Understanding Predictive Analysis in Scientific Research
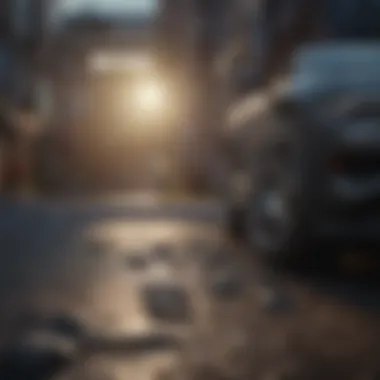
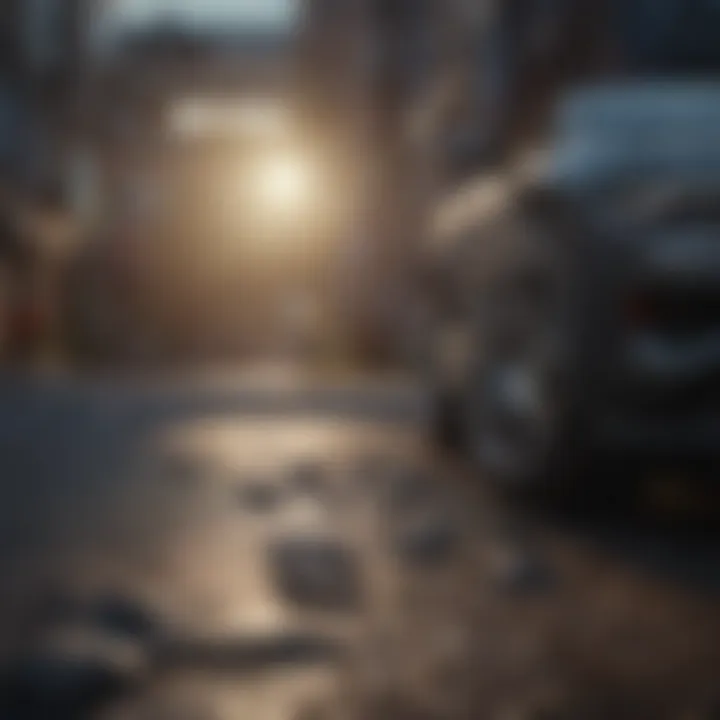
Intro
Predictive analysis stands as an important framework within scientific research, facilitating the extraction of trends and the forecasting of outcomes across numerous disciplines. Its application ranges from predicting disease outbreaks in biology to estimating chemical reactions in chemistry, and even modeling climate change in earth sciences. By integrating statistical techniques and machine learning algorithms, researchers are equipped with the tools needed to analyze complex datasets and make informed decisions.
Understanding predictive analysis is crucial for students, researchers, and professionals alike. As the volume of scientific data continues to grow exponentially, the ability to predict future results becomes a significant asset. This article will delve into the various dimensions of predictive analysis, exploring its methodologies, significance, and the contributions it brings to scientific advancement.
Research Highlights
Overview of Key Findings
Predictive analysis has shown remarkable ability in varied domains. In biology, predictive models can help uncover patterns in genomics, enhancing our understanding of diseases and improving treatment protocols. In chemistry, advanced algorithms enable the prediction of molecular interactions and reaction outcomes, thus speeding up drug design processes and material science innovations. Physics utilizes predictive modeling to simulate complex systems, allowing for the anticipation of phenomena from particle collisions to cosmological events. Additionally, in earth sciences, predictive analysis is vital in modeling climate change impacts, thus informing environmental policies.
Significance of the Research
The relevance of predictive analysis cannot be overstated. It has the potential to guide research agendas, influence policy decisions, and foster innovation across scientific fields. By employing data-driven insights, researchers can prioritize areas that require immediate attention, allocate resources effectively, and derive meaningful conclusions based on solid empirical evidence.
"Predictive analysis empowers scientists to move beyond mere observation, facilitating a proactive approach to research."
Emphasizing its significance, the integration of predictive techniques in scientific research enhances not only the understanding of complex systems but also the capability to explore previously uncharted territories.
Original Research Articles
Summary of the Article
The article seeks to provide a comprehensive overview of predictive analysis in scientific research. It synthesizes various methodologies, discusses their applications, and explores the societal implications generated by predictive modeling in different scientific domains.
Author Contributions
The researchers contributing to this domain bring diverse expertise. Their backgrounds in statistics, data science, and specific scientific fields create a multidisciplinary approach, ensuring a robust exploration of predictive techniques. Their work not only advances academic knowledge but also provides practical applications that benefit society as a whole.
Prolusion to Predictive Analysis
Predictive analysis has emerged as a crucial tool in scientific research, allowing scholars and professionals to forecast outcomes based on historical data. Its significance lies in the ability to make informed decisions, streamline processes, and enhance understanding across various scientific domains. This section will explore the foundational concept of predictive analysis and its relevance within the broader context of scientific inquiry.
Definition and Importance
Predictive analysis is defined as the practice of using statistical algorithms and machine learning techniques to identify the likelihood of future outcomes based on historical data. Its importance cannot be understated. By transforming raw data into actionable insights, predictive analysis provides a framework for anticipating trends, making data-driven decisions, and optimizing research efforts.
In fields such as biology and environmental science, predictive analysis enables researchers to model complex systems. This assists in forecasting disease outbreaks, ecological shifts, and climate change effects. The predictive capabilities allow for preemptive actions, ultimately supporting sustainability and public health initiatives.
Furthermore, it plays a vital role in chemical research, facilitating reaction predictions and material property evaluations. This predictive power substantially reduces experimental costs and time, thus accelerating innovation.
"Predictive analysis empowers researchers to foresee possibilities and mitigate uncertainties, enhancing scientific inquiry."
The integration of predictive analysis is not merely about employing data; it also represents a paradigm shift in how researchers approach problems. It prompts a deeper understanding of variables at play, leading to more robust hypotheses and experiments.
Historical Context
The development of predictive analysis can be traced back to the early 20th century, emerging from advances in statistics and computing. Early examples include the use of regression analysis to identify trends in biological data and epidemiological modeling to predict disease spread.
As the field of data science evolved, so too did predictive techniques. With the rise of computational power and accessibility to large datasets, predictive analysis experienced a significant enhancement during the late 20th and early 21st centuries. Technologies like machine learning and artificial intelligence have further propelled its applications, fostering interdisciplinary collaborations across fields.
In summary, the historical trajectory of predictive analysis illustrates its growing importance in scientific inquiry. As methodologies improve and data availability expands, the impact of predictive analysis is set to rise, providing unprecedented insights and fostering innovation across diverse scientific realms.
Foundational Concepts of Predictive Modeling
Predictive modeling is a crucial aspect of predictive analysis, serving as the backbone for applications in various scientific fields. Understanding foundational concepts is essential for anyone looking to harness the power of data and modeling techniques effectively. These concepts lay the groundwork, facilitate meaningful insights, and enable informed decision-making. By grasping these elements, researchers can optimize their methodologies, ensuring reliable results and powerful predictions.
Data Collection and Preparation
Data collection forms the first step of any predictive modeling process. It involves gathering relevant data from varied sources such as laboratory experiments, surveys, or public databases. The quality of predictions depends significantly on the data collected. Poor-quality data can lead to misleading outcomes.
Preparation of data is the next step, which includes several sub-processes:
- Cleaning: Removing irrelevant or incomplete records.
- Transformation: Adjusting data formats or modifying data types to ensure consistency.
- Normalization: Scaling the data to a common range without distorting differences.
Proper data collection and preparation are vital because they directly affect the accuracy and reliability of predictive models. A well-prepared dataset serves as a strong foundation for any subsequent analysis.
Statistical Techniques Used
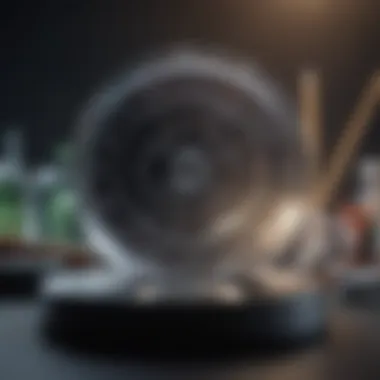
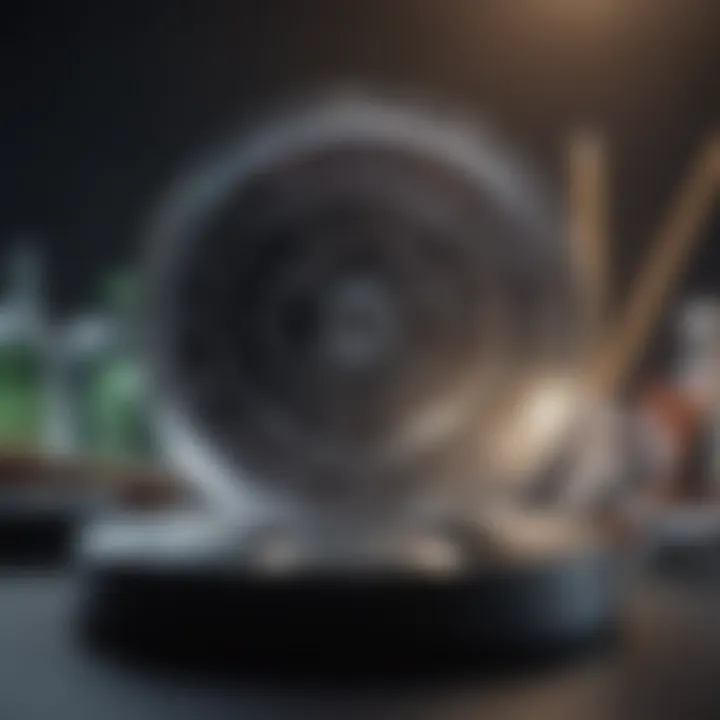
Statistical techniques play a significant role in predictive modeling. They help in analyzing relationships and patterns within data. Common statistical methods include:
- Regression Analysis: This technique is used to model the relationship between a dependent variable and one or more independent variables. Examples are linear regression and logistic regression.
- Time Series Analysis: Useful for modeling data that is collected over time, it helps in forecasting future values based on past trends.
- Bayesian Analysis: This method incorporates prior knowledge along with current data to refine predictions.
These techniques provide a robust framework. They allow researchers to validate predictive models and assess the uncertainty inherent in estimations. Understanding the statistical underpinnings enhances the ability to create models that can generalize beyond just the data used for training.
Machine Learning Approaches
The evolution of predictive modeling has been greatly influenced by machine learning. Machine learning approaches automate the modeling process, allowing for complex patterns to be discovered that may be missed by traditional statistical methods. Some popular machine learning approaches in predictive analysis are:
- Supervised Learning: This includes techniques like decision trees, support vector machines, and neural networks. It requires labeled data for training, which means the outcome variable is known.
- Unsupervised Learning: Techniques such as clustering (e.g., K-means) and association rules help uncover hidden patterns within data without prior labels.
- Reinforcement Learning: This approach focuses on how agents ought to take actions in an environment to maximize cumulative rewards.
Machine learning approaches increase the modeling capability. They are especially useful in dealing with large and complex datasets, making them invaluable in fields ranging from biology to earth sciences.
The integration of advanced statistical techniques and machine learning approaches equips researchers with tools to yield better insights from data. Understanding these foundational concepts is essential in leveraging predictive analysis for scientific advancement.
Applications in Biology
In the realm of scientific research, predictive analysis serves as a pivotal tool, particularly in biology. This section explores its various applications, focusing on how predictive models enhance our understanding and management of biological systems. They offer crucial insights that help in decision-making in health care and environmental conservation. Therefore, understanding the applications of predictive analysis in biology is fundamental for students, researchers, and professionals alike.
Predicting Disease Outcomes
Predictive models in the context of disease outcomes are increasingly important. These models analyze extensive data from various health records, genetic information, and environmental factors to forecast disease progression. This can greatly aid clinicians in devising personalized treatment plans.
- Key Components: A variety of factors are considered, including:
- Benefits: The application of predictive analysis in medicine allows for:
- Patient demographics
- Genetic predispositions
- Historical treatment responses
- Lifestyle factors
- Early detection of diseases, such as cancer or diabetes.
- More effective resource allocation in healthcare facilities.
- Enhanced understanding of disease mechanisms leading to innovative treatment approaches.
The success of these predictive models primarily depends on the quality of the data collected and the methodologies applied. Inaccurate or biased data can lead to wrong conclusions, potentially affecting patient outcomes negatively. Hence, continuous improvement of data quality stands as a significant consideration in predictive modeling.
"Predictive modeling holds the potential to redefine how we approach patient care, making it more proactive rather than reactive."
Ecological Modeling
Another critical area of predictive analysis is ecological modeling. This involves the use of models to predict interactions between organisms and their environment. It assists in understanding biodiversity changes and habitat loss, critical concerns in today's world.
- Key Areas Covered: In ecological modeling, scientists examine:
- Benefits: Employing predictive analysis in ecology offers various advantages:
- Species distribution and their responses to climate change.
- The impact of invasive species on native habitats.
- The outcomes of conservation efforts.
- Enables effective management of endangered species.
- Supports restoration efforts by identifying key habitats.
- Facilitates policy-making decisions for environmental conservation.
By employing predictive analysis, ecologists can simulate future scenarios, enabling proactive strategies to combat environmental issues. Failure to utilize these tools could lead to mismanagement of natural resources and further ecological degradation.
By integrating predictive analysis into both disease and ecological modeling, scientists can draw more accurate insights, allowing for better decision-making and resource management in the face of numerous challenges.
Applications in Chemistry
The applications of predictive analysis in chemistry hold significant importance in advancing the discipline. As chemical research continues to grow more complex, the ability to predict outcomes can save time and resources. Through predictive modeling, chemists can hypothesize reaction pathways and forecast material properties before conducting experimental work. This preemptive approach not only enhances efficiency but also reduces the environmental impact associated with wasteful trial-and-error methods.
Predictive analysis in chemistry allows for the evaluation of a vast array of data, including molecular structures and reaction conditions. By leveraging datasets, researchers can uncover patterns that facilitate the optimization of chemical processes. This further enhances the reliability and accuracy of predictions, which benefits various fields, from drug development to materials science.
Reaction Prediction
Reaction prediction involves forecasting the products of chemical reactions based on specific reactants and conditions. This process is vital for chemists, as it allows for targeted experimentation and resource management. Advanced predictive models apply algorithms to predict the likelihood of certain reactants yielding desired products, considering factors such as temperature, pressure, and catalysts.
Several approaches can be utilized for reaction prediction:
- Machine Learning: Algorithms like neural networks analyze historical reaction data to uncover patterns, enabling accurate predictions of outcomes.
- Quantum Chemistry: This method uses quantum mechanical principles to simulate chemical reactions, offering insights into molecular behavior at a fundamental level.
- Knowledge-Based Systems: These systems compile expert knowledge and databases of reactions to generate predictions based on established chemistry rules.
Through these methodologies, chemists can better plan their experiments, reducing time spent on unsuccessful reactions.
Material Property Forecasting
Material property forecasting refers to the ability to predict the characteristics of new or existing materials. This application of predictive analysis is crucial in developing alloys, polymers, and other composite materials that meet specific requirements. Understanding properties like strength, conductivity, and reactivity before synthesis saves researchers substantial resources.
The techniques employed in material property forecasting include:
- Computational Modeling: Simulations are performed to estimate properties based on chemical structure and composition, guiding the design of new materials.
- Data Mining: A wealth of data on existing materials is sorted and analyzed to predict new property trends, facilitating innovation.
- Cross-disciplinary Approaches: Collaborations between chemists, physicists, and engineers create a holistic understanding of material behavior under various conditions.
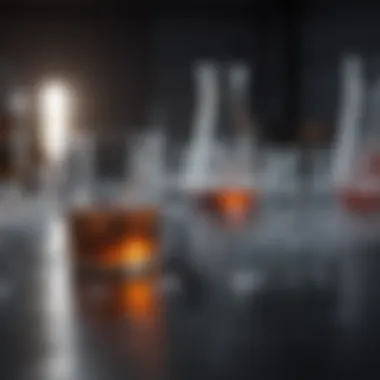
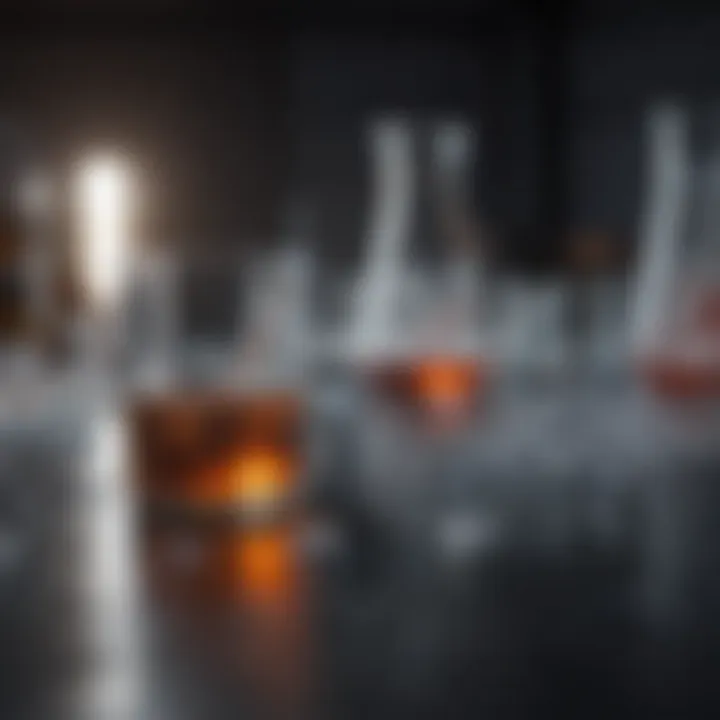
Efforts in this area have far-reaching implications across industries including construction, electronics, and nanotechnology, fostering sustainable developments.
Predictive analysis in chemistry not only streamlines research but also propels innovation forward, making it an indispensable tool for contemporary chemists.
Applications in Physics
Predictive analysis plays an increasingly vital role in the field of physics. By leveraging data-driven models, physicists can forecast complex phenomena and refine theoretical frameworks. This application not only hastens research progress but also enhances experimentation designs, making it a cornerstone for advancements in the discipline.
Predictive Simulations
Predictive simulations are indispensable in modern physics. They allow researchers to model scenarios that are either impractical or impossible to recreate in a laboratory setting. For example, simulations can depict high-energy particle collisions, helping scientists understand fundamental forces and particles at play in the universe.
These simulations utilize algorithms that can analyze a vast amount of variables, generating results that can influence real-world physics. Notably, complex physical systems like plasma or fluid dynamics can be modeled accurately. Furthermore, advancements in computational power have led to more refined simulations with higher detail, enriching our understanding of theoretical constructs.
Some key benefits of predictive simulations include:
- Ability to visualize processes in real-time.
- Reduction in experimental costs and time.
- Enhanced ability to test hypotheses and validate theories.
- The opportunity to explore previously unexamined physical phenomena.
"Simulations must become a more integrated part of scientific research to advance our quest for knowledge in physics."
Quantum Computing Predictions
Quantum computing predictions represent another frontier in predictive analysis within physics. With quantum computers, scientists can solve equations that classical computers may find impossible or too slow to compute. This capability can lead to breakthroughs in quantum mechanics and materials science.
The potential applications are vast. For instance, predicting the behavior of electrons in a material could lead to the development of new materials with unique properties. Additionally, quantum algorithms can process large datasets efficiently, enabling predictions that were not feasible before.
Key considerations for the application of quantum computing in predictive analysis include:
- Data Complexity: Quantum systems often produce vast amounts of data that require careful management and interpretation.
- Error Rates: Quantum computing is still subject to high error rates, which can affect the reliability of predictions.
- Interdisciplinary Collaboration: Effective use of quantum computing for predictions will necessitate collaboration between physicists, computer scientists, and data analysts.
By focusing on predictive simulations and quantum computing, the physics community can harness the full power of predictive analysis, facilitating deeper insights into the fabric of reality and the universe's underlying principles.
Applications in Earth Sciences
Predictive analysis plays a crucial role in earth sciences. By utilizing various data and modeling techniques, researchers can forecast significant events and better understand complex earth systems. Understanding these applications is essential for developing adaptive strategies against climate change, managing natural resources, and preparing for natural disasters. The insights derived from predictive modeling can greatly influence policy decisions and public safety, highlighting its importance.
Climate Modeling
Climate modeling is a fundamental aspect of predictive analysis in earth sciences. Climate models simulate the interactions between the atmosphere, oceans, land surface, and ice, providing essential information about climate dynamics. These models help in understanding how various factors, such as greenhouse gases, influence climate change over time.
By analyzing historical data and ongoing observations, climate models allow scientists to predict future climate scenarios. Effective climate modeling leads to informed decisions regarding environmental policies and mitigation strategies. It aids governments and organizations in preparing for potential climate impacts, such as rising sea levels and changing weather patterns.
Some critical benefits of climate modeling include:
- Improved Forecasting: Advanced models can predict extreme weather events with increased accuracy, enhancing preparedness.
- Resource Management: Insights from climate models can guide sustainable resource allocation and disaster response strategies.
- Policy Development: Predictive analyses form the basis for effective policy-making concerning climate change.
"Climate models provide invaluable foresight, enabling societies to prepare for impending changes in our environment."
Natural Disaster Forecasting
Natural disaster forecasting significantly leverages predictive analysis to minimize risks and enhance resilience. By analyzing data on geological activity, weather changes, and historical disaster patterns, researchers can create models that forecast events like earthquakes, hurricanes, and floods.
The importance of accurate natural disaster predictions cannot be overstated. Timely forecasts can save lives and reduce property damage. Strategies such as emergency planning and resource allocation are informed by these predictions.
Some key considerations in natural disaster forecasting are:
- Data Quality: High-quality data is essential for accurate modeling and predictions. Poor data can lead to incorrect forecasts, endangering lives.
- Technological Integration: Advanced technologies, like satellite imagery and geographic information systems, enhance predictive capabilities.
- Public Awareness: Effective communication of forecasts to the public is vital for ensuring safety and preparedness.
In summary, predictive analysis in earth sciences is pivotal. Both climate modeling and natural disaster forecasting contribute to our ability to anticipate changes in the earth's systems, enabling societies to adapt and respond effectively.
Challenges and Limitations
Understanding the challenges and limitations of predictive analysis is essential for any researcher or practitioner in scientific fields. Every methodology, regardless of its efficacy, can face obstacles that hinder its accurate application. Addressing these challenges can help improve the reliability and validity of predictive models. Both data quality and the proper fit of models are critical elements to understand in this context. Recognizing these limitations informs future research and applications, shaping better practices and methodologies.
Data Quality Issues
Data quality is a cornerstone of reliable predictive analysis. Poor quality data can lead to misleading conclusions, affecting the outcomes of research significantly. Issues often arise from various sources, including inconsistent data collection methods, sampling errors, or incomplete datasets. Furthermore, external factors, such as environmental influences or technological variances, can introduce noise into the data.
Researchers must prioritize data validation processes. Ensuring the data is accurate, complete, and relevant to the study is critical. Key steps include:
- Data Cleaning: This involves correcting or removing inaccurate records from datasets.
- Source Verification: Confirming that data comes from credible and trustworthy sources.
- Consistency Checks: Regularly comparing datasets to maintain uniformity across different studies.
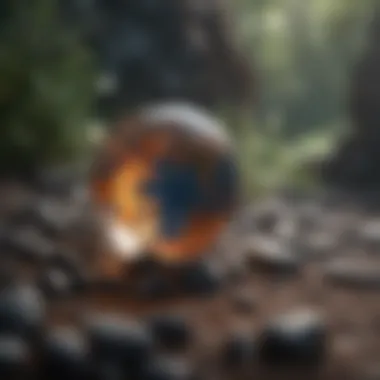
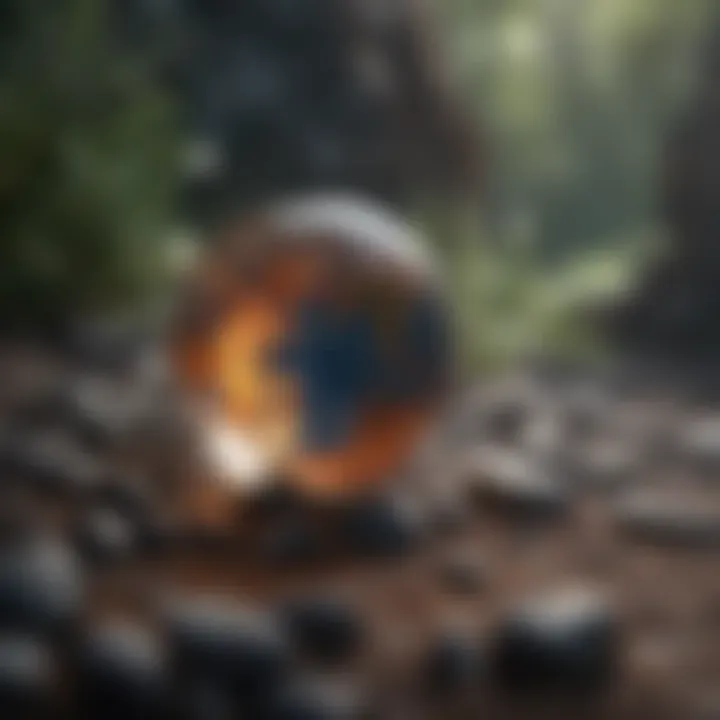
Inadequate attention to data quality can compromise predictive models, resulting in incorrect assumptions and forecasts. It is essential for scientists to regularly assess their data quality and take corrective actions when necessary.
Model Overfitting and Underfitting
Model overfitting and underfitting are two common challenges that can substantially affect predictive analysis. Overfitting occurs when a model learns too much from the training data, capturing noise alongside the actual patterns. Consequently, the model may perform well during training but struggles to generalize to new, unseen data. This can create false confidence in a model's predictions.
In contrast, underfitting happens when a model is too simplistic to capture the underlying patterns in the data. Such a model fails to learn from the dataset effectively, resulting in poor performance even on training data.
To address these issues, researchers often employ various techniques:
- Cross-Validation: This method involves using multiple datasets to train and validate a model. It helps ensure that the model generalizes well.
- Regularization: This technique adds a penalty to the loss function used during training, discouraging complex models that may overfit the data.
- Feature Selection: By choosing the most relevant features in the dataset, researchers can reduce the risk of overfitting, ensuring that only pertinent information influences model predictions.
"Balancing model complexity and data fidelity is crucial for effective predictive analysis."
Identifying whether a model is overfitting or underfitting requires ongoing evaluation and adjustment. Understanding these concepts can significantly enhance the effectiveness of predictive analysis across various scientific disciplines.
Ethical Considerations
Ethics in predictive analysis is a critical area that demands attention. As technology evolves, the implications of using predictive models are becoming more pronounced. It is crucial for researchers and practitioners to understand the ethical dimensions of their work. This section will explore two major ethical concerns: privacy and data security, and bias in predictive models.
Privacy and Data Security
Privacy is a fundamental right, and its protection is essential in the realm of predictive analysis. When extensive datasets are collected, they often include sensitive personal information. This can lead to significant risks if data is mishandled or improperly accessed. Organizations must implement stringent security measures to protect this data from breaches and unauthorized use.
Benefits of strong privacy protocols include:
- Increased trust from the public in scientific research.
- Compliance with legal frameworks such as GDPR, which governs data protection.
- Enhanced data integrity, ensuring that research findings are credible and respected.
Key considerations for maintaining privacy include:
- Anonymizing data to prevent identification of individuals.
- Regular audits and updates to data security practices.
- Engaging with stakeholders to ensure transparency about data usage.
"Ensuring data privacy is not just a legal obligation but also a moral imperative that underpins the integrity of research."
Bias in Predictive Models
Bias in predictive models can lead to skewed results and outcomes that might affect decisions in adverse ways. When data used for these models reflects existing social biases, the predictions can reinforce stereotypes and inequalities. It is important to recognize the sources of data bias, which can arise in various forms, such as historical prejudices embedded in datasets or the demographic underrepresentation in training data.
Addressing bias involves:
- Diverse data collection to include a wider array of perspectives and experiences.
- Ongoing evaluation of models to identify and rectify potential biases.
- Encouraging transparency in algorithms used for predictions.
The implications of bias in predictive models are profound. They can misinform public policy, affect healthcare access, and influence job opportunities. It becomes imperative for researchers to approach predictive analysis with a mindset attuned to ethics and responsibility.
Future Directions in Predictive Analysis
As we look forward in the realm of predictive analysis, its importance continues to grow. The rapid development of computational power and data generation capabilities sets a new stage for scientific research. These advancements will allow predictive models to become more accurate and reliable. The future directions of predictive analysis not only focus on improving existing methodologies but also on exploring new applications and opportunities across various scientific disciplines. Here are several critical aspects of this evolving field.
Advancements in Technology
The intersection of predictive analysis with new technologies promises profound advancements. For instance, the rise of quantum computing is anticipated to revolutionize data processing. Quantum algorithms can potentially handle and analyze data sets at unprecedented speeds, enhancing predictive accuracy. Additionally, the integration of big data technologies, such as Hadoop and Apache Spark, allows for real-time data analysis. This capability will lead to timely insights and more robust predictions in scientific research.
Among the notable advancements are developments in artificial intelligence. Machine learning algorithms are becoming more sophisticated, capable of learning from vast datasets and improving their predictive capabilities over time. These techniques can uncover hidden patterns and relationships within data, which were previously challenging to identify. Moreover, automation in data collection and processing is streamlining workflows, making predictive analyses more accessible to researchers across all fields.
Interdisciplinary Approaches
In the pursuit of more effective predictive models, interdisciplinary collaborations are emerging as a crucial theme. Researchers from diverse fields are coming together to share expertise and insights. This cooperation has proven beneficial in refining methodologies and enhancing the relevance of predictive analysis.
For example, the convergence of biology, informatics, and mathematics is leading to more accurate biological predictions. Similarly, ties between social sciences and data science are driving better understanding of human behavior through predictive modeling. By embracing varied perspectives, the depth and applicability of predictive analysis can be expanded significantly.
Engaging in interdisciplinary communication can also address and mitigate identified challenges. Creating standards for data sharing and collaboration across disciplines can enhance data quality and provide more meaningful predictions.
Collaboration and advancements set the stage for predictive analysis to impact scientific research significantly in the coming years.
Culmination
The conclusion of this article synthesizes the insights gained through various sections about predictive analysis and its significance in scientific research. The examination of predictive models illuminates their capacity to assist researchers in making informed decisions based on data-driven forecasts. This emphasis is particularly crucial across different disciplines such as biology, chemistry, physics, and earth sciences.
Summary of Key Insights
Through our exploration, it becomes evident that predictive analysis is multifaceted. Key insights from this narrative include:
- Predictive modeling thrives on accurate data collection and preprocessing. Data quality directly impacts outcomes.
- Statistical techniques and machine learning approaches bolster predictive accuracy, allowing for deeper insights into complex systems.
- Applications such as disease prediction in biology or material property assessments in chemistry exemplify the utility of these analytical methods.
- The ethical considerations, such as bias and data privacy, are significant for responsible use of predictive analytics.
Overall, predictive analysis serves as an essential tool that helps in not just recognizing patterns but also in gaining foresight into future events based on current and historical data.
Implications for Future Research
Moving forward, the landscape of predictive analysis continues to evolve. Several implications arise for future studies, particularly regarding:
- Integration of advanced technologies such as artificial intelligence and big data analytics may enhance predictive accuracy and efficiencies.
- Broader interdisciplinary collaborations might arise, merging various scientific approaches and methodologies to create robust predictive frameworks.
- Ethical frameworks must also evolve. This includes ensuring models are unbiased and that data privacy is respected while pursuing scientific advancements.