correlation strengths and enable researchers to employ more complex statistical tests. Conversely, qualitative data can offer insights into context and behavioral aspects, enriching the understanding of results.
Data as Evidence in Scientific Discourse
In scientific discourse, data is often regarded as the most compelling form of evidence. The phrase “data-driven” emphasizes that conclusions drawn should be grounded in solid empirical findings. When researchers present their findings, the underlying data serves to validate their arguments and lend credibility to their claims. This is especially true in peer-reviewed journals, where data presentation directly influences acceptance for publication.
Moreover, the transparency of data is vital for reproducibility, allowing other researchers to verify results. Data sharing can promote collaborative science and expedite knowledge dissemination. When data is shared openly, it can foster an environment that encourages new inquiries and enhances collective understanding across disciplines.
Furthermore, the ethical dimension of data as evidence cannot be overlooked. Researchers must consider not just how data supports their claims but also its collection and representation. Misuse or selective presentation of data can undermine scientific integrity and public trust in research findings. The ethical considerations surrounding data usage emphasize the responsibility researchers have towards maintaining veracity in their work.
In summary, the role of data in hypothesis testing and as evidence in scientific discourse is indispensable. They establish frameworks for inquiry, provide validation for claims, and influence the evolution of scientific thought. Accurate data not only enhances scientific rigor but also cultivates a shared understanding of knowledge across divers fields.
Measurement Techniques
Measurement techniques are foundational in the field of data analysis and scientific research. Understanding the variety of methodologies allows researchers to choose the appropriate approach for capturing and interpreting data. The effectiveness of any research relies heavily on these techniques, which provide the basis for drawing conclusions and validating hypotheses. In this section, we will explore three key areas of measurement techniques: quantitative versus qualitative measurement, statistical methods in measurement, and the impact of technological advancements.
Quantitative vs Qualitative Measurement
Quantitative measurement focuses on numerical data, which can be counted or measured. This form of measurement provides distinct advantages, such as the ability to perform statistical analysis that can uncover patterns and relationships within datasets. Examples of quantitative measurement techniques include surveys with scaled responses, experiments that yield measurable outcomes, and data mining from databases.
In contrast, qualitative measurement deals with non-numerical data. It aims to provide insights into the characteristics, qualities, and subjective experiences associated with a phenomenon. Techniques such as interviews, open-ended surveys, and observational studies characterize this approach. While quantitative methods provide broad, generalizable results, qualitative methods offer depth and context, capturing nuances that numbers sometimes cannot convey.
The choice between these two measurement types often depends on the research question at hand. For instance, a study aimed at understanding the effectiveness of a new drug may require quantitative measurement to assess its impact on patient health, while also incorporating qualitative methods to gain insight into patient experiences. Thus, blending both approaches can yield more comprehensive insights.
Statistical Methods in Measurement
Statistical methods are crucial in transforming raw data into meaningful information. These methods help researchers ensure that the measurements they obtain are reliable and valid. Basic statistics such as mean, median, and mode provide summaries of data, allowing for quick comparison and interpretation.
When direct measurement is impractical, inferential statistics can be used. This involves making predictions about a population based on a sample. Techniques such as confidence intervals, hypothesis tests, and regression analysis allow researchers to understand variability and make informed conclusions based on their sample data.
Moreover, the application of advanced statistical models can enhance measurement precision. Techniques such as multi-variate statistics can manage complex datasets, allowing for a deeper analysis and understanding of interactions between multiple variables. Emphasizing proper statistical methodology is essential to ensure the results can be accepted and utilized in scientific discourse.
Technological Advancements in Measurement
The landscape of measurement has evolved considerably due to technological advancements. Tools and techniques that were previously labor-intensive and time-consuming have become more efficient and accurate with modern innovations. For example, data collection has benefited from automated systems and sensors. These devices can provide real-time data, minimizing human error and significantly increasing the volume of data available for analysis.
The rise of software solutions has revolutionized the field. Programs such as R and Python facilitate complex data analyses through libraries designed specifically for statistical calculations and visualizations. Machine learning algorithms further push the boundaries of traditional measurement approaches by automatically detecting patterns in large datasets.
Also, cloud computing has enabled researchers to store and process vast amounts of data. This availability allows for greater collaboration across disciplines, facilitating comprehensive studies that draw upon diverse datasets. Nonetheless, as technology continues to advance, it is vital to consider the implications of data accuracy, security, and ethical uses of measurement technology.
"The methods of measurement directly impact the reliability of scientific conclusions. Accurate measurement tools ensure research is built on a solid foundation."
In summary, measurement techniques play a critical role in research endeavors. The distinction between quantitative and qualitative methods, the deployment of statistical analysis, and the influence of technological advancements together shape how data is collected, interpreted, and utilized. Each component is essential for rigorous scientific inquiry, highlighting the intricate relationship between measurement and the pursuit of knowledge.
Data Collection and Management
Data collection and management play a crucial role in the scientific research process. Researchers must carefully gather, organize, and analyze data to derive valid conclusions and insights. Effective data collection ensures that information is accurate, comprehensive, and relevant, while robust data management systems support the storage, retrieval, and analysis of this data. Failing to address these areas can lead to misinterpretation or skewed results, ultimately affecting the integrity of the research.
Methods of Data Collection
There are various methods of data collection that researchers can employ, depending on their specific goals and the nature of their study. Some common methods include:
- Surveys and Questionnaires: These tools gather opinions, behaviors, or characteristics of a population. They can be distributed physically or electronically, providing flexibility in reaching a wider audience.
- Interviews: One-on-one interviews yield in-depth data as they allow researchers to dive deeper into participants' thoughts and experiences.
- Observations: Observational studies involve examining subjects in their natural environment. This method can provide insights that are often missed with surveys or questionnaires.
- Experiments: Experimental research involves collecting data through controlled environments, manipulating variables to test hypotheses.
- Secondary Data Sources: Researchers can also utilize existing datasets or literature, which can save time and resources while providing valuable insights.
"Understanding the right method of data collection is integral to ensuring the research outcomes are credible and reliable."
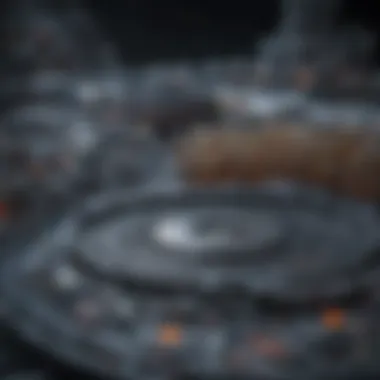
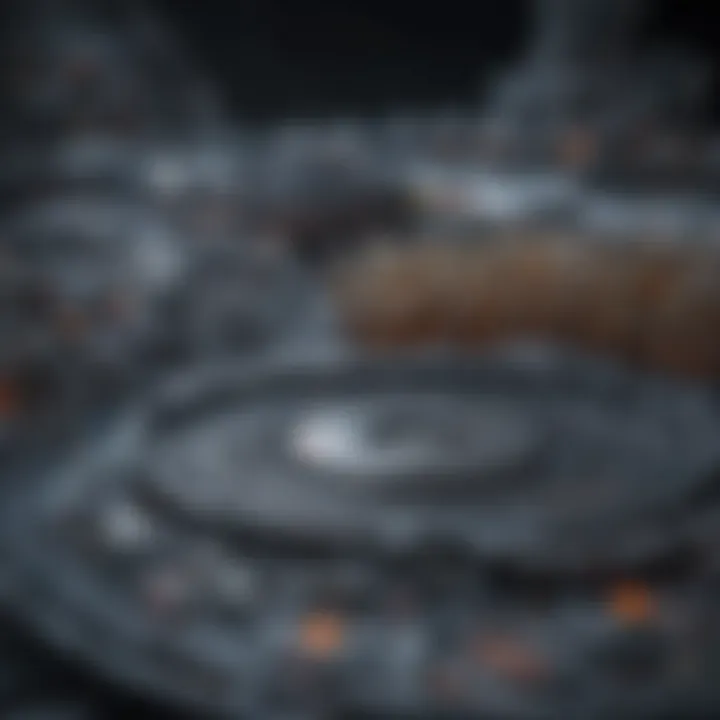
Each method has its own benefits and drawbacks, and the choice often hinges on the research question and the available resources. Integrating multiple methods can enrich the study and provide a more holistic view.
Data Management Systems
Effective data management systems are essential for organizing and storing data in a manner that facilitates easy access and analysis. The components of a good data management system include:
- Data Storage Solutions: Options include cloud storage, local servers, and databases. Each option has its advantages, such as scalability and security features.
- Data Catalogs: These systems help in organizing data by creating metadata entries, making it easier for researchers to locate relevant data.
- Data Analysis Tools: Software such as R, Python, or specialized statistical packages allow researchers to analyze data efficiently.
- Data Backup Protocols: Regular backups are critical to prevent data loss due to hardware failures or other unforeseen events.
Having a systematic approach to data management is essential, as it enhances the reproducibility of research findings and supports ongoing data sharing within the scientific community.
Data Privacy and Ethical Considerations
With the increasing amount of data collected, it is vital for researchers to consider data privacy and ethical implications. Key points include:
- Informed Consent: Participants must understand how their data will be used. This builds trust and ensures compliance with ethical standards.
- Data Anonymization: Researchers should make efforts to anonymize personal data to protect participants' identities.
- Regulatory Compliance: Familiarity with regulations such as the General Data Protection Regulation (GDPR) helps avoid legal issues and fosters accountability.
- Ethical Review Boards: Submitting research proposals to an ethics committee ensures that data collection methods meet ethical standards and address potential biases.
These considerations not only protect individuals but also uphold the credibility of the research process. Adhering to ethical standards is vital for advancing knowledge while maintaining public trust in scientific inquiry.
Challenges in Data Measurement
Data measurement, albeit critical to scientific research, presents a myriad of challenges that can impact the validity and reliability of findings. These challenges do not merely stem from technical difficulties but also speak to the ethical implications of how data is gathered, analyzed, and interpreted. Understanding these challenges is essential for researchers and practitioners in order to ensure the integrity of scientific inquiry.
First, leveraging accurate data is pivotal. However, the introduction of biases during any stage of data collection and measurement can distort conclusions, leading to erroneous insights. This fact necessitates a rigorous examination of methodologies prior to implementation. Rigorous methodologies not only promote accuracy but also ensure that the data reflects the real-world phenomena it attempts to measure.
Moreover, issues such as precision and accuracy frequently arise. It is not uncommon for researchers to confuse these two terms, leading to misunderstandings that can significantly alter the interpretation of results. Proper training and a clear understanding of these concepts can prevent misinterpretations that undermine research quality.
Henceforth, addressing these challenges is essential as it builds a strong foundation for reliable scientific research. An understanding of data bias, the distinction between precision and accuracy, and the ethical considerations surrounding these topics can enhance the robustness of findings. This section will delve into the aspects of data bias and precision versus accuracy, illuminating the complexities researchers must navigate in their quest for reliable data.
Data Bias and its Impacts
Data bias refers to systematic errors that lead to inaccurate conclusions in scientific measurement and analysis. It can manifest during the data collection phase, analysis, or even while interpreting results. Such biases can be introduced in various forms, including sampling bias, measurement bias, and confirmation bias. Each type of bias can severely impact the research findings.
- Sampling Bias: Occurs when the sample chosen is not representative of the larger population, potentially skewing results.
- Measurement Bias: Arises from flawed instruments or procedures that consistently misrepresent measurements.
- Confirmation Bias: Results when researchers favor information that supports their hypotheses, consciously or unconsciously ignoring contrary data.
Addressing data bias is paramount. Researchers must implement strategies such as random sampling, ensuring calibration of measurement instruments, and conducting peer reviews. Failure to tackle data bias can lead to flawed conclusions, undermining the credibility of the entire study.
"Data bias undermines the very foundation of scientific integrity and must be meticulously addressed to ensure valid research outcomes."
Precision vs Accuracy
Precision and accuracy are oftentimes used interchangeably, yet they hold distinct meanings in the realm of data measurement. Accuracy refers to how close a measured value is to the true value, while precision indicates the repeatability or consistency of measurements.
In scientific measurement, achieving both high precision and high accuracy is ideal. However, these two concepts can sometimes be at odds. One can obtain precise measurements that are inaccurate if the measuring instrument is miscalibrated. Conversely, an accurate measurement may lack precision if varied conditions disrupt consistency.
Understanding the difference between accuracy and precision is crucial. Researchers must ensure that their methodologies promote both aspects. This involves:
- Employing calibrated instruments.
- Establishing clear measurement protocols.
- Conducting multiple trials to ascertain reliability.
By fostering a thorough comprehension of these concepts, researchers can enhance the credibility of their findings. Misinterpretations stemming from poor understanding can lead to consequences that affect not only individual studies but also broader fields of inquiry.
Case Studies from Various Disciplines
Understanding the practical application of data and measurement through case studies is essential for grasping their impact on scientific inquiry. Case studies enable researchers and students alike to see how theoretical frameworks translate into real-world scenarios. These examples not only provide a clinical view of methods and outcomes but also emphasize the importance of accurate measurement. By analyzing cases from various disciplines, we can appreciate the specificity needed in different contexts, examine failures and successes, and derive insights that contribute to best practices in research methodologies.
Biological Research: Measurement of Growth Rates
In biological research, measuring growth rates can be critical for various investigations, from understanding species ecology to agricultural productivity. Precise growth measurements allow scientists to evaluate how organisms respond to different environmental conditions, genetic variations, or treatment applications.
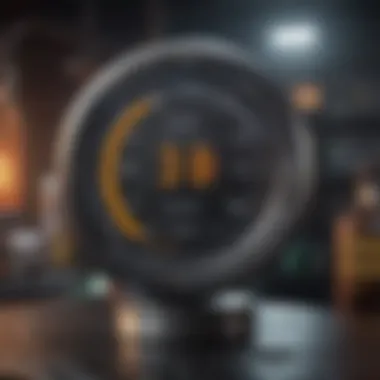
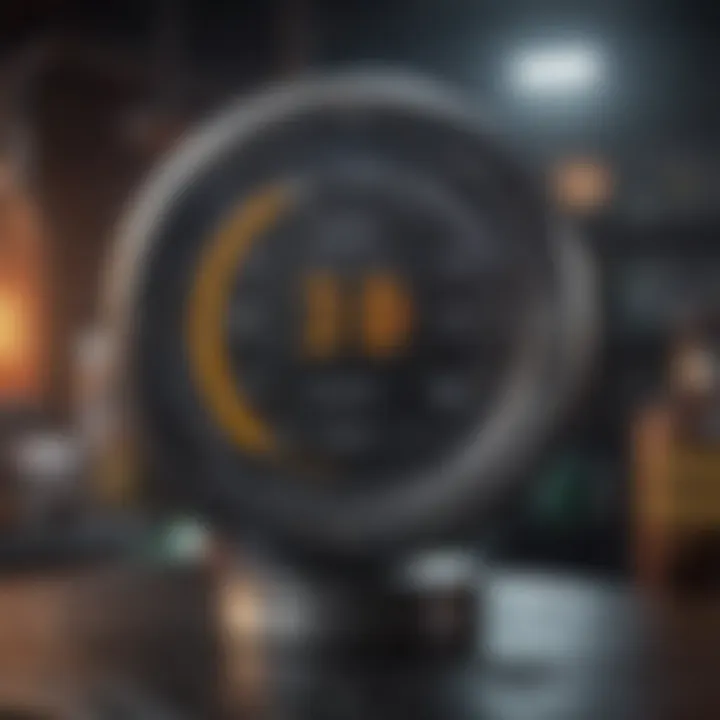
When measuring growth rates, various metrics may be employed, such as length, biomass, or population size. For instance, in developing agricultural crops, researchers often focus on quantifying the increase in height over specific time intervals. This measurement helps in understanding the effectiveness of different fertilizers or irrigation techniques.
In the laboratory, methodologies such as calipers or image analysis software can be used for accuracy. Those methods minimize human error and provide replicable results. Biologists also benefit from statistical tools, allowing them to analyze data trends over time, deepen understanding about growth patterns, and make predictions about future growth.
Chemical Analysis: Quantifying Substance Concentrations
In chemical analysis, quantifying substance concentrations is pivotal for both quality control in industries and research laboratories. Whether measuring pollutants in environmental samples or determining active ingredients in pharmaceuticals, accurate concentration measurement informs decisions and regulatory compliance.
Techniques such as spectrophotometry or chromatography are commonly employed to obtain these measurements. For example, in determining the concentration of a drug in blood plasma, scientists can use high-performance liquid chromatography (HPLC). The precision of these methods is often crucial since even small variances can lead to major differences in outcome.
Moreover, presenting concentration data accurately is vital for stakeholders, ranging from scientists to policymakers. This data dictates regulations for safe levels of contaminants in drinking water or pharmaceutical efficacy, underscoring the need for stringent measurement practices.
Environmental Studies: Measuring Ecological Impact
Environmental studies focus on measuring ecological impact to understand the effects of human activities on ecosystems. This involves assessing changes in biodiversity, pollution, and habitat degradation. For instance, long-term ecological studies often employ a measure of species diversity in an area to gauge health of an ecosystem.
Common metrics include species richness, abundance, and evenness. These indicators help in assessing the effects of logging, mining, or urban development. Here, methodologies such as remote sensing or geographical information systems (GIS) can enhance the capability to measure changes over wide areas and over time.
Moreover, managing and interpreting these measurements is essential for environmental policy making. By understanding ecological impact through rigorous measurement, researchers can advocate for conservation strategies and more sustainable practices, leading to better stewardship of natural resources.
"Accurate measurements in ecological studies not only inform scientists but shape policies that impact communities and stewardship of the planet."
The integration of these case studies illustrates the diversity and depth of data measurement across various fields. They provide a practical lens through which to view the theories and methodologies discussed in more academic contexts, bridging the gap between theory and application.
Future Trends in Data and Measurement
The landscape of data and measurement is continuously evolving. This evolution is driven by technological advancements, shifts in methodologies, and the increasing importance of data accuracy in scientific research. Understanding future trends allows researchers to adapt and optimize their approaches, ensuring integrity and effectiveness in their work. Moreover, as we look ahead, it becomes vital to consider how these trends may reshape various fields and influence decision-making processes.
Emerging Technologies: AI and Machine Learning in Data Analysis
Artificial Intelligence (AI) and machine learning (ML) are playing significant roles in data analysis. These technologies enable researchers to process vast amounts of data with greater efficiency than traditional methods. For instance, AI algorithms can recognize patterns in complex datasets, providing insights that might be missed by human analysis. Furthermore, machine learning models can improve over time, adapting to new data and refining their predictions.
- Automated Data Processing: AI can automate tasks such as data cleaning and normalization, saving researchers time and effort while reducing the risk of human error.
- Enhanced Predictive Analytics: With machine learning, researchers can deploy models that predict outcomes based on historical data, aiding in hypothesis testing and experimental design.
- Personalized Research Approaches: AI enables tailored methodologies, allowing for more specialized analyses suited to specific research questions.
These advancements also raise considerations around training datasets and potential biases in the algorithms. Hence, transparency and ethical considerations in AI and ML must remain at the forefront of their application in scientific research.
Big Data and Its Implications for Measurement
Big data refers to data sets that are so large or complex that traditional data processing applications cannot handle them. The implications for measurement are profound.
- Volume: The sheer volume of data means that researchers must employ new strategies for data collection and analysis to derive meaningful insights.
- Variety: Big data encompasses diverse formats, from structured spreadsheets to unstructured text and multimedia. Measurement techniques must evolve to accommodate this variety effectively.
- Velocity: Data flows in at an unprecedented speed. Researchers must react quickly, creating systems that can process and analyze data in real time.
Incorporating big data in measurement enhances accuracy but also introduces challenges, such as ensuring data quality and relevance. The analyst's ability to discern significant information from the noise is crucial in leveraging big data effectively.
"The increasing reliance on big data compels scientists to refine their measurement frameworks, ensuring robust and ethical analysis."
Ending
The conclusion in any comprehensive examination of data and measurement serves as a pivotal point that encapsulates the essence of the discussions preceding it. It synthesizes various themes explored throughout the article, allowing readers to grasp the overarching significance of data and measurement across diverse scientific domains. This section reinforces the value of rigorous methodologies, accuracy, and ethical considerations that are paramount in research.
Summary of Key Insights
In summation, the interplay between data and measurement is essential in fostering scientific progress. Several key insights emerge:
- Data as the foundation of inquiry: The validity of research findings hinges on the quality and integrity of the data collected.
- Measurement techniques matter: Both quantitative and qualitative methods have distinct roles and implications for research outcomes.
- Technological advancements: Innovations, particularly in areas like AI and machine learning, have revolutionized data analysis, creating new opportunities and challenges for measurement.
- Ethics and data privacy: As data collection grows, so do concerns about privacy and the ethical use of information, which must be addressed in any research framework.
- The influence of accuracy: Precision in measurement directly correlates with the reliability of scientific conclusions, affirming that attention to detail cannot be overstated.
Understanding these insights is crucial for students, researchers, educators, and professionals who aim to engage meaningfully with data and measurement practices.
The Ongoing Evolution of Data and Measurement
The landscape of data and measurement is in a constant state of flux, evolving with technological advancements and societal demands. As new tools and methodologies are developed, researchers must adapt their practices to integrate these innovations effectively. For instance, the rise of big data necessitates novel strategies for analysis, prompting the development of sophisticated statistical methods and data management systems. Additionally, the incorporation of artificial intelligence in analyzing vast datasets showcases a paradigm shift that influences how measurements are taken and interpreted.
Moreover, the conversation around ethical practices continues to grow, challenging researchers to be vigilant about the implications of their work. With the advent of new technologies, considerations around data privacy and ethical usage become ever more significant.
Continued exploration in this realm is essential, as the ability to measure accurately will determine the direction and impact of scientific inquiry in the future. Drawn by these currents of change, the journey of data and measurement unfolds, revealing new frontiers for exploration and understanding.