Exploring Decision Support Systems in Healthcare
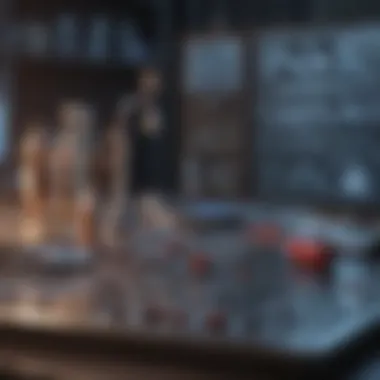
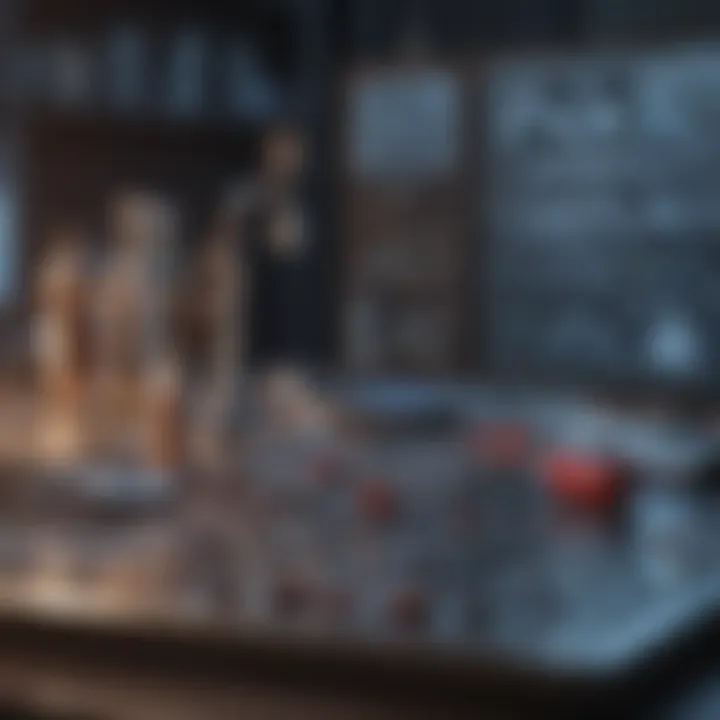
Intro
In today's rapidly evolving healthcare environment, decision support systems (DSS) are of utmost importance. These systems play a vital role in clinical decision-making by providing healthcare professionals with important data and insights. By leveraging technology, DSS enhances patient care, streamlines operations, and contributes to a more efficient healthcare system.
The impact of decision support systems is multi-faceted. They are designed to assist in a variety of functions, which can enhance diagnostic accuracy and improve treatment outcomes. Furthermore, these systems address not only technological aspects but also ethical considerations, thereby offering a holistic view of their role in modern medicine.
Understanding the significance of DSS requires a closer look at their various types, functionalities, and real-world applications. This article discusses the critical components of decision support systems and their contribution to healthcare innovation, making it essential reading for anyone engaged in this field.
Intro to Decision Support Systems
In the complex landscape of healthcare, making informed decisions is crucial. Decision support systems (DSS) play a pivotal role in guiding healthcare professionals through these complexities. These systems integrate data, knowledge, and analytical tools to assist in clinical decision-making. By leveraging technology effectively, DSS improve patient care, streamline processes, and can enhance overall operational efficiency within healthcare institutions. Understanding the significance of these systems is essential, as they take a pivotal position in modern healthcare practices.
Definition and Purpose
Decision support systems are computer-based tools designed to aid human decision-making. Their primary purpose is to provide clinicians with crucial information that can enhance patient care. This information can range from patient histories and clinical guidelines to diagnostic criteria and treatment plans. By having access to a wealth of knowledge, healthcare workers can make decisions that are backed by evidence rather than solely relying on experience or intuition.
DSS can be categorized into several types based on their functionality. Clinical decision support systems focus specifically on patient care, while operational decision support systems may analyze data related to healthcare management. Both contribute significantly to improving outcomes and enhancing the quality of care.
Historical Background
The development of decision support systems in healthcare is deeply rooted in advancements in technology. Initially, the concept of decision support emerged in the 1970s, primarily within fields such as business and management. Gradually, its potential became evident in healthcare settings. Early systems were often simplistic, relying on basic algorithms and rule-based processes.
Over decades, advancements in information technology dramatically shifted the landscape. The integration of electronic health records and data mining techniques in the late 1990s and early 2000s marked significant milestones. Systems became more sophisticated, allowing for real-time data analysis and predictive modeling. As a result, DSS gained traction, transforming from basic tools into essential resources for clinicians. This evolution continues today, as emerging technologies like artificial intelligence and machine learning integrate further into decision support systems.
Types of Decision Support Systems
Understanding the different types of decision support systems (DSS) is crucial for appreciating their role in healthcare. Each type of DSS serves a specific purpose, addressing unique challenges within clinical and operational settings. They enhance efficiency, promote evidence-based practices, and ultimately aim to improve patient outcomes.
Clinical Decision Support Systems
Clinical Decision Support Systems (CDSS) are pivotal in aiding healthcare providers. Their main function is to translate complex patient data into actionable insights. This assists clinicians in making better informed decisions about diagnosis and treatment options. CDSS can integrate patient history, symptoms, and even genetic information to suggest relevant interventions.
One significant benefit of CDSS is the reduction in diagnostic errors. By analyzing a variety of data points, these systems can highlight potential issues that may be overlooked by human practitioners. Moreover, they can also present evidence-based recommendations which enhances the quality of care. By encouraging adherence to clinical guidelines, CDSS promotes effective health management.
Operational Decision Support Systems
Operational Decision Support Systems focus more on the managerial aspects of healthcare institutions. These systems optimize resource allocation, scheduling, and operational planning. For example, they can analyze patient flow through a hospital or clinic and suggest adjustments to staffing levels to meet demand.
Such systems significantly enhance efficiency. This is especially important in emergency situations where timely decisions are crucial. Operational DSS can help healthcare administrators to predict peak times and manage operational budgets.
Data-driven Decision Support Systems
Data-driven Decision Support Systems leverage vast amounts of data collected from various sources. These systems analyze trends, patterns, and correlations that can improve healthcare services. By utilizing advanced data analytics and algorithms, they provide insights into patient care, treatment efficiencies, and outcomes.
The integration of data-driven DSS in healthcare allows for predictive analytics, where possible future events are anticipated based on historical data. This capability is vital in preventative health strategies. For instance, identifying patients at risk for chronic conditions allows for early interventions that can lead to improved health outcomes.
Components of Decision Support Systems
Decision Support Systems (DSS) in healthcare comprise several key components that work together to enhance the decision-making process. Understanding these components is vital as they not only define how effective the system will be, but also play a crucial role in improving patient outcomes and operational efficiency. The primary components include data management, knowledge base, and processing mechanisms. Each part holds significance in shaping the functionality and effectiveness of DSS.
Data Management

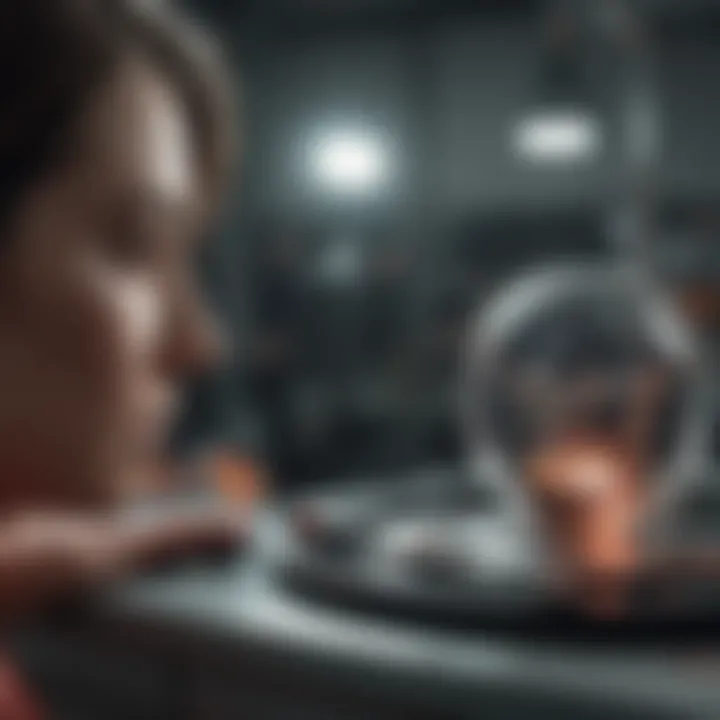
Data management serves as the backbone of any Decision Support System. It involves the collection, storage, and retrieval of relevant information that healthcare professionals need for informed decisions. Data can come from various sources, including electronic health records (EHR), patient surveys, and clinical databases. Here are some key aspects:
- Data Quality: Ensuring high-quality data is essential. Poor data can lead to incorrect conclusions, potentially harming patient care.
- Data Integration: DSS must integrate data from different systems to provide a comprehensive view. This integration supports holistic decision-making.
- Data Security: With sensitive patient information at stake, robust security measures are necessary. Protecting data against breaches upholds patient trust and complies with regulations.
Overall, effective data management enhances the reliability of the DSS and ensures that healthcare professionals have access to accurate information when needed.
Knowledge Base
The knowledge base is another critical component of Decision Support Systems. It encompasses the rules, algorithms, and best practices that guide clinical and operational decisions. This base draws on extensive medical literature, case studies, and clinical guidelines. Important features include:
- Up-to-Date Information: The knowledge base must be frequently updated to reflect the latest research and standards of care. This adaptability ensures that healthcare providers access current guidance.
- Clinical Guidelines: A well-structured knowledge base should include evidence-based guidelines that clinicians can utilize for diagnoses and treatment options.
- Expert Systems: In some advanced DSS, expert systems leverage artificial intelligence to simulate human expertise. They can suggest diagnoses or recommend treatments based on patient data.
The quality of the knowledge base directly impacts the accuracy and reliability of the support provided, making this component crucial in healthcare.
Processing Mechanisms
The processing mechanisms of Decision Support Systems establish how data is analyzed and how insights are generated. This component defines the system's ability to interpret data and produce actionable recommendations. Key elements of processing mechanisms include:
- Algorithms and Models: Algorithms are essential for analyzing data and identifying patterns. Employing sophisticated models helps predict outcomes and recommend interventions.
- Real-Time Processing: Some DSS offer real-time analytics, allowing instant insights during patient encounters. This capability is imperative for urgent decision-making.
- User Interfaces: An intuitively designed user interface facilitates easier interaction with the system, enhancing the usability of DSS for healthcare professionals.
In summary, effective processing mechanisms maximize the system’s potential, providing timely and relevant support to clinicians.
"The integration of effective components in Decision Support Systems not only enhances efficiency but also aligns efforts towards better patient care outcomes."
Benefits of Decision Support Systems in Healthcare
Decision support systems (DSS) have evolved significantly in healthcare, becoming integral tools that enhance both the quality of care and operational efficiency. Their implementation carries multiple benefits that can transform how healthcare providers make decisions. Understanding the importance of these systems offers insights into their potential to impact patient care positively.
Improved Patient Outcomes
One major benefit of decision support systems is their ability to improve patient outcomes. These systems assist healthcare professionals in diagnosing and treating patients more effectively. For instance, Clinical Decision Support Systems provide recommendations based on patient data and clinical guidelines. As a result, physicians can make more informed decisions, minimizing the risk of misdiagnosis.
Research has shown that when clinicians utilize DSS, the quality of care tends to improve. This is because DSS often incorporates evidence-based guidelines that reflect the latest medical research. Thus, treatments personalized to individual patient needs can be developed more readily. This leads to a higher adherence to treatment protocols, which ultimately enhances recovery rates.
Additionally, DSS can help prevent adverse events. By identifying contraindications and potential medication interactions, these systems act as a safety net. They ensure that critical information is available at the point of care, reducing the likelihood of errors that can compromise patient safety.
"Incorporating decision support systems has transformed clinical practice, leading to significant advancements in patient care delivery."
Enhanced Efficiency
Another key advantage of decision support systems is enhanced efficiency in healthcare operations. By automating routine tasks and optimizing workflows, DSS allows healthcare providers to allocate their time more effectively. For example, electronic health records (EHR) integrated with DSS provide quick access to patient information. This speeds up the decision-making process and decreases the time spent searching for critical data.
Moreover, these systems help in resource management. By analyzing data trends, they can assist in forecasting demand for services, enabling better staff allocation and resource utilization. In turn, this can reduce wait times for patients and enhance their overall experience within healthcare settings.
In emergency situations, rapid access to decision support tools can be a matter of life or death. DSS provides real-time data analysis and collaborative features, promoting faster responses in critical care scenarios. Hence, the operational dynamics of healthcare facilities improve.
Support for Evidence-Based Practice
Decision support systems fundamentally support evidence-based practice within healthcare. These systems synthesize a vast amount of data, making it easier for healthcare providers to adhere to established best practices. Consequently, decision-making shifts towards an informed basis, relying on accurate and timely data rather than solely on intuition or outdated practices.
The implementation of DSS can lead to standardized practices across healthcare providers. When physicians rely on data-driven recommendations, it fosters uniformity in treatment approaches. This is especially vital in large healthcare organizations where multiple providers may be involved in patient care.
In addition, the integration of DSS into healthcare promotes continuous learning. As these systems evolve, they incorporate new research findings, keeping practitioners up-to-date with advancements in medical science. This continuous learning environment ensures that healthcare delivery remains relevant and beneficial to patients.

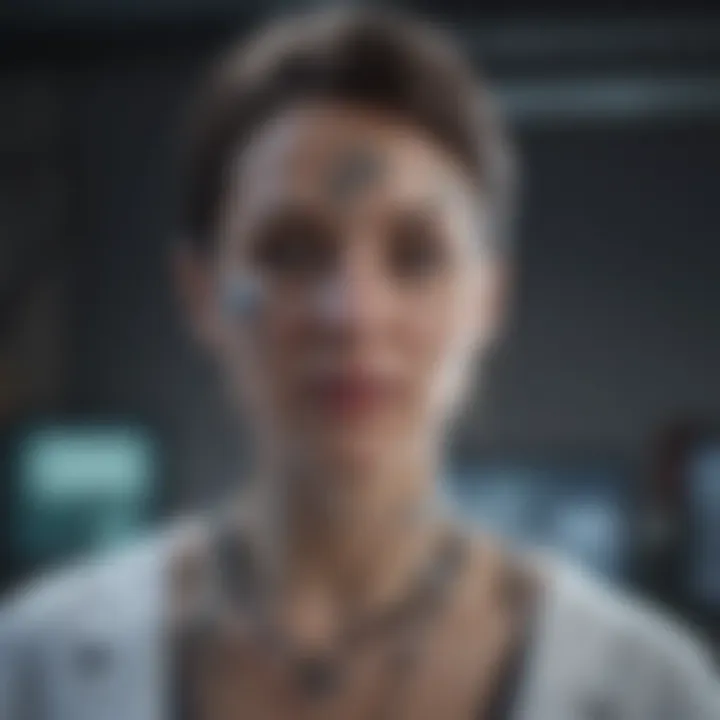
In summary, the benefits of decision support systems in healthcare span across improved patient outcomes, enhanced efficiency, and robust support for evidence-based practice. Their transformative potential underscores the necessity for ongoing investment and development in healthcare technology.
Challenges in Implementing Decision Support Systems
Implementing Decision Support Systems (DSS) poses unique challenges that can significantly influence their efficacy in healthcare settings. Understanding these hurdles is crucial for healthcare organizations striving to enhance efficiency and improve patient outcomes. The challenges are often intertwined with technological, organizational, and human factors that require careful navigation. Each challenge is important as it highlights the need for strategic planning and focused efforts to ensure successful deployment.
Integration with Existing Systems
One of the primary challenges is the integration of DSS with existing healthcare technologies. Health systems often rely on a variety of software solutions, including Electronic Health Records (EHRs) and practice management systems. The complexity arises from the need for seamless interoperability between these different platforms. Without proper integration, data silos can occur, leading to incomplete or inaccurate patient information.
This situation can result in missed opportunities for decision support. If data is not effectively shared between systems, the DSS may not have access to crucial patient history, medications, or diagnostic data. Thus, clinical staff may not leverage the full potential of the DSS during decision-making.
"The ability to integrate systems is not just a technical issue; it is also a matter of clinical workflow and user experience."
Strategies for overcoming integration challenges often involve investing in standardized communication protocols, such as HL7 or FHIR. These standards can facilitate smoother data exchange, allowing DSS to operate effectively within the broader healthcare ecosystem.
User Acceptance and Training Needs
User acceptance plays a pivotal role in the successful implementation of DSS. Healthcare professionals must trust and feel comfortable using these systems. If the users lack confidence in the system's recommendations or find the interface cumbersome, they are less likely to utilize it in practice. This creates an ongoing challenge where the potential benefits of the DSS are not realized.
Training is a critical element that can bridge the acceptance gap. Organizations must invest time and resources in comprehensive training programs. These programs should cover not only how to use the DSS but also its advantages. When users understand how a DSS can enhance their clinical decision-making and improve patient care, they are more inclined to embrace the change.
Data Quality and Availability
Data quality is essential for the effectiveness of any decision support system. Poor data quality can lead to flawed recommendations, which may compromise patient safety and the overall integrity of healthcare services. In many cases, healthcare providers face difficulties in maintaining high standards for data entry and management.
Moreover, the availability of data can be a significant issue. Inadequate data can stem from inconsistent data entry practices, lack of standardized medical terminology, or simply insufficient data collection. DSS that rely on incomplete or erroneous data can exacerbate the existing challenges in decision-making processes.
To address these concerns, organizations must focus on establishing data governance protocols. This includes ensuring data accuracy, consistency, and timeliness. Regular audits and continuous training on data management can help maintain high data quality, which in turn enhances the effectiveness of DSS in healthcare settings.
In summary, the challenges encountered during the implementation of Decision Support Systems must not be overlooked. Each aspect, from integration to user acceptance to data quality, plays a vital role in determining the overall success of these systems. Addressing these challenges is not only beneficial but essential for optimizing healthcare delivery.
Real-World Applications of Decision Support Systems
The integration of decision support systems into healthcare settings provides significant advantages that enhance both decision-making and patient care. Real-world applications of these systems demonstrate their ability to improve clinical outcomes, streamline processes, and facilitate research. Understanding these applications is essential because they showcase the practical impact of decision support systems on the healthcare ecosystem. The various implementations also address specific needs within clinical and operational settings, making them invaluable tools for healthcare providers.
Case Studies in Clinical Settings
In clinical environments, decision support systems have been pivotal in transforming patient interactions and treatment protocols. Many hospitals utilize clinical decision support systems (CDSS) to assist physicians in diagnosing and treating patients more effectively. For instance, the implementation of UpToDate as a CDSS allows healthcare providers to access evidence-based clinical information at the point of care. This immediate access enables quicker decision-making regarding treatment options, thus elevating standards of care.
Consider the case of a hospital that integrated a CDSS focused on sepsis management. This system utilizes real-time patient data, including vital signs and laboratory results, to alert clinicians of possible sepsis. In a study, the implementation led to a significant reduction in sepsis-related mortality. By alerting healthcare professionals early in the process, the CDSS acts as an effective safety net—not only enabling better patient outcomes but also fostering collaboration among the medical team.
Such case studies highlight the efficacy of decision support systems in optimizing clinical protocols and enhancing the quality of care.
Operational Use in Health Management
Beyond clinical settings, decision support systems also play a crucial role in operational health management. These systems provide administrative support that can lead to more efficient healthcare delivery. A notable example includes the use of predictive analytics in managing patient flow in emergency departments. By analyzing historical patient data and patterns, systems can forecast patient volumes, allowing hospitals to allocate staff and resources more effectively. This reduces wait times and improves patient satisfaction.
Another application is found in scheduling systems, which utilize algorithms to optimize appointment management. For instance, systems like QGenda help practices ensure that they meet demand without overbooking slots. As a result, clinics can enhance patient access while also optimizing their resources—an essential aspect of operational efficiency.
The successful execution of these systems showcases their versatility in addressing both clinical and operational challenges within healthcare settings. It emphasizes the necessity for ongoing adaptations and integrations of decision support systems to positively influence healthcare delivery in the modern era.
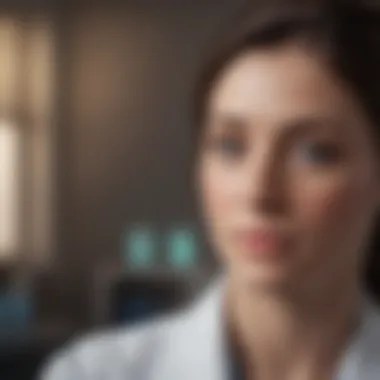
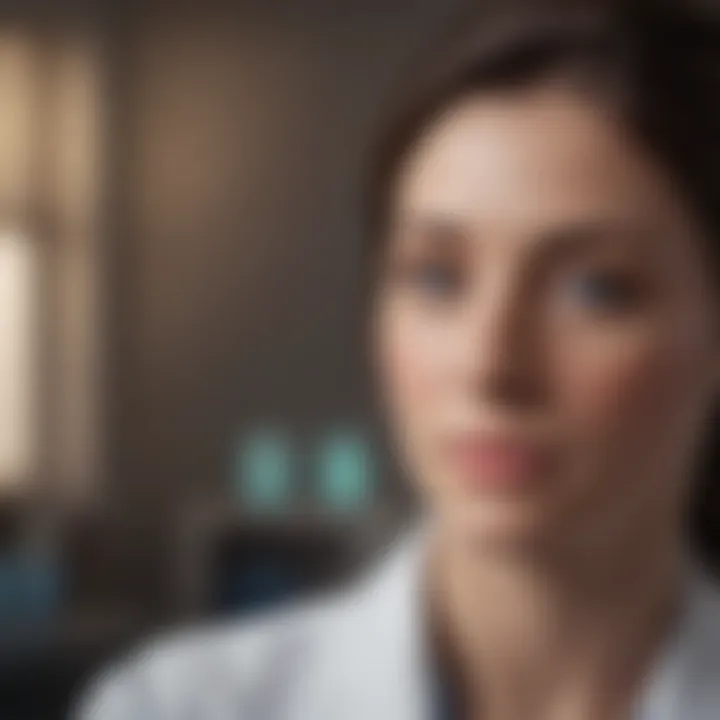
"The increasing reliance on decision support systems is not only about technology; it's about improving patient-centric approaches in healthcare delivering better outcomes for all involved."
As healthcare continues to evolve, the real-world applications of decision support systems will remain a focal point for any serious dialogue concerning the future of health management and patient care.
Future Trends in Decision Support Systems
The evolution of healthcare technology is profoundly shaped by decision support systems (DSS). As the systems gain traction, future trends will likely focus on enhancing their capabilities. These trends are critical not only for the advancement of healthcare operations but also for the quality of patient care. In this section, we will discuss two significant trends: artificial intelligence integration and personalized medicine applications.
Artificial Intelligence Integration
Artificial intelligence (AI) represents a fundamental shift in the capabilities of decision support systems. AI can analyze vast amounts of data quickly and efficiently. This integration leads to better diagnostic accuracy and personalized treatment recommendations. One key benefit is the ability to predict patient outcomes based on historical data. For example, machine learning algorithms can identify patterns in patient records that may not be evident to human practitioners.
Moreover, AI can enhance clinical workflows. By automating routine tasks and assisting healthcare professionals in clinical decision-making, AI reduces their cognitive load. This support allows them to focus on complex cases requiring human judgment. Robust AI systems can adapt over time, learning from new data inputs. This adaptability contributes to continuous improvement in their decision-making processes.
However, the integration of AI also poses challenges. There are concerns about bias in algorithms, particularly if training data lacks diversity. Ensuring fairness in AI-driven decisions is essential. Ongoing evaluations and updating the algorithms are crucial to address these biases, fostering equal healthcare access.
Personalized Medicine Applications
Personalized medicine represents another significant trend in decision support systems. This approach tailors medical treatment to individual characteristics. With advances in genomics and biotechnology, healthcare providers can offer therapies based on patients' genetic profiles. Decision support systems play a pivotal role in this customization.
DSS can aggregate and analyze genetic data alongside clinical information to provide actionable insights. For instance, they may suggest the most effective treatment for a patient suffering from a specific condition by matching it with genetic predispositions. This capability not only enhances treatment efficacy but also minimizes the risk of adverse reactions that may arise from standard treatments.
The implications of personalized medicine extend beyond individual care. They offer insights into population health management. By analyzing aggregated data, decision support systems can identify health trends and emerging diseases within specific demographics. Consequently, healthcare organizations can allocate resources more efficiently and develop preventive strategies tailored to the community.
Ethical Considerations in Decision Support Systems
Ethical considerations in decision support systems (DSS) play an important role in the implementation and usage of these systems. As these technologies continue to develop and become more integrated into healthcare, the ethical challenges become increasingly significant. Understanding bias, fairness, privacy, and data security is essential for practitioners and stakeholders alike. These considerations not only impact patient outcomes but also shape trust in technology and the healthcare system as a whole.
Bias and Fairness Issues
Bias in decision support systems can arise from various factors in data collection and algorithm design. If the underlying data is not representative of the entire patient population, the outcomes can favor certain groups over others, leading to unequal treatment. For example, a system trained on data predominantly from one demographic may misinterpret or overlook the needs of others.
The consequences of bias can be severe. Inequitable access to treatments or misdiagnoses can occur, which ultimately affects patient care and outcomes. It is crucial for healthcare professionals to scrutinize the algorithms and datasets used in these systems. Continuous monitoring and refinement of models are necessary to minimize biases. This effort should also include diversity among teams that develop and implement these systems.
"Data is not just numbers; it's the stories of real lives – and those stories must be told fairly."
Patient Privacy and Data Security
Patient privacy and data security represent another critical ethical concern. DSS often utilize sensitive information to function effectively. This includes personal health data, treatment history, and genetic information. Any breach of this data can lead to severe consequences, including identity theft or discrimination.
Healthcare organizations must prioritize strong data protection protocols. These should include encryption, access controls, and regular audits. Moreover, transparency with patients about how their data is used is vital to maintaining trust. Patients should feel confident that their information will not be misused or inadequately protected.
The End: The Future of Decision Support Systems in Healthcare
In the rapidly evolving landscape of healthcare, Decision Support Systems (DSS) play an increasingly critical role. They are not merely tools, but essential components that shape how medical decisions are made. Their future is intertwined with technological advancements and societal needs. The integration of these systems presents both opportunities and challenges, requiring careful consideration of their impact.
Summary of Key Insights
Decision Support Systems have shown significant promise in enhancing clinical decision-making. Their capabilities to analyze large datasets and provide insights can lead to improved patient outcomes and operational efficiency. The emergence of artificial intelligence and machine learning further amplifies these advantages by enabling personalized treatment plans and predictive analytics.
Key insights include the following:
- Adaptability: DSS must evolve with advances in technology to meet changing healthcare needs.
- User-centered design: Systems should be developed with input from healthcare providers to ensure practicality and effectiveness.
- Ethical frameworks: Addressing bias and ensuring patient privacy remain paramount.
Implications for Stakeholders
The implications of Decision Support Systems are vast and require attention from multiple stakeholders in healthcare. Healthcare providers, patients, and policymakers all play essential roles in the implementation and success of these systems.
- Healthcare Providers: Must engage in continuous training to adapt to new technologies while ensuring user acceptance.
- Patients: Can expect more personalized care, benefiting from tailored treatment plans derived from DSS analytics.
- Policymakers: Need to establish guidelines that address ethical considerations like data privacy and security.