Key Lessons from Artificial Intelligence: Insights and Impact
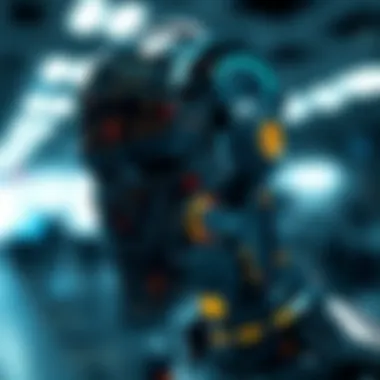
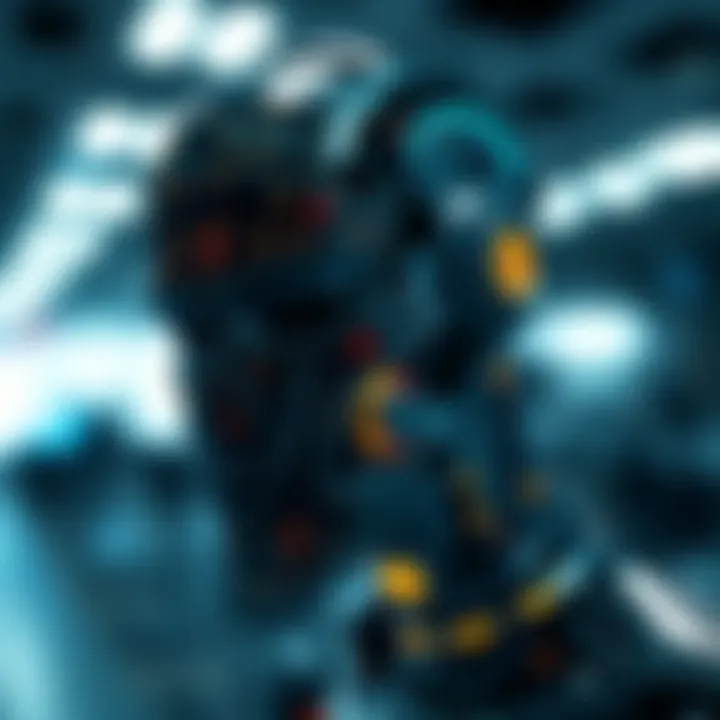
Intro
Artificial intelligence (AI) isn’t just a buzzword tossed around tech conferences and classroom lectures; it’s reshaping the fabric of industries and societies alike. From healthcare to finance, the lessons we've gleaned from AI applications are not merely academic but also vastly practical. What’s often overlooked, though, is the depth and nuance these lessons offer. These range from understanding ethical implications to recognizing the need for skilled interdisciplinary collaboration.
In this article, we delve deep into the essential takeaways that stem from the evolution of AI technologies. We’ll look closely at educational pathways, ethical considerations, and the practical uses that are transforming everyday sectors into highly efficient machines. By exploring the societal ramifications and driving forces behind AI, we aim to paint a comprehensive picture that informs our readers of both the potentials and pitfalls of these ever-advancing technologies.
It's a complex landscape we're navigating. While AI presents solutions, it also raises questions about ethics, privacy, and the future of work. Adopting AI responsibly is not just advisable; it’s essential. Understanding these lessons can pave the way for future developments that are not only innovative but grounded in ethicality and awareness.
In the following sections, we will unpack the research highlights that illuminate these insights, offering you the chance to explore the depth of what AI has taught us thus far.
Prelude to Artificial Intelligence Lessons
In the realm of technology, artificial intelligence stands as a beacon of progress, illuminating paths across various sectors. Understanding its lessons is not merely an academic endeavor; it’s an essential part of navigating the complexities of modern society. The usefulness of these lessons extends beyond the confines of research and tech labs, affecting our daily lives in ways we may not always recognize. When we talk about the importance of AI lessons, we refer to their significance in shaping policy, guiding ethical practices, and offering frameworks for future innovations.
Artificial intelligence is vastly more than algorithms and data processing. It encapsulates a harvest of experiences, concepts, and teachings that drive both its development and application. Notably, it also opens discussions about societal implications—ranging from its potential to enhance efficiency to its capacity for disruption. For example, an understanding of AI’s impacts on employment structure can help communities prepare better strategies for workforce adaptation.
Understanding Artificial Intelligence
At its core, AI involves creating systems capable of performing tasks that typically require human intelligence. This includes recognizing speech, making decisions, or interpreting images. The challenges come from not only developing these systems but also ensuring they operate ethically and effectively. A solid grasp of AI entails knowing how algorithms function, the data required for machine learning, and the potential biases embedded therein.
- AI methods often harness vast datasets, yet the quality of these datasets can substantially influence the outcomes. When datasets reflect historical biases, for instance, the AI model might perpetuate those biases, thereby impacting outcomes adversely.
- Machine learning, a significant subset of AI, focuses on enabling computers to learn from data. However, this learning isn't always linear—sometimes, the path can be rife with unexpected consequences.
By gaining a deeper understanding of artificial intelligence, stakeholders can engage in meaningful dialogues about its application and limitations. This comprehension paves the way for better decision-making and ensures that AI serves humanity's best interests rather than merely automating tasks.
The Importance of AI Education
With the fast-paced evolution of artificial intelligence, the need for robust education in this field cannot be overstated. An informed populace is critical to responsibly harnessing the benefits of AI while mitigating risks. Education empowers individuals to comprehend not just the technology itself but also its societal ramifications.
- Curriculum reforms that integrate AI at various educational levels can nurture a generation that is adept in critical thinking and problem-solving, with the ability to innovate.
- Professional training and resources aimed at industry-specific applications helps professionals to utilize AI effectively, understanding its tools and limitations thoroughly.
The integration of AI literacy programs across various disciplines ensures that emerging technologies are not seen as mere tools but as integral components of our future decision-making processes. Since the implications of AI spread widely across societal and ethical spectrums, it's essential that education remains at the forefront, allowing people to contribute positively to the discourse surrounding AI deployment.
"Education is the most powerful weapon which you can use to change the world." – Nelson Mandela. True in the context of AI, this mentality encourages proactive engagement with the field.
By delving into these key lessons about artificial intelligence, we not only embrace the technology itself but also prepare to engage with its various complexities thoughtfully.
Core Concepts of AI
Understanding the core concepts of artificial intelligence is crucial for anyone looking to navigate this complex landscape. It lays the foundation for grasping how AI works, its capabilities, and its limitations. When you dive into AI without a solid understanding of these concepts, it can feel like trying to catch smoke with your bare hands. This section serves as a bridge for students, researchers, educators, and professionals to understand the key building blocks that make up the field.
Machine Learning Fundamentals
Machine learning is often at the heart of modern AI systems. Unlike traditional programming, where a developer writes explicit instructions, machine learning allows systems to learn from data. This is crucial because it makes AI adaptable and capable of improving with experience.
There are several types of machine learning:
- Supervised Learning: The model is trained on labeled data, meaning the outcomes are already known. For instance, if you feed a model a bunch of photos of cats and dogs with labels, it learns to differentiate between the two.
- Unsupervised Learning: This involves algorithms identifying patterns in unlabeled data. Think of it as giving a child a puzzle with no picture and letting them figure it out on their own.
- Reinforcement Learning: In this approach, an agent learns to make decisions by receiving rewards or penalties. It's akin to training a dog—the more treats it gets for sitting, the better it becomes at that task.
Understanding these fundamentals allows one to appreciate the challenges involved in training models and the various data requirements.
Natural Language Processing Insights
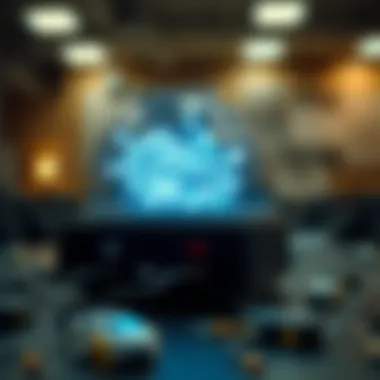
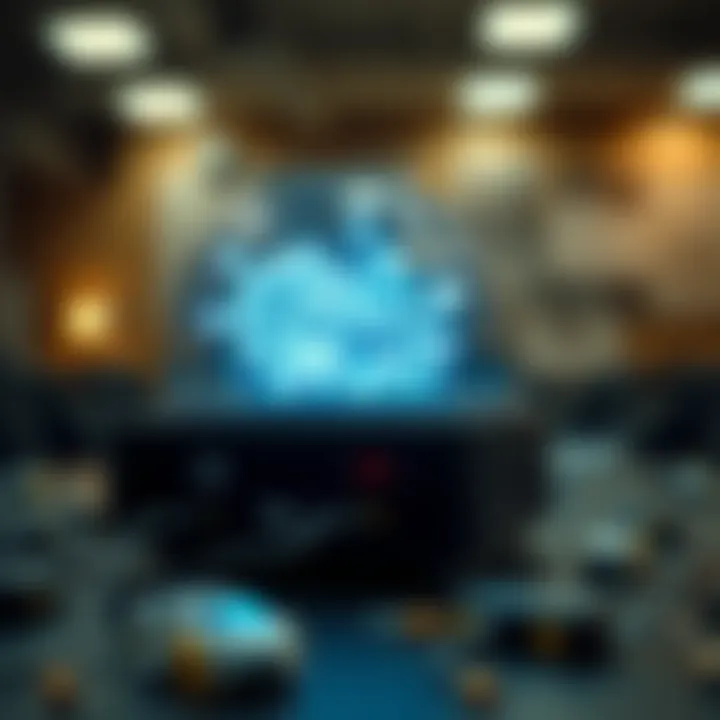
Natural language processing (NLP) bridges the gap between human communication and computer understanding. It empowers machines to read, interpret, and even respond to human language in ways that are meaningful.
NLP applications range from chatbots that assist customer service to tools that translate languages. Yet, there are inherent complications, such as:
- Ambiguity in Language: Words often have multiple meanings. For example, "bank" can denote a financial institution or the side of a river.
- Cultural Context: Different languages and dialects may use expressions that don't translate effectively, making it difficult for an AI to adapt across various regions.
This layer of complexity makes NLP an incredibly fascinating field, opening doors to new ways of human-computer interaction.
Ethics in AI Development
Ethics should be at the forefront of any AI initiative. As AI systems become more pervasive, the potential for biased outputs, invasion of privacy, or even autonomous decision-making raises significant concerns. The notion of "what is fair?" in AI is not simply a black-and-white question.
Some critical considerations include:
- Bias in Algorithms: When training data reflects historical inequalities or stereotypes, AI systems may perpetuate these biases. This can have dire consequences in fields like hiring or law enforcement.
- Accountability: Who is responsible if an AI system makes a mistake? This question becomes particularly tricky when self-driving cars or drones are involved.
- Privacy: AI often requires vast amounts of data. How this data is collected, stored, and used raises critical ethical questions that cannot be overlooked.
Addressing these ethical dilemmas in AI development is crucial for ensuring that advancements benefit society as a whole, rather than creating further divides. It's essential that as we move forward, we remain vigilant about the impact of what we create.
Understanding core concepts in AI is not just about knowledge for knowledge’s sake; it's a journey that has real-world implications for all our lives.
Pedagogical Approaches to AI Learning
The methods we use to teach AI can shape not just how students understand the technology, but also how they’ll apply it in the real world. Pedagogical approaches in AI learning should be diverse and adaptable to accommodate various learning styles and objectives. In doing so, these methods foster not only knowledge acquisition but also critical thinking and problem-solving skills, which are essential in the rapidly changing landscape of AI technology.
One significant aspect to consider is the integration of theoretical and practical knowledge. Understanding algorithms is vital, yes, but without the ability to implement them, the knowledge remains abstract. By exploring real-world problems through AI, learners can relate complex concepts to tangible outcomes. This connection amplifies engagement and retention, making for a win-win situation.
Moreover, there exists a robust need for educators to stay updated on the latest advancements in AI technologies. As new tools and methodologies emerge, the educational content must evolve. This adaptability ensures that students receive relevant, practical knowledge that equips them for their future careers in a tech-dominated world.
Curriculum Development in AI Education
Creating a curriculum for AI education is a bit like planting a garden; it requires proper planning, the right resources, and the understanding that growth takes time. A well-structured curriculum must blend foundational principles with the latest technological advancements.
- Foundation Building: Students should begin with the basics, covering topics like data structures, algorithms, and programming languages such as Python or R. It’s critical to ensure that learners are comfortable with these concepts before they dive into more complex AI topics like machine learning and deep learning.
- Hands-On Experience: Classroom learning should be complemented with hands-on experiences. This might include workshops, coding exercises, and exposure to AI tools such as TensorFlow or PyTorch. This approach not only solidifies knowledge but also boosts confidence in applying it.
- Cross-Disciplinary Modules: AI rarely exists in a vacuum. Integrating modules that focus on ethics, societal impacts, and domain-specific applications of AI (like healthcare or finance) will prepare students for the multidisciplinary nature of real-world AI applications.
- Regular Assessment and Feedback: Developing effective assessments to gauge student understanding is paramount. These assessments should promote critical thinking and problem-solving rather than mere rote memorization. Regular feedback loops ensure that educators can adjust the curriculum according to students' needs and industry trends.
Project-Based Learning in AI
Project-based learning stands out as an incredible approach to AI education. Through projects, students can explore their own interests while learning. This form of learning not only makes theory more digestible but also fosters collaboration among peers.
When students engage in hands-on projects, several benefits emerge:
- Real-World Application: Students tackle real-life problems, applying what they’ve learned to create solutions. The satisfaction that follows crafting a working model or application can bolster their motivation.
- Collaboration Skills: Most AI projects require teamwork. Students learn to communicate, delegate tasks, and leverage each other's strengths, which mirrors the collaborative nature of most tech environments today.
- Innovation and Creativity: With the freedom to explore various aspects of AI, students can innovate and think outside the box. This can lead to unique solutions or insights that standard learning might not foster.
- Portfolio Development: As they embark on projects, students build a portfolio that showcases their skills and accomplishments, which is incredibly valuable when entering the job market.
in summation, pedagogical approaches to AI learning are crucial for nurturing a generation of thinkers and doers in the realm of technology. Balancing curriculum development with project-based experience helps cultivate a learning environment that is both engaging and effective, equipping students with the necessary skills to tackle future challenges in artificial intelligence.
Interdisciplinary Perspectives in AI
In today’s complex world, the application of artificial intelligence encompasses a vast array of domains, each with its own set of unique challenges and opportunities. The interdisciplinary perspectives in AI serve as a critical framework that guides us to understand how this technology can be harnessed to benefit various fields beyond just technology and engineering. Integrating knowledge from sociology, psychology, ethics, law, and more gives us a more holistic viewpoint of AI's impact and potential.
Collaborative Learning Across Disciplines
Collaboration between distinct academic and professional fields is the lifeblood that fuels innovation in AI. When experts from various disciplines converge, they bring different lenses through which to view problems and solutions. For instance, blending computer science knowledge with insights from behavioral psychology can lead to the development of more user-friendly AI systems that account for human nuances in decision-making. This cross-pollination of ideas is not merely beneficial but essential in creating AI applications that are both effective and ethical.
- The interplay of disciplines promotes:
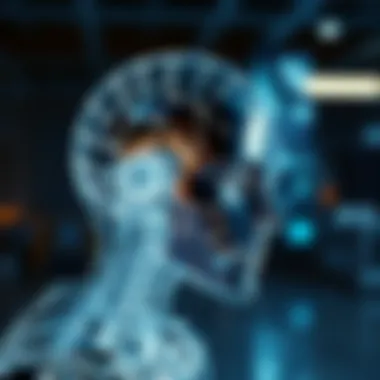
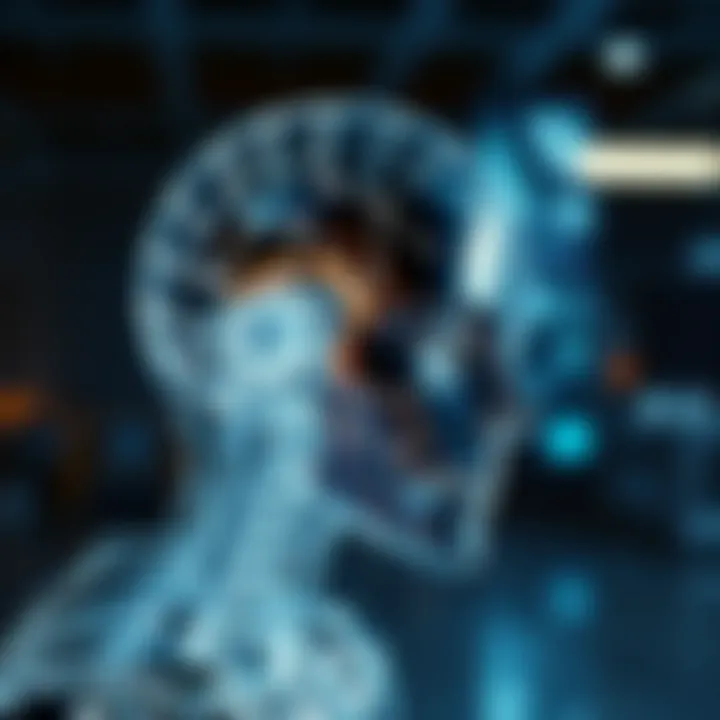
- Diverse problem-solving strategies
- Comprehensive risk assessments
- Multi-faceted evaluations of outcomes
In practice, collaborative learning can take shape in many forms. Project-based approaches, hackathons, or interdisciplinary workshops where participants share their specialized knowledge can enhance understanding and co-creation. Dedicating spaces for dialogue among fields provides fertile ground for unique solutions that might not emerge within silos.
"Bringing diverse minds together can spark the ideas that lead to truly transformative solutions in AI."
Integrating AI into Non-Technical Fields
While AI is often associated with chipsets and coding, its influence permeates various non-technical areas as well. The challenge lies in articulating how AI can not only supplement but also enhance these fields. For example, in healthcare, AI can assist doctors with diagnosing diseases through predictive analytics, not as a replacement but as a powerful assistant human can leverage. In the realm of education, teachers can use AI-driven tools for personalized learning experiences tailored to each student’s unique needs.
- Potential sectors for AI integration:
- Arts and Humanities: Automated tools aiding in curation and analysis of art pieces can reshape how we engage with culture.
- Law and Governance: AI can support legal research, simplifying complex data processes that influence policy-making decisions.
- Social Sciences: This field can use AI for analyzing large sets of social data, helping to uncover trends in public behavior and opinion.
However, the key to successfully integrating AI into non-technical fields depends on understanding the specific requirements and ethical considerations of those domains. Practitioners need to engage with technologists carefully to ensure that AI applications align with humanistic values, providing real benefits without overshadowing the essential human elements.
In summary, recognizing and embracing the interdisciplinary perspectives in AI isn’t just an academic exercise—it’s a call to action. Blending varied fields leads to innovative practices that can elevate the way we work with AI while ensuring ethical considerations remain at the forefront.
Practical Applications of AI Lessons
Understanding the practical applications of artificial intelligence offers profound insights into how AI is shaping various sectors today. These applications provide tangible benefits, streamlining processes, enhancing decision-making, and improving outcomes across industries. Knowledge of these uses plays a pivotal role in grasping the true potential of AI, not only reflecting its capabilities but also its challenges and the ethical considerations that accompany such integration. By examining practical applications, one can appreciate the multifaceted nature of AI and its relevance in real-world scenarios.
AI in Healthcare: Transformative Outcomes
The integration of AI in the healthcare sector has sparked a transformative wave that’s changing how practitioners approach patient care. From predictive analytics that pinpoint potential health crises to personalized medicine that tailors treatments to individual patients, the role of AI is becoming indispensable.
- Patient Monitoring: AI applications can track patient vitals in real-time, providing alerts that can prevent emergency situations.
- Disease Diagnosis: Machine learning algorithms analyze medical images with an accuracy that rivals human specialists, sometimes identifying anomalies that even experienced radiologists may miss.
- Drug Discovery: AI speeds up drug development by predicting outcomes of pharmaceutical trials, cutting down the timeline from years to mere months.
Moreover, the ethical concerns surrounding data privacy and algorithmic bias are paramount. Ensuring transparency in AI decision-making processes can reinforce trust in these technologies while mitigating risks.
"AI in healthcare not only augments human capacities but also raises the bar for what is possible in medicine.”
AI in Financial Services
The financial services industry has seen a significant shift with the introduction of AI technologies. As financial markets become more complex, AI tools are utilized to enhance the management of risks, streamline operations, and improve customer engagement.
- Fraud Detection: AI systems analyze transaction patterns to flag unusual activities, significantly enhancing security measures.
- Investment Analysis: Algorithms can assess vast amounts of data to forecast market trends, helping financial professionals make better-informed decisions.
- Customer Support: Chatbots and virtual assistants are becoming common, providing 24/7 support and handling a multitude of inquiries efficiently.
However, reliance on algorithms for financial predictions presents challenges. It's vital to maintain an ethical framework that ensures fairness, transparency, and accountability in all AI financial applications.
AI in Education: Enhancing Learning Experiences
Artificial intelligence has opened new doors in the realm of education. By personalizing learning experiences, AI addresses diverse student needs, fostering an environment where everyone can thrive.
- Personalized Learning Paths: AI systems can analyze a student’s learning habits and adapt curriculum to suit their strengths and weaknesses.
- Administrative Efficiency: AI automates routine tasks such as grading and attendance tracking, allowing educators to focus more on teaching.
- Accessibility: For students with disabilities, AI tools offer unprecedented levels of accessibility, ensuring equal opportunities in learning.
While these applications provide commendable benefits, educators must remain vigilant about the ethical implications of data collection and student privacy. It's crucial to harness AI responsibly, ensuring that advancements in education do not compromise student rights and dignity.
AI Ethics and Governance
The landscape of artificial intelligence is not just about algorithms and data; it's deeply intertwined with ethical considerations and governance. As AI continues to evolve, it raises crucial questions about how it interacts with society. Ethical governance serves as the backbone to ensure that AI contributes positively, aligning technological innovation with societal values. This section delves into the significance of ethics and regulations as crucial elements in the development and implementation of AI systems.
The Role of Regulations in AI Development
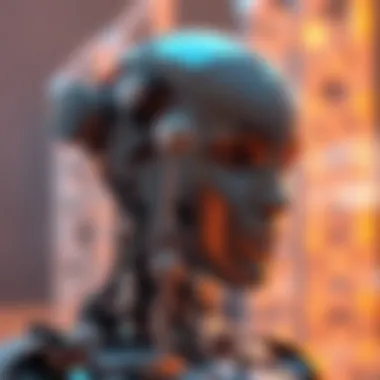
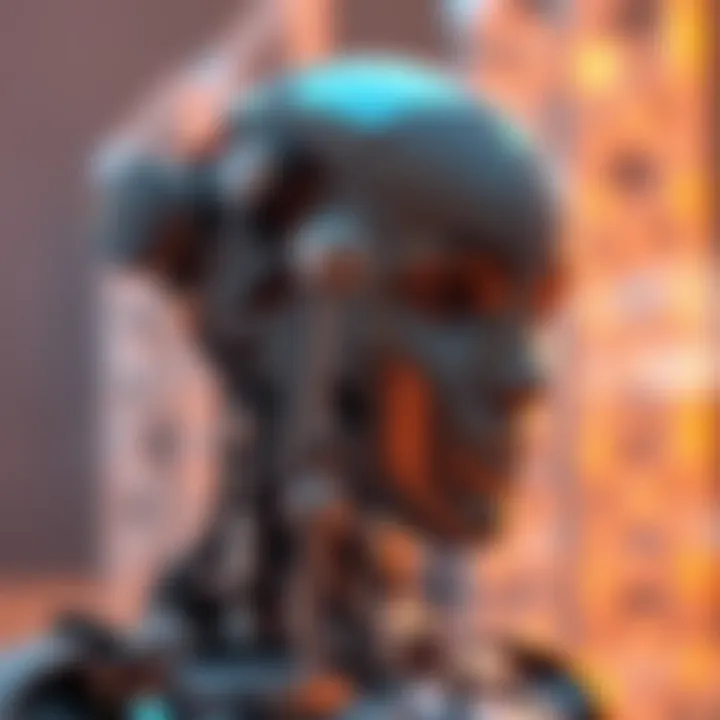
In a world rapidly adopting AI technologies, regulations are vital. They act as a safety net, ensuring the responsible development of AI systems. Without these regulations, we risk creating a Wild West scenario where anything goes, potentially leading to disastrous consequences. Here are some key aspects of regulations in AI:
- Ensuring Transparency: Regulations demand that AI developers maintain transparency about how systems function. This transparency helps users understand AI decisions, especially in critical areas like healthcare or law enforcement.
- Boosting Accountability: Laws can hold companies responsible for the outcomes of their AI systems. This accountability mandates that organizations prioritize ethical considerations, fostering a culture of responsibility in AI development.
- Promoting Safety Standards: Regulations can outline specific safety standards that AI technologies must meet. This inclusion not only protects consumers but also assures developers of stability and trust as they innovate.
However, implementing effective regulations is no walk in the park. There's a balance to strike, as overly stringent regulations can stifle innovation, while too lax regulations can lead to unethical practices. Finding that middle ground is where the challenge lies.
"Regulations act as a compass in the ever-changing landscape of artificial intelligence, guiding developers toward ethical decisions."
Ethical Frameworks for AI Implementation
Alongside regulations, implementing sound ethical frameworks is crucial in guiding the development of responsible AI technologies. These frameworks provide the moral ground upon which AI systems are built. Here are some prominent principles often included in ethical frameworks:
- Fairness: AI systems must be designed to be fair. This means avoiding biases that can lead to discrimination against certain groups. Fairness should be at the forefront of AI design, requiring constant assessment.
- Privacy Protection: The ethical handling of data is paramount. AI systems should respect individuals' privacy, ensuring that data usage aligns with consent and protection laws.
- Beneficence: This principle insists that AI should aim to do good. Developers must consider the broader impact of their technologies, ensuring that AI serves humanity rather than posing threats.
These ethical guidelines not only shape how AI is designed but also influence how it’s received by society. The interplay between ethics and regulations forms a dynamic partnership that can elevate AI to serve the common good rather than merely the interests of a few.
Future Perspectives on AI Education
As we look ahead into the rapidly evolving landscape of artificial intelligence, it's crucial to understand how education must adapt to keep pace with these changes. The field of AI is not static; it’s a moving target that demands continual learning and adaptability from both students and educators. Incorporating future perspectives into AI education can lead to significant benefits, including enhanced relevance of academic programs, better preparation for the workforce, and fostering a culture of lifelong learning.
Adapting Curriculum for Emerging AI Technologies
To keep up with the fast-paced developments in AI technologies, educational curriculums must be dynamic and adaptive. It's no longer enough to stick to a one-size-fits-all approach. Here are some considerations for adapting educational curriculums:
- Integration of New Technologies: Emerging technologies, such as federated learning and quantum computing, should be integrated into current curriculums. It is important that students are not only introduced to traditional machine learning concepts but also exposed to the new tools and frameworks shaping the future.
- Interdisciplinary Approach: AI is not confined to computer science alone. Fields like psychology, ethics, and even arts contribute significantly to its application. Curriculums should, therefore, promote interdisciplinary learning, enabling students to draw connections between AI and other fields, enriching their understanding and sparking innovation.
- Hands-on Experiences: Theoretical knowledge is vital, but without practical application, students might struggle to transfer their knowledge into real-world scenarios. Incorporating project-based learning, internships, and collaborations with tech companies can provide invaluable experience.
Adapting curriculum in this way not only prepares students for current industry demands but also cultivates a mindset geared towards innovation and problem-solving.
The Impact of Lifelong Learning in AI
The nature of AI is such that learning cannot stop after formal education. Lifelong learning emerges as a critical component in keeping skills fresh and relevant. Here's why:
- Rapid Technological Changes: AI technologies are continuously evolving. What was cutting-edge yesterday could become obsolete today. Professionals must commit to ongoing education through workshops, online courses, and conferences to stay current.
- Diverse Learning Opportunities: The rise of online learning platforms has democratized access to education. Professionals can now learn at their own pace and choose subjects that directly relate to their career paths or personal interests.
- Enhancing Skills for Future Challenges: Continuous education allows individuals to develop new skills, adapt to unexpected challenges, and remain competitive in a job market that increasingly values adaptability and ongoing professional development.
Lifelong learning is the bridge that connects educational outcomes with the demands of the ever-changing AI landscape.
Concluding Thoughts on AI Lessons
The insights drawn from the world of artificial intelligence highlight not just its capabilities but also the profound impacts it can wield on various facets of society. This article expounds upon pivotal lessons that surface through diverse educational frameworks, ethical considerations, and real-world applications. Each section unravels a thread of AI’s complexity, weaving together a narrative that transcends simple technology integration.
Reflections on the Journey of Learning AI
Reflecting on the journey through the realm of AI, it becomes evident that each step forward is more than just an accumulation of knowledge; it's about fostering a mindset attuned to innovation and curiosity. Understanding AI isn't merely about grasping algorithms or coding languages; it's about looking deeper into the principles that govern intelligent systems.
Learning AI requires a combination of theoretical grounding and practical applications. Educators and learners alike need to embrace a symbiotic relationship that encourages exploration and critical thinking. Gaining insights from machine learning or natural language processing should provoke questions about their societal implications, driving a deeper comprehension of both the power and the pitfalls of these technologies.
In addition, the unique interdisciplinary perspectives alluded to throughout this article play a pivotal role in shaping one's approach to AI. An engineer, for example, may look at algorithm efficiency, while a sociologist might dissect the cultural ramifications of AI's implementation. It's the convergence of these viewpoints that enriches the educational tapestry surrounding artificial intelligence.
“Knowledge is only as good as the understanding that comes with it.”
The Role of Continuous Adaptation in AI Education
In the amorphous landscape of technology, the necessity for continuous adaptation in AI education cannot be overstated. As new advancements unfold with incredible speed, the demand for learners to keep pace with these changes intensifies. This leads to a scenario where static curricula become obsolete, potentially leaving students ill-prepared for the realities of the job market.
Hence, incorporating elements of lifelong learning into AI education is essential. This involves:
- Regular curriculum updates: Educators should consistently revise course materials to reflect the latest trends and technologies in AI.
- Flexible learning paths: Students should have the option to pursue specialized tracks within AI that cater to individual interests and career goals.
- Industry collaboration: Partnerships with tech companies can help academic institutions understand future hiring needs, ensuring that the workforce is adequately prepared.
To foster a culture of adaptability, educators must also emphasize meta-cognitive skills, which enable learners to evaluate their own understanding and knowledge gaps. Developing the ability to critically assess where one stands in the grand scope of AI developments turns education into a dynamic, continuous cycle of growth and discovery.