Understanding Source Data Review in Scientific Research
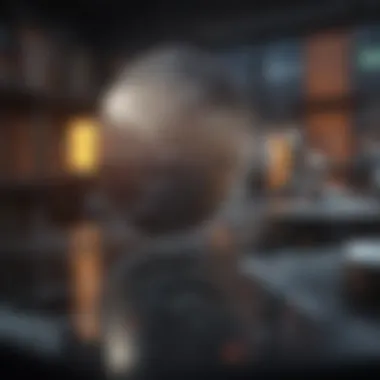
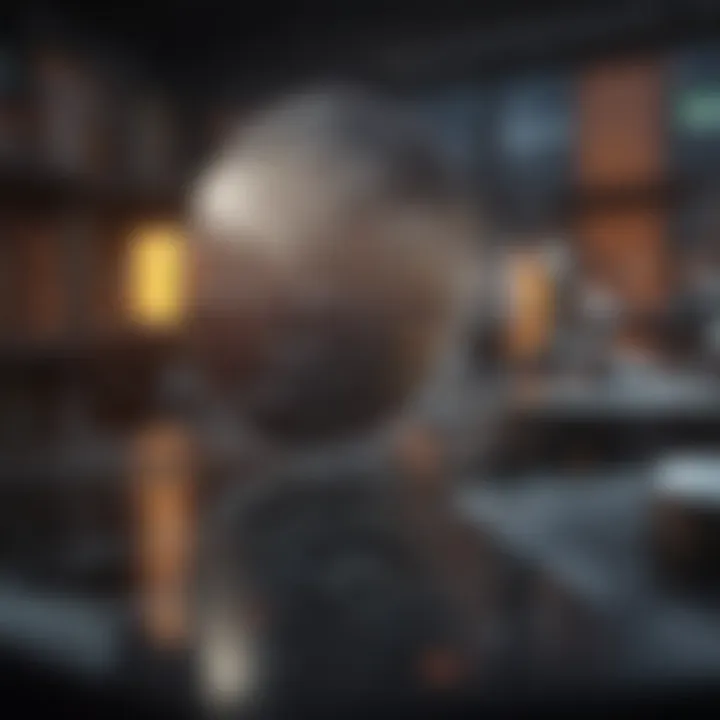
Intro
In the realm of scientific research, the bedrock of reliability hinges on the scrutiny of source data. When researchers put pen to paper or click the mouse to craft their findings, it becomes paramount that the data supporting their claims withstands rigorous examination. In essence, source data review is not merely a checklist; it’s a thorough process that can make or break research integrity.
As anyone who's spent time in the lab can attest, the road to knowledge is paved with facts and evidence. Yet the journey is often riddled with challenges—from data inconsistencies and human errors to the ever-present specter of bias. Recognizing and addressing these issues is critical for ensuring that the research can be trusted.
Throughout this article, we will explore various aspects of source data review. We’ll unpack common methods used in evaluation, discuss challenges faced by researchers, and offer best practices that can be easily adopted across disciplines. In doing so, we aim to enhance your understanding of why source data review is not just a step in the research process but a cornerstone of valid scientific inquiry.
Understanding Source Data Review
In the realm of scientific inquiry, the phrase "source data review" carries significant weight. It refers to the rigorous examination of data collected throughout research processes. A thorough understanding of this topic is vital. The integrity of research findings hinges upon the validity and reliability of the data reviewed. If the foundation—namely, the source data—is flawed, then any conclusions drawn may ultimately lead to misinterpretations and incorrect assumptions.
The importance of source data review extends beyond mere accuracy; it acts as a gatekeeper of quality. This process ensures researchers adhere to ethical standards and maintain transparency in their work. By respecting the principles of source data review, researchers contribute to a culture rooted in reliability. Consequently, this solidifies the trust the public bestows upon scientific findings.
Moreover, the implications of not undertaking a thorough review are severe. Instances of data manipulation or negligence can result in misleading results, which, once published, may misguide further research and public policy. Therefore, understanding the nuances of source data review is not simply an academic exercise—it reflects a commitment to the advancement of knowledge.
Definition and Importance
Defining source data review can initially seem straightforward—it’s essentially a critical evaluation process of the information gathered during research. However, this definition belies its complexities. It involves scrutinizing data sources, assessing their reliability, and determining the methods used for collection.
The importance of this review cannot be overstated. For one, it fosters scientific rigor by ensuring that studies are repeatable and replicable. Second, adhering to strong review practices can significantly reduce biases that may infiltrate research outcomes. It is thus a safeguard that cultivates a culture where findings can be trusted and built upon in future studies.
Lest we forget, the role of peer review weaves seamlessly into the fabric of source data review. It provides that added layer of scrutiny that is indispensable in championing accurate scientific discourse.
Historical Context of Data Review
To appreciate the current practices in source data review, it helps to take a stroll down memory lane. Historically, the act of reviewing data isn't anything new. Early scientific thinkers, from Aristotle to Galileo, recognized the need for rigorous examination of findings, albeit without today's tools and methodologies.
In more contemporary settings, the mid-twentieth century marked a turning point. With the advent of advanced statistical methods, researchers began to place a greater emphasis on data review. Initiatives aimed at standardizing data practices started to crop up, highlighting the need for clear guidelines.
Fast-forward to the present day, and the landscape has transformed yet again—technological advancements like digital data management systems allow researchers to conduct more thorough reviews with unprecedented efficiency. Thus, while the essence of source data review hasn't changed, its approach has evolved, driven by a commitment to maintain credible research standards in an increasingly complex scientific arena.
Key Components of Source Data Review
Understanding the key components underlying source data review is crucial to appreciating how rigorous research is conducted. The nuts and bolts of data review helps to maintain the integrity and trustworthiness of research outcomes. This section encapsulates the essence of various factors, laying bare the intricate interplay between them and their cumulative impact on the quality of scientific inquiry. The right approach ensures not only adherence to scientific standards but also enhances comprehension among peers and stakeholders.
Data Collection Methods
Qualitative vs. Quantitative Methods
When delving into the realm of data collection, one cannot overlook the distinction between qualitative and quantitative methods. These two paradigms serve different but equally important purposes. Qualitative methods largely focus on understanding behaviors and experiences through narrative data, enhancing the depth of research. On the flip side, quantitative methods provide numerical insights, allowing for robust statistical analyses that can identify patterns across larger populations.
One significant advantage of qualitative methods is their ability to unearth nuances that numbers alone cannot capture. For example, in a study exploring patient satisfaction, interviews can yield rich insights that a simple survey would miss. Conversely, quantitative methods can offer objective measurements, like the percentage of patients satisfied, lending credibility to one’s findings.
Surveys and Questionnaires
Surveys and questionnaires often serve as the backbone of data collection in many research fields. A well-structured survey can reach a vast audience, providing a snapshot of opinions or behaviors efficiently. The beauty of such instruments lies in their versatility—tailoring questions to suit specific research needs means researchers can capture precisely what they aim to explore.
However, crafting an effective survey necessitates thoughtful consideration. Poorly framed questions can lead to ambiguous responses, diluting the quality of the data collected. For instance, a leading question can skew results, thereby impacting outcomes. To mitigate such risks, employing pilot tests or pre-testing the instruments is advised.
Experimental Procedures
Experimental procedures are typically more controlled than surveys and can lend themselves to more definitive conclusions about causation. This method involves manipulating one variable to observe its effect on another while keeping all other factors constant. This controlled environment allows for clearer relationships to be established, which is essential in empirical research.
The strength of experimental procedures is their ability to provide reliable data through repeatability and robust designs. One might argue that they come with high internal validity. Nevertheless, these setups may not always reflect real-world conditions, often leading to questions about external validity and generalizability. Therefore, researchers must balance rigor with practical applicability when designing their experimental frameworks.
Data Validation Techniques
Cross-verification with Existing Data
Cross-verification with existing data is a foundational aspect of ensuring the integrity of new research. By comparing new findings to established datasets, researchers can assess the consistency and reliability of their results. For example, if a new study on drug efficacy yields outcomes that starkly contrast with existing literature, this discrepancy raises red flags.
This technique’s major benefit lies in reinforcing the credibility of findings. Consistency across different datasets often strengthens the final conclusion. However, researchers must be cautious; reliance on outdated or biased data can lead them astray.
Statistical Analysis for Accuracy
In the world of empirical research, statistical analysis plays a pivotal role in data validation. This technique helps to determine whether observations are genuinely significant or just the result of random variability. By applying various statistical models and tests, researchers can quantify the reliability of their data, thereby asserting its validity.
The capability to infer conclusions with statistical backing significantly bolsters a study's acceptance. Nevertheless, one must engage in careful interpretation, as misapplication or misinterpretation of statistical techniques can render results invalid—even if computed accurately.
Automated Validation Tools
As technology advances, automated validation tools are becoming vital players in the field of data validation. These applications can swiftly analyze massive datasets, identifying anomalies and patterns with precision. This not only saves time but also mitigates human error, leading to more consistently reliable outcomes.
The unique feature of automated tools lies in their capacity for continuous monitoring—as datasets evolve, these tools can provide real-time validation, which is invaluable in dynamic research environments. Nonetheless, such reliance also means that researchers must understand the tools they are employing; blind reliance can lead to complacency and oversights.
"Data validation, much like a fine sieve, ensures only the best information makes its way into the heart of research."
By meticulously weaving together these components of data collection and validation, researchers can fortify their studies, ensuring that their findings stand up to scrutiny and contribute meaningfully to the broader academic discourse.
Challenges in Source Data Review
Engaging in source data review is like walking a tightrope; the need for accuracy is precariously balanced against the inevitable challenges that arise during the process. It is crucial for researchers to understand the multifaceted nature of these challenges to ensure their findings hold water in scientific discourse. This section delves into two key challenges: bias in data collection and issues arising from incomplete or inaccurate data.
Bias in Data Collection
Understanding and addressing biases within data collection is essential for meticulous source data review. Bias can seep into research at various stages and, if unaddressed, can distort the very conclusions that researchers aim to make. The following subsections shed light on specific types of biases and methods to navigate them.
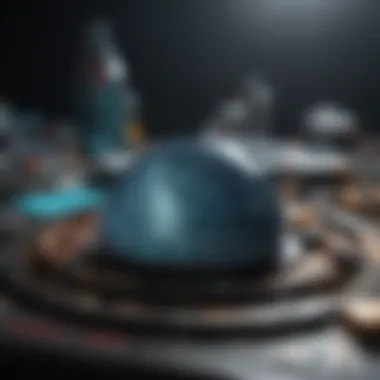
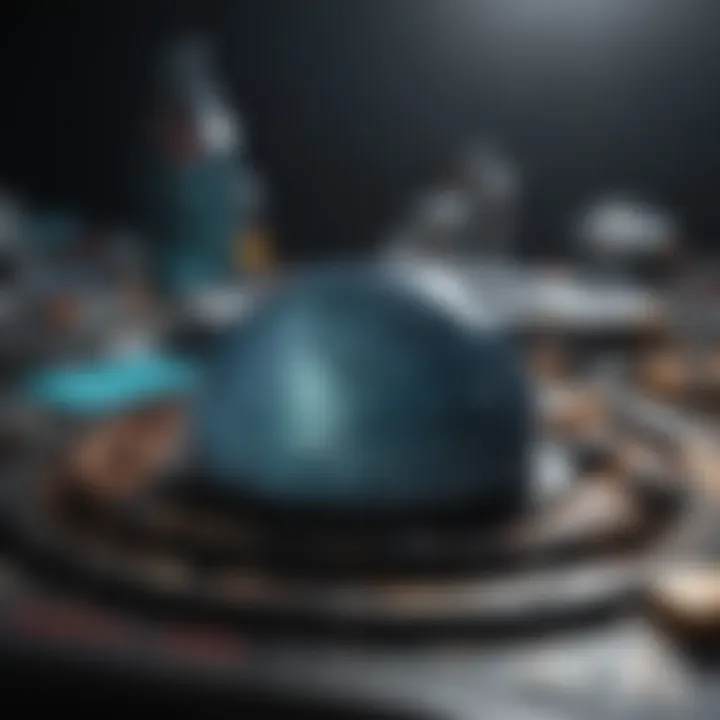
Understanding Selection Bias
Selection bias occurs when certain individuals or data points are disproportionately represented in the study due to systematic differences in their selection. It can skewer results and lead to skewed conclusions. This type of bias is a prominent issue because it can create a facade of reliability in studies where it does not exist. For example, if a clinical trial predominantly includes healthy participants, the outcomes might present an overly optimistic view of a medical intervention's efficacy. Addressing selection bias safeguards the core integrity of research findings, making it a focal point of this exploration. Recognizing its unique characteristics—how it manifests in various settings—helps researchers develop robust methodologies that minimize its effects.
Mitigating Researcher Bias
Researcher bias, stemming from the personal beliefs or expectations of those conducting the study, can lead to selective reporting or interpretation of results. This bias is particularly insidious as it often goes unnoticed until it significantly affects the conclusions drawn. Researchers must be consciously aware of their own biases and actively work to counteract them. This active reflection is a beneficial practice not only for individual studies but also for fostering a culture of transparency in scientific research. A tailored approach, such as blind data analysis or diverse review boards, can lessen these effects. Ultimately, understanding and mitigating researcher bias fosters a more honest portrayal of results, enhancing the article's credibility.
Addressing Confirmation Bias
Confirmation bias tends to manifest when researchers seek or emphasize data that supports their pre-existing beliefs while disregarding contradicting evidence. This bias can compromise the objectivity of research and lead to significant misinterpretations of findings. Acknowledging this bias is essential for researchers striving for thoroughness and accuracy. It’s valuable for this article to spotlight confirmation bias—its patterns, and potential fallout—because it directly relates to how rigorous source data review must be. Employing strategies like pre-registration of studies, where methodologies and hypotheses are established before the data is analyzed, serves as a proactive approach to minimizing confirmation bias. This balanced approach not only enriches the research but also adds a layer of rigor necessary for sound scientific inquiry.
Incomplete or Inaccurate Data
Incomplete or inaccurate data can act as a fly in the ointment, rendering the best methodologies futile. To uphold the highest standards of scientific research, being able to identify, evaluate, and manage these data shortcomings is vital.
Identifying Data Gaps
Detecting gaps in the data is akin to piecing together a jigsaw puzzle with missing pieces. If a study lacks comprehensive datasets, this shortfall can skew results and lead to invalid conclusions. Detecting these gaps early can direct researchers to either seek additional data or adjust their study designs. Highlighting this point in the article is invaluable, as it calls attention to the necessity of rigorous oversight throughout the research process. Fostering an environment that prioritizes thorough data collection practices can reduce the likelihood of gaps occurring.
Impact of Data Errors
Data errors can arise from various sources, be it human mistakes during data entry or flawed algorithms in data processing. The impact of these errors can propagate through research results, leading to misguided interpretations. Recognition of data errors, especially in fields like epidemiology, where a single incorrect entry could alter public health conclusions, must be emphasized. This topic is crucial for the article, presenting an opportunity to underscore the importance of meticulous review processes at all stages of data handling. Incorporating routine checks and balances can significantly counteract the risk of data errors.
Strategies for Rectification
Implementing effective strategies for rectifying inaccuracies and gaps in data is paramount. Researchers should develop systematic protocols for data validation to ensure that datasets remain robust. Establishing regular audits or cross-verification with other credible datasets enhances quality assurance and rectification efforts. It ’s beneficial for this article to discuss various strategies like these so that researchers can come away equipped with actionable solutions. Correcting identified issues not only improves the validity of current findings but also reinforces the foundational principles of rigorous scientific research.
To foster scientific integrity, data review processes must address challenges posed by biases and data inaccuracies. The commitment to quality data in research underpins the reliability and applicability of study outcomes.
Best Practices for Effective Source Data Review
In the realm of scientific research, the practice of source data review stands as a cornerstone for ensuring the reliability and integrity of findings. Implementing best practices not only enhances the quality of research but also boosts the confidence of the scientific community in published work. By following structured protocols, researchers can systematically identify discrepancies, verify authenticity, and maintain the rigorous standards expected in scholarly efforts.
Establishing Clear Guidelines
Framework for Review Procedures
A solid framework for review procedures serves as the backbone of any effective data review process. By outlining precise steps and methodologies, researchers create a roadmap that guides them through the review. This varies widely among different fields; in clinical research, for instance, a step-by-step protocol often includes stages for data entry, validation, and analysis.
One key characteristic of this framework is its adaptability. Different disciplines can tailor the framework to suit their specific needs and challenges. Its structured nature helps minimize errors and omissions, making it a popular choice among researchers looking to enhance overall data quality. Moreover, it often includes unique features like checkpoints for various stages of data analysis, ensuring that any discrepancies are caught early in the process. However, setting up such a framework can be time-consuming, requiring a significant initial investment of resources.
Documentation Standards
Equally important in the review process are the documentation standards employed by researchers. These standards ensure that all data collection and review processes are meticulously recorded, safeguarding against the loss of crucial information. The essential characteristic of documentation standards is their role in fostering transparency. By maintaining high-quality documentation, researchers can trace their steps and methodologies, making it easier for others to replicate their studies.
A particular benefit of stringent documentation is that it promotes accountability among research team members. Each entry must be justifiable and traceable back to the original source data. Yet, an inherent disadvantage is the potential for overwhelming workloads, which could lead to burnout among researchers. They may find it tedious to keep meticulous records when under time constraints.
Ethical Considerations
Ethical considerations in source data review play a crucial role in maintaining the integrity of scientific research. Adhering to ethical standards helps researchers avoid misconduct, such as data falsification or misrepresentation. The key characteristic of ethical considerations is their focus on protecting human subjects and maintaining their confidentiality. It becomes particularly pertinent in fields like social sciences, where personal data is often involved.
Incorporating ethical guidelines not only fosters trust within the research community but also enhances the credibility of findings presented. However, researchers must be wary of strict adherence leading to paralysis by analysis, where the worry of ethical breaches hinders decision-making. Balancing ethical obligations with the need for timely research is a nuanced yet vital aspect of conducting reputable research.
Collaboration and Peer Review
Engaging Diverse Perspectives
Engaging diverse perspectives during source data review adds layers of understanding that a singular viewpoint often misses. Incorporating feedback from a spectrum of stakeholders—be it statisticians, specialists from different fields, or even ethicists—can yield a more rounded evaluation of the data. One key characteristic of this approach is that it encourages critical thinking and robust discussion, which ultimately enriches the research process.
By fostering an environment where various viewpoints are welcomed, researchers can identify blind spots in their analysis that may otherwise go unnoticed. On the flip side, navigating differing opinions can lead to increased conflict and slow down decision-making. Thus, while collaboration enhances depth, it also introduces complexity to the review process.
Benefits of Cross-disciplinary Input
The advantages of cross-disciplinary input cannot be overstated. It combines knowledge from varied fields to address complex problems, enriching the data review process. For example, a team including environmental scientists and public health experts can offer insights that may significantly improve the quality of a study addressing climate change and health impacts.
The key characteristic of this approach is the infusion of innovative methodologies and frameworks drawn from different disciplines. Such diversity encourages researchers to think outside their usual paradigms. However, the challenge lies in communication; jargon and terminologies unique to each field can create barriers. Furthermore, such collaboration usually requires more time to align perspectives.
Implementing Feedback Mechanisms
Feedback mechanisms serve as the glue that holds the source data review process together. These mechanisms allow researchers to receive constructive criticism aimed at improving both the data quality and the interpretations drawn. A major characteristic of effective feedback mechanisms is their regularity; scheduled reviews often yield better outcomes compared to sporadic feedback sessions.
A unique feature of robust feedback systems is the potential for iterative refinement of research output. By continually re-evaluating findings against accumulated critiques, researchers can enhance the final product significantly. Conversely, establishing such systems can be resource-intensive, demanding commitment and discipline from all team members involved.
By applying these best practices, researchers can elevate the standard of source data review, thereby ensuring that scientific work remains credible and impactful.
Technological Advancements in Data Review
The pace of development in technology significantly influences how source data is reviewed in scientific research. This section elucidates the transformative impact of these innovations, focusing on the benefits they bring, the specific technologies in play, and the considerations researchers must keep in mind while adopting these advancements.
Role of Big Data Analytics
Big data analytics is revolutionizing the way researchers handle and interpret vast amounts of data. It allows scientists to sift through large datasets quickly, identifying patterns that may be missed by manual review. One standout characteristic of big data analytics is its ability to process real-time data, enabling timely insights that can influence research outcomes.
- Efficiency: This speed of analysis not only enhances the workflow but also bolsters the credibility of the research results.
- Complexity Handling: The ability to handle complex datasets offers researchers a clearer picture of the variables at work. This technology stands out as a popular choice for those aiming to leverage huge amounts of information for more nuanced conclusions.
Yet, it's worth mentioning that big data analytics also carries some challenges.
- Data Overload: The sheer volume of data often leads to information overload, where the key insights can get lost in the noise.
- Skill Requirements: Running these analyses requires specific skills that might not be within reach for every researcher.
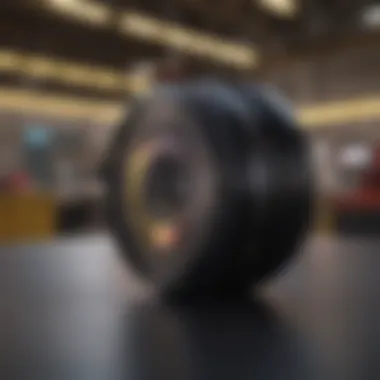
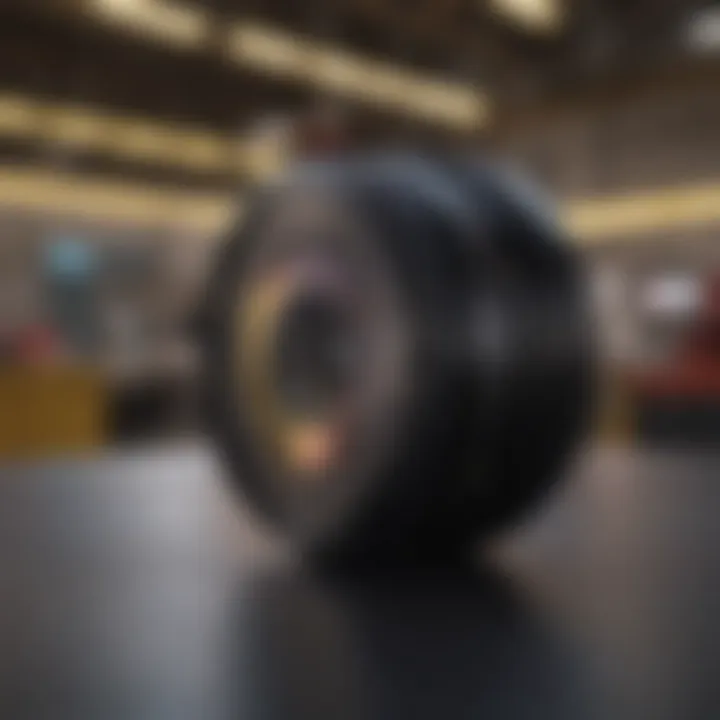
Data Mining Techniques
Data mining techniques contribute significantly to extracting valuable information from raw data. These methods are geared towards discovering hidden patterns. A defining characteristic of data mining is its ability to utilize algorithms for classification and clustering, which streamlines data analysis.
- Pattern Recognition: For example, scientists can uncover trends in clinical data that indicate potential treatment effects.
- Predictive Power: This unique feature allows data mining to predict future trends based on historical data, making it a favorable choice in research that depends on such foresight.
However, data mining isn’t without its downsides.
- Risk of Overfitting: Sometimes, the models created can overspecialize, failing when applied to new data situations.
- Resource Intensive: The need for substantial computational resources can hinder smaller teams from utilizing these techniques.
Machine Learning Applications
Machine learning applications have made a mark by enhancing data review processes through automation and advanced algorithmic approaches. The crux of machine learning lies in its adaptability—algorithms improve as they are exposed to more data. This characteristic makes it an essential tool for the analysis of large datasets, as it vastly increases the accuracy of models built for research.
- Dynamic Learning: It provides researchers an edge as they can adjust guidance based on new data flows.
- Versatile Applications: The breadth of scenarios in which machine learning can be applied—from clinical research to environmental studies—highlights its significance in enhancing the efficacy of data review.
Nonetheless, the reliance on machine learning does introduce its challenges.
- Algorithmic Bias: Decisions made through these methods can carry biases stemming from the initial dataset.
- Complexity: To properly implement machine learning requires a robust understanding, which can pose a barrier for some researchers.
Predictive Modeling for Data Accuracy
Predictive modeling is crucial for maintaining accuracy in research data. This approach forecasts future outcomes based on historical data trends. A key aspect of predictive modeling is its capacity for scenario simulation, which allows researchers to visualize different possibilities based on varying inputs.
- Enhanced Decision Making: It aids in informed decision-making—researchers can weigh the potential consequences of their choices before acting.
- Resource Allocation: This technique offers the ability to optimize resources by predicting where efforts will be most effective, making it a desirable method within this article’s context.
However, like other techniques, it presents some challenges.
- Dependence on Quality Data: The effectiveness of predictions is directly tied to the quality and comprehensiveness of the input data.
- Complex Models: Creating these models can be complex and time-consuming, often requiring significant expertise.
Artificial Intelligence in Data Validation
AI technologies are reshaping the landscape of data validation processes. They enhance data integrity and ensure reliability through automation and advanced analysis capabilities.
Automated Quality Checks
Automated quality checks are turning the tide in how data validity is established. These checks can sift through data at staggering speeds, identifying errors more efficiently than manual reviews. One important feature of these checks is their consistency; they follow strict algorithms, ensuring that the same criteria are applied across datasets.
- Error Detection: This consistency allows for rapid identification of any discrepancies, which is vital in maintaining the quality of research outcomes.
- Scalability: Given their ability to handle massive datasets, automated quality checks serve as a beneficial tool in this article by enabling researchers to review data more thoroughly.
Still, there are limitations to consider.
- Lack of Context Understanding: Unlike human reviewers, automated systems may struggle to grasp the context of certain anomalies.
- Maintenance Needs: Regular updates are necessary to ensure these systems remain effective, which can require additional resources.
Intelligent Data Processing
The emergence of intelligent data processing has further streamlined the data review phase. This approach leverages AI to adapt and optimize data handling based on specific research needs. One standout feature is its ability to learn from every interaction, thereby becoming increasingly more efficient in its processing tasks.
- Customization: This allows researchers to tailor their data handling approaches and ensures relevant insights are highlighted.
- Interconnected Systems: This interconnectedness serves as a boon for those trying to analyze complex relationships in their data, making it an effective choice for improving research quality.
However, reliance on intelligent data processing can come with issues.
- Complex Integration: Integrating such advanced systems into existing infrastructures can pose challenges.
- Dependency on Data Quality: As with predictive models, the success of intelligent data processing hinges on the quality of the data itself.
AI-driven Insights for Review
AI-driven insights are transforming the analytical landscape in scientific research. These insights generate hypotheses and contribute novel perspectives based on data analysis. The primary characteristic of this approach is its capacity for deep learning, which can uncover insights that might elude human interpretation.
- Innovative Findings: This feature equips researchers with new angles to explore, making it an essential tool in their toolkit.
- Rapid Analysis: The speed at which AI can analyze and present insights fosters a more dynamic research culture and is a popular approach discussed in this article.
Yet, the use of AI-driven methods also has its drawbacks.
- Interpretability Issues: Often, the conclusions drawn by AI systems are difficult for humans to comprehend, which can lead to confusion and skepticism about findings.
- Resource Investment: The set-up costs and ongoing operational expenses can be substantial.
In summary, technology is reshaping source data review processes, ushering in more profound insights and enhancing the thoroughness of evaluations. While there are challenges associated with these advances, the benefits they offer for accuracy and efficiency in scientific research are undeniable.
Case Studies of Source Data Review
Case studies in the realm of source data review serve as informative anecdotes that allow us to stitch together a richer understanding of research practices. They provide concrete examples of how effective data review can shape research outcomes. This section reflects on instances where methodologies in data review were not only applied but led to transformative results in various fields. From biological studies addressing life-saving discoveries to environmental research that impacts global sustainability, the implications of adhering to stringent data review norms are profound and far-reaching.
Successful Implementations
Biological Research
In biological research, source data review stands as a pillar of scientific rigor. One prominent aspect of biological research is the investigation of gene editing technologies. This field has made tremendous strides toward potential treatments for genetic disorders. A significant example includes a study employing CRISPR technology aimed at addressing sickle cell disease.
The process of source data review highlighted the importance of accuracy in gene targeting. The key characteristic of biological research lies in its focus on real-life applications; findings can lead directly to medical breakthroughs. This approach also fosters collaboration among scientists, clinicians, and policy-makers, creating a dynamic ecosystem of knowledge. A unique feature of biological research is its potential for rapid translation into clinical practice, but it carries disadvantages such as ethical debates surrounding gene editing, making thorough data review essential.
Environmental Studies
Environmental studies have become ever more critical as climate change poses pressing challenges. A specific aspect of this research often revolves around biodiversity assessments. A notable case was when researchers examined the impact of deforestation on local wildlife in the Amazon basin. Here, source data review helped verify field observations and remote sensing data accuracy.
The collaborative nature of environmental research serves as its key characteristic. Researchers typically work with indigenous populations and local governments to gain insights and ensure that findings are holistic. The unique feature of integrating community knowledge enhances data richness, but the complexity of environmental data can lead to difficulties in cross-validation, necessitating robust source data review processes.
Clinical Trials
Clinical trials epitomize the significance of source data review, particularly when assessing new pharmaceuticals. An exemplary aspect of this field is the rigorous evaluation protocols established by the FDA. In a recent trial for a heart medication, thorough data review processes identified discrepancies in patient reporting, which could have otherwise led to misleading conclusions about the drug's efficacy.
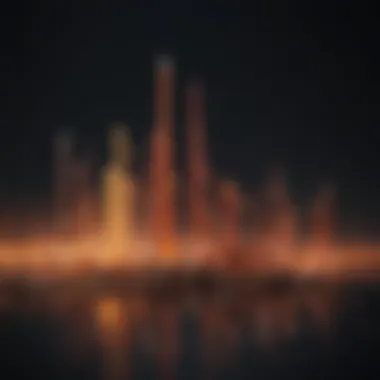
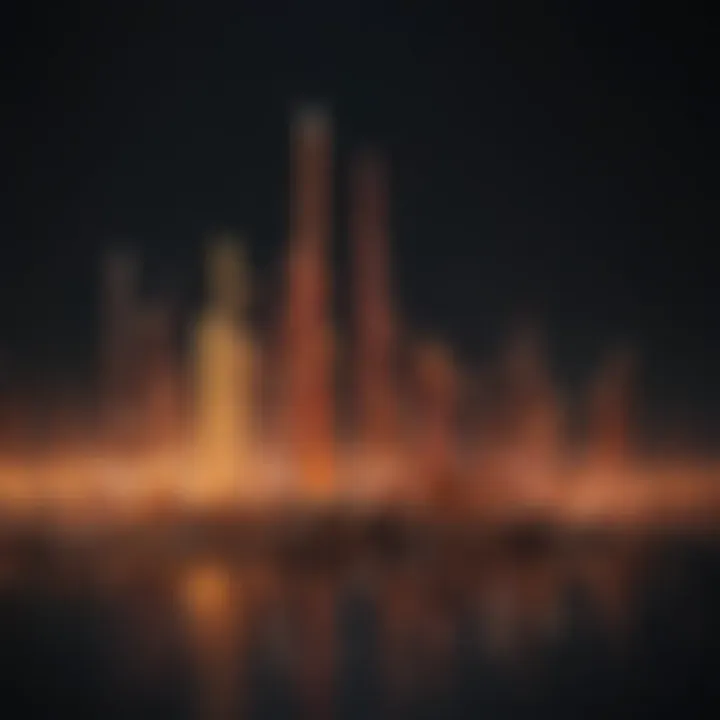
The rigorousness inherent in clinical trials marks its key characteristic. This structure not only protects participants but also elevates the reliability of outcomes. One unique feature is the dependence on long-term follow-up data, which presents both advantages, such as comprehensive safety assessments, and disadvantages, like potential attrition bias among participants. The role of source data review in clinical trials cannot be understated, as it actively safeguards against threats to research integrity.
Failures and Lessons Learned
High-Profile Research Retractions
At times, the story of source data is not one of triumph but rather cautionary tales. High-profile research retractions have surfaced as pivotal moments that illuminate the potential pitfalls of inadequate data review. Notable cases such as those involving fraudulent practices highlight the dire consequences of neglecting source data integrity. The retraction of a study purporting to link vaccines to autism demonstrates how the lack of rigorous peer review can lead to widespread misinformation and public health crises.
The characteristic of high-profile retractions lies in their illuminating nature; they reveal the vulnerabilities present in the research process, prompting calls for enhanced scrutiny. The unique feature of these cases serves as a sobering reminder that trust in science relies on the data’s authenticity, showcasing how improper reviews can dismantle reputations and halt advancements in public health measures.
Implications of Poor Data Review
Navigating the complexities of research without robust data review mechanisms can lead to severe repercussions. Poor data integrity can result in false conclusions, potentially delaying critical advancements in fields like medicine or technology. An illustrative instance relates to a study on artificial sweeteners, where data inaccuracies sparked a heated debate among scientists and the public regarding their safety.
Poor data review has the characteristic of creating ripples that extend well beyond the immediate publication. When foundational studies are flawed, they can skew subsequent research, creating a cascade of misinformation. The unique aspect here is the impact on public policy; flawed data can lead to misguided regulations or health recommendations, underscoring the profound necessity for rigorous source data review processes.
Strategies to Avoid Pitfalls
To mitigate the risks associated with frail data review practices, various strategies can be adopted. Firstly, implementing structured review protocols, akin to those utilized in high-stakes environments like healthcare, establishes a foundation for accountability. For instance, employing external audits can help maintain objectivity and further reinforce data validity, creating a robust feedback loop.
The key characteristic in this regard is the proactive stance taken to eliminate potential errors before they inflate into larger problems. A unique feature of these strategies is the emphasis on interdisciplinary collaboration; involving statistical experts in the review process enhances depth and broadens the analytical lens applied to data. This not only boosts research quality but also helps prevent the missteps that have marred past research efforts.
"The importance of strict source data review cannot be overstated, as the integrity of research findings rests upon it, influencing policy, public perception, and future inquiry."
In sum, the case studies outlined here illuminate the necessary pillars supporting the endeavor of scientific research. Whether successful implementations showcase the power of collaborative effort or failures warn of the repercussions of poor oversight, they all resonate with the core message: robust source data review is non-negotiable in the pursuit of scientific truth.
Future Directions in Source Data Review
As we navigate the rapidly evolving landscape of scientific research, the importance of source data review cannot be overstated. This section explores future directions, considering cutting-edge trends and technologies, as well as the critical need for ongoing education among researchers. Both elements are essential to strengthen the integrity of research and ensure valid outcomes.
Emerging Trends and Technologies
Integration of Blockchain for Data Integrity
The integration of blockchain in data management presents a groundbreaking approach to preserving data integrity. One of its core characteristics is the unchangeable nature of blockchain, which locks data in a way that ensures fraudulent alterations are nearly impossible. This aspect appeals to the scientific community that prioritizes authenticity. With this technology, researchers can trace the provenance of their data, providing transparent records of how information was collected, processed, and analyzed.
Advantages of Incorporating Blockchain:
- Enhanced data security: No centralized point of failure reduces the risk of tampering.
- Improved trust: Stakeholders can verify data integrity independently.
Disadvantages include complexities in understanding and adopting the technology, especially for smaller labs with limited resources.
Use of Cloud-based Review Platforms
Cloud-based review platforms are gaining traction for their ability to facilitate collaboration among researchers. One key feature is accessibility; teams can work on projects from various geographical locations without skipping a beat. This is particularly beneficial for multidisciplinary projects where insights from diverse fields are necessary.
Advantages of Cloud Platforms:
- Scalability: Easily accommodates varying workloads.
- Cost-effectiveness: Reduces the need for physical infrastructure.
However, potential challenges include data security concerns and reliance on internet connectivity.
Future of Collaborative Data Review
The future of collaborative data review revolves around participatory approaches that engage multiple stakeholders, from academia to industry. A significant characteristic is the democratization of research, where insights from various disciplines converge. This approach cultivates an environment rich in diverse perspectives.
Benefits of Collaborative Review Include:
- Broadened understanding of data: Different experts contribute unique insights.
- Optimized solutions: Diverse teams often devise creative solutions to complex problems.
Yet, challenges may arise from differences in disciplinary standards and communication styles, which could create barriers for effective collaboration.
Importance of Continuous Education
Training Programs for Researchers
Training programs are fundamental in keeping researchers up-to-date with the latest methodologies and tools in data review. Tailored curriculums equip scholars with not only theoretical knowledge but also practical skills essential for effective data management.
Benefits of Training Programs:
- Hands-on experience with new technologies.
- Improved competence and confidence in reviewing source data.
Such programs may require investment, and access can vary depending on institutional resources.
Workshops and Publications
Workshops and publications serve as vital gateways for disseminating knowledge within the research community. Workshops allow for real-time feedback and interaction, enhancing the learning experience. Publications, whether academic articles or practitioner guides, continue to disseminate cutting-edge developments and best practices relevant to source data review.
Usefulness of Workshops and Publications Include:
- Networking opportunities that can lead to collaborations.
- Staying current with new trends and advancements in the field.
On the flip side, reliance on self-directed learning from publications may not provide the same level of engagement as hands-on workshops.
Encouraging Lifelong Learning
A culture that encourages lifelong learning is pivotal in the rapidly changing landscape of scientific research. Researchers benefiting from this culture remain adaptable and capable of embracing new technologies and methodologies.
Advantages of Continuous Learning:
- Fostering innovation as researchers continuously refine their knowledge.
- Strengthening professional identity and role in the research community.
Challenges may include balancing research duties with educational commitments, especially for early-career researchers.
In summary, the future of source data review is marked by technological advancements and a steadfast commitment to continuous education. As researchers harness these emerging trends, they not only enhance their own capabilities but also contribute to the integrity and reliability of scientific inquiry.