Understanding Black Box AI: Challenges and Ethics
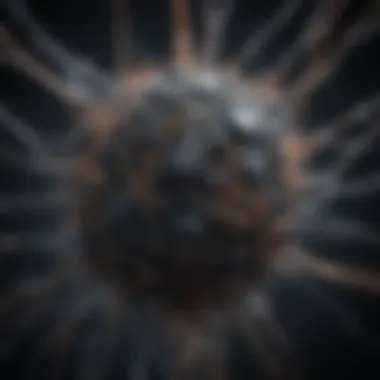
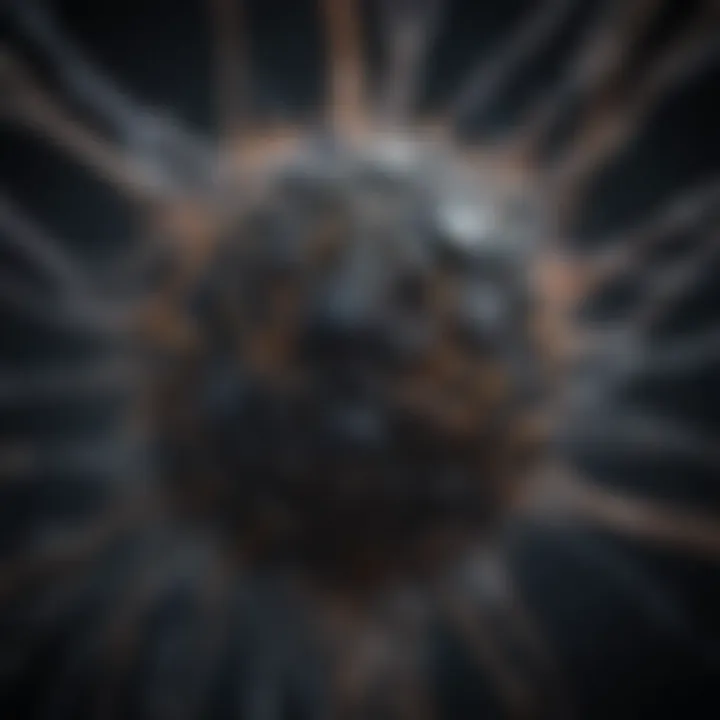
Intro
In recent years, the rapid development of artificial intelligence has transformed numerous fields, but the emergence of black box models has stirred quite a debate among technologists and ethicists alike. Black box AI, as it's commonly referred to, raises significant questions about transparency, interpretability, and trust. Unlike traditional algorithms, these models often function in ways that are not readily understandable even to their creators. As a result, their application in critical areas—such as healthcare, finance, and justice—poses a unique challenge. Navigating this intricate landscape requires a deeper understanding of both their inner workings and the ethical ramifications they introduce.
While the allure of black box models lies in their ability to process vast amounts of data and identify patterns that would otherwise remain hidden, this capability comes with an inherent risk. When algorithms make decisions that are opaque, they can inadvertently perpetuate biases and lead to unfair outcomes. Thus, as we delve deeper into this topic, we will explore not only the advantages these models bring but also the comprehensive implications they carry in our decision-making processes.
As we embark on this journey through the complexities of black box AI, it’s essential to grasp where it all began, how it has evolved, and the pressing need for interpretability without compromising on performance. Understanding these facets will not only equip us with the tools to critically engage with this technology but also guide us in pursuing ethical practices in its development and deployment.
Research Highlights
Overview of Key Findings
Our exploration reveals several key insights into black box AI:
- Translucency vs. Transparency: There's a fine line between being opaque and being comprehensible. Some models can be tweaked to offer a level of transparency without undermining their predictive power.
- Trust and Acceptance: The uptake of black box systems hinges greatly on users' trust. This is often governed by how transparently developers communicate the model's workings and outputs.
- Ethical Considerations: There's a critical need for ethical frameworks to ensure systems are designed with fairness in mind.
These findings reflect not only the complexities of black box models but also the opportunities available for improvement—both in technology and in the processes that surround it.
Significance of the Research
The implications of our findings are profound. By addressing the challenges posed by black box AI, we can enhance the dialogue surrounding ethics and technology. More importantly, it urges a reconsideration of how we approach AI design and implementation. Emphasizing ethical considerations and transparency may pave the way for broader acceptance and integration of AI systems in society. The ultimate goal is striking a balance where innovation flourishes alongside ethical standards.
"If the machine can learn, so can we learn from it—we just need to ensure our learning doesn't compromise our values."
In summary, engaging with these insights will provide a robust foundation as we move forward. It offers a multi-faceted perspective essential for students, researchers, educators, and professionals who are navigating this complex and evolving landscape.
Original Research Articles
Summary of the Article
In our comprehensive examination of black box artificial intelligence, we analyze its architecture, applications, and implications. Key themes encompass the need for greater interpretability, the ethical ramifications of deploying such technology, and strategies for reliance on AI that achieve performance alongside transparency.
Author Contributions
This article draws on extensive interdisciplinary research, bringing together insights from computer science, ethics, and social sciences. Each contribution seeks to enhance understanding while advocating for responsible use of AI technologies, ensuring that conversations around black box models remain rooted in integrity and transparency.
Preamble to Black Box Artificial Intelligence
Understanding black box artificial intelligence is crucial in today’s rapidly evolving tech landscape. The term evokes a sense of mystery, hinting at the opaque nature of decision-making processes employed by such AI systems. The relevance of this topic extends beyond pure technology; it touches on ethics, trust, and societal impacts, making it a pivotal area of study for students, researchers, educators, and professionals alike.
Black box models primarily operate in ways that are not readily interpretable by humans. This lack of transparency can breed skepticism among users and stakeholders, heightening concerns about accountability and bias. Moreover, as AI continues to integrate into critical sectors like healthcare, finance, and transportation, the stakes only get higher. A misstep or misunderstood action could have significant repercussions.
The benefits of understanding black box AI cannot be overstated. This comprehension allows stakeholder’s a glimpse into the mechanisms at play, fostering confidence in technology. By diving into the characteristics, rise, applications, and ethical implications of these models, this article aims to illuminate the path forward. In doing so, we hope to highlight the delicate balance between harnessing powerful AI capabilities and maintaining a clear understanding of their operations.
Definition and Characteristics of Black Box Models
Black box models can be defined as algorithms that provide predictions or classifications without revealing the inner workings that lead to those outputs. Essentially, users see the results but not the rationale behind them. This lack of insight poses a challenge when it comes to trust; if one cannot understand how a decision was made, it becomes difficult to trust that decision.
Characteristics of black box models include complexity, non-linearity, and high dimensionality. These factors often contribute to their predictive power, yet make it almost impossible for humans to interpret how inputs translate into outputs. For example, deep learning models, like neural networks, can learn intricate patterns from vast datasets, yet their layered architecture obscures the path taken to reach a conclusion.
The Rise of Black Box AI in Recent Years
The last decade has witnessed a dramatic upsurge in the adoption of black box AI across various industries. Factors contributing to this trend include the explosion of data, advancements in computational power, and improvements in algorithms. As businesses scramble to make sense of the massive influx of information, they turn to powerful AI systems, often without fully grasping their complexities.
One notable instance is in the financial sector, where predictive analytics based on black box models have become a go-to for risk assessment and fraud detection. These systems snipe through mountains of transactions, spotting anomalies that human analysts might miss.
However, the reliance on black box systems is not without its pitfalls. As organizations increasingly lean on these AI methodologies, the potential for unintended consequences grows. Regulatory scrutiny has followed suit, pushing for greater transparency and accountability. The challenge now lies in finding ways to either demystify these models or develop new approaches that balance efficiency with comprehensibility.
Technological Foundations of Black Box AI
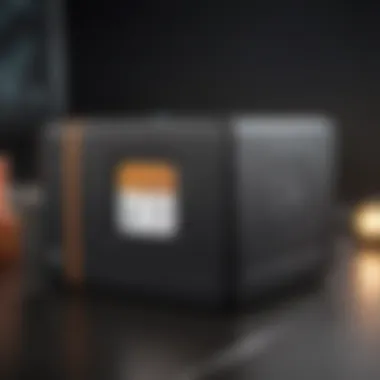
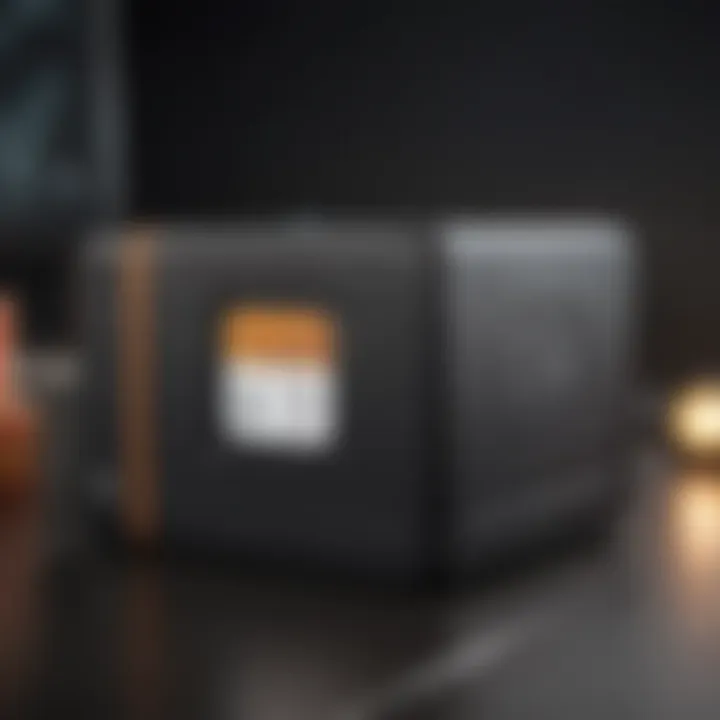
In the realm of artificial intelligence, understanding the technological foundations of black box AI models is crucial for grasping their complexities and appreciating their vast potential. Black box AI refers to systems where the inner workings are not transparent, making it challenging to interpret how inputs are transformed into outputs. Grasping the underlying technologies that empower these models is vital for ensuring that they function effectively, maintain reliability, and—importantly—achieve a semblance of accountability.
Machine Learning Algorithms and Their Complexities
Machine learning is the bedrock of black box AI. It comprises numerous algorithms that learn from data, adjust to patterns, and predict outcomes. Among them, deep learning models—especially neural networks—have gained prominence due to their ability to handle large datasets effectively. For instance, convolutional neural networks (CNN) are the linchpin behind image recognition technologies, interpreting a colossal amount of pixel data, yet their decision-making process remains opaque.
These algorithms exhibit complexities that can often escape even seasoned data scientists. Why? Because the intricate interrelations between nodes in a neural network create a web of decisions that is as convoluted as a spider's web. Furthermore, the training of such models involves hundreds of thousands, if not millions, of parameters, which makes it an uphill task to ascertain how specific features contribute to the final decision.
One pressing concern is the phenomenon known as overfitting. When a model learns the training data too well, it fails to generalize to new data. This can result in a deceptively high performance score during testing that does not translate into real-world applications. Thus, while machine learning algorithms provide advantageous predictive capabilities, they also introduce layers of complexity that can obscure understanding and interpretation.
Key Technologies Enabling Black Box Functionality
Several key technologies provide the structural integrity that underpins black box AI. These technologies not merely enhance model capability but also help in fostering the very black-box nature of these systems.
- Neural Networks: As mentioned, neural networks—particularly deep neural networks—form the backbone of many black box systems. Their layered architecture facilitates complex feature extraction but comes at the cost of interpretability.
- Big Data: The surge in available data from various sources empowers black box AI to learn from a richer context. The challenge is that integrating data from diverse streams can lead to unexpected correlations that misguide outcomes.
- Cloud Computing: The scalability of cloud resources allows large-scale model training and deployment, which furthers reliance on algorithms that are less interpretable due to their size and complexity.
- Transfer Learning: This technology enables pre-trained models to adapt to new tasks efficiently. While it enhances performance, it can muddy the waters regarding understanding which specific element of the original model contributes to success in a new context.
To wrap it up, the technologies that facilitate black box functionality present substantial benefits but also introduce significant challenges. The choice of algorithms, data handling techniques, and deploying methods intertwine to create systems whose inner workings may be less than transparent. As we progress in this domain, keeping a laser focus on these foundational aspects is imperative, as they define not only the performance but also the ethical implications of deploying such technologies in sensitive environments.
Applications of Black Box AI
The emergence of black box artificial intelligence has led to transformational changes across various sectors, making it an integral component of modern technological applications. Utilizing complex algorithms, these models have shown remarkable proficiency in areas where human decision-making is often constrained by time or cognitive biases. However, despite their prowess, the opaque nature of these systems raises pressing questions regarding their implications for transparency and accountability. Understanding the various applications of black box AI shines a light on both the potential benefits and inevitable concerns associated with its integration into critical domains.
Use in Healthcare and Diagnostics
In healthcare, black box AI has carved out a significant niche, particularly in diagnostics and treatment recommendations. For instance, algorithms that analyze medical images such as X-rays or MRIs can identify anomalies with astonishing accuracy, often on par with, or even exceeding, human radiologists. This capability not only increases the speed of diagnosis but also improves patient outcomes by facilitating early detection of diseases.
In addition, predictive models are being used to customize treatment plans based on individual patient data. This shift from a one-size-fits-all approach to personalized medicine exemplifies how black box AI can enhance treatment effectiveness.
Still, the reliance on these systems isn’t without challenges. The lack of transparency, particularly in understanding how decisions are made, can be alarming for both healthcare professionals and patients. The ethical implications of accountability in case of misdiagnosis must be addressed.
Role in Financial Services and Risk Assessment
In the financial realm, black box models wield considerable influence in risk assessment and fraud detection. Institutions like banks increasingly depend on these algorithms to evaluate creditworthiness, which allows for quicker loan approvals while also reducing potential risk. For example, algorithms can sift through a vast array of data points, including social media activity and transaction histories, to forecast an applicant's financial behavior more accurately than traditional methods.
Fraud detection systems also benefit immensely. By continuously learning from transaction data, these models can identify unusual patterns, flagging them for further investigation. The ability to adapt in real-time is crucial, given the sophistication of fraudulent activities today. However, as with healthcare, there exists a downside. The opacity of these systems raises concerns about bias and fairness in credit assessments, which could perpetuate systemic inequalities.
Deployment in Autonomous Systems and Robotics
The rise of autonomous systems and robotics has demonstrated the far-reaching promise of black box AI. From self-driving vehicles to drones used in deliveries or surveillance, these technologies are grounded in decision-making processes often shielded from user scrutiny. Self-driving cars, for instance, utilize cameras and sensors to interpret their surroundings, making split-second decisions based on complex datasets. Here, the black box nature can present challenges, especially concerning safety and accountability—who is liable if an autonomous vehicle malfunctions?
Furthermore, the integration of AI into manufacturing robots is revolutionizing productivity. Robots can optimize production lines through data-driven adjustments that enhance efficiency and minimize costs. Yet, relying exclusively on black box insights can lead to overconfidence and a lack of human oversight.
Thus, while the applications of black box AI span critical industries and hold immense potential, they also underscore the essential conversation about the ethical ramifications tied to these technologies.
Ethical Implications of Black Box AI
The rise of black box artificial intelligence raises significant questions about its ethical dimensions. Understanding these implications is crucial as society becomes increasingly reliant on algorithms that operate in opaque ways. The moral challenges inherent in black box models can have far-reaching effects on individuals, organizations, and broader social structures. This section aims to dissect some of the key ethical considerations surrounding black box AI.
Challenges to Transparency and Accountability
Transparency is the bedrock of trust. Yet, black box AI models often operate without clear visibility into how they arrive at particular decisions. The mechanics are often shrouded in mathematical complexities and proprietary details. As a result, it becomes exceedingly difficult to hold these systems accountable.
When an algorithm makes a mistake—say, misidentifying a person in a security context—who is responsible? The developers? The businesses applying the technology? The absence of accountability structures can lead to a blame game, where no one takes ownership of the outcomes, good or bad.
Consider a real-world example from the criminal justice system. Risk assessment tools, like those used in sentencing, utilize black box AI to predict recidivism. If the algorithm leads to unjust sentencing, victims and judges alike are left without clear recourse. To combat this challenge, there is a growing call for models that ensure more evaluative transparency, helping to illuminate the decision-making process.
Bias and Fairness in Decision-Making
Bias in AI is a hot topic, and black box algorithms can inadvertently reinforce social inequalities. If a dataset reflects historical biases, the AI’s decisions will likely mirror those patterns. This perpetuates cycles of discrimination, particularly in sensitive areas such as hiring or lending.
For instance, a study focused on employment algorithms revealed troubling patterns where minority candidates were systematically underrepresented in selection processes. Decisions that should be based on merit instead hinge on biases baked into the training data, leading to outcomes that are both unfair and discriminatory. As a response, researchers advocate for strategies that emphasize fairness, aiming to create data sets that minimize bias.
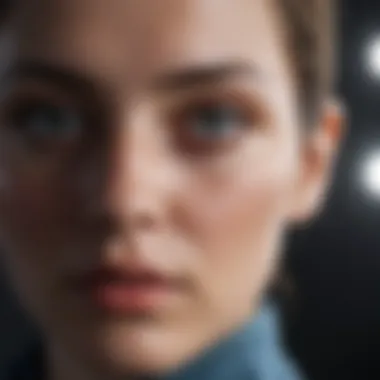
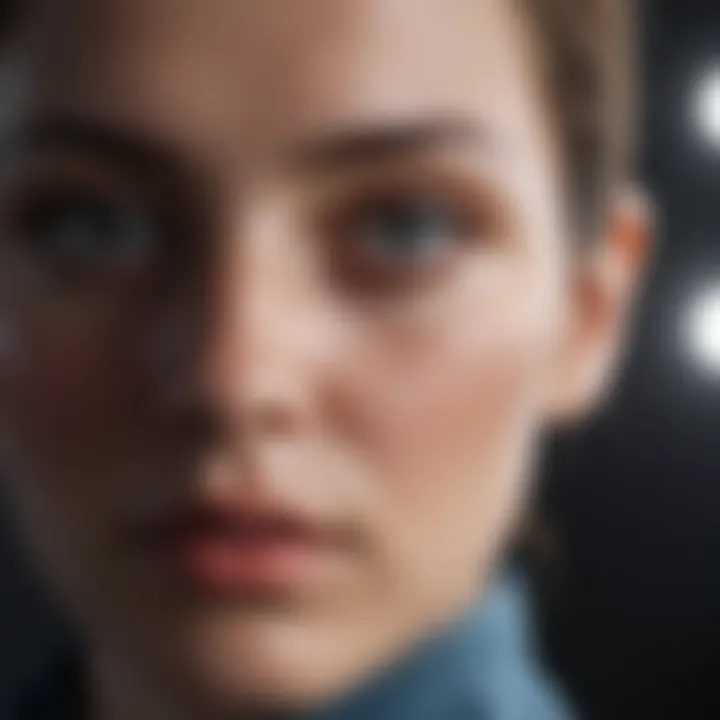
"An AI without fairness and equality is like a ship without a compass; it may lead to uncertain destinations."
Consequences for Privacy and Data Protection
Black box AI often involves massive troves of personal information. Users may not realize just how much scrutiny they are under. Data protection laws like GDPR impose strict regulations on personal data usage. However, the complexity of black box AI means compliance becomes difficult. If the AI can’t explain how it uses data or where it goes, ensuring that it does so ethically becomes a game of cat and mouse.
Consider the implications when users unknowingly allow their data to be part of a model training process, only for that data to be used in ways they never consented to. This breach of privacy can lead to trust erosion and hesitance to engage with technology deemed necessary in modern life. To offset this, companies are increasingly needing to embrace privacy-by-design principles, ensuring data protection mechanisms are baked into the AI’s architecture from the outset.
Strategies for Enhancing Interpretability
In the ever-expanding landscape of black box artificial intelligence, the quest for interpretability stands as a significant necessity. As AI systems continue to grow in complexity, understanding their operations becomes increasingly vital. These strategies not only serve to demystify the seemingly opaque decision-making processes of AI but also play a critical role in building trust with end-users.
Enhanced interpretability provides numerous benefits, such as identifying biases, fostering accountability, and ensuring compliance with ethical standards. In a world where AI influences everything from healthcare diagnostics to financial assessments, it is crucial for stakeholders to have a clear view of how these tools operate. Without this clarity, users may find themselves at the mercy of algorithms with little recourse to challenge their outcomes.
Model-agnostic Approaches and Techniques
When focusing on interpretability, model-agnostic approaches emerge as a key player in the domain of black box AI. These techniques aim to provide explanations that are applicable across various types of models, rather than being limited to specific algorithms.
- LIME (Local Interpretable Model-agnostic Explanations): This technique works by perturbing the input data and observing the resulting changes in predictions. It generates local approximations around specific instances to reveal how different features influence outcomes.
- SHAP (SHapley Additive exPlanations): Drawing from cooperative game theory, SHAP values offer a way to attribute the impact of each feature on the predictions made by the model. It ensures that explanations are consistent and adheres to logical fairness considerations.
These methods provide stakeholders with insights into how features contribute to decision-making processes, promoting a better understanding of black box systems. They can help in assessing why a model arrived at a specific result, enabling organizations to verify if the AI operates within ethical norms.
Towards Explainable AI Frameworks
Efforts towards creating explainable AI frameworks represent the next step in making black box AI systems more user-friendly and transparent. Such frameworks prioritize designing AI that is inherently interpretable from the outset.
- Incorporating Visual Explanations: Integrating intuitive visual representations of how features interact can illuminate AI's reasoning, making it accessible.
- Leveraging Natural Language Processing: By utilizing natural language techniques, AI can offer explanations in layperson's language rather than technical jargon, fostering better comprehension among diverse user groups.
- User-Centric Design: Focusing on users’ needs and providing tailored explanations can significantly enhance the interpretability of AI systems.
Ultimately, progressing towards explainable AI frameworks is not merely about sharpening interpretability but also about aligning AI’s functionality with human values. As these frameworks develop, the interaction between human and AI can transform dramatically, leading to more informed decisions and a greater assurance in the technologies that pervade our lives.
"The best way to predict the future is to invent it." - Alan Kay
The journey towards enhancing interpretability in black box AI is paramount, as it paves the way for a future where technology and human values can coalesce to create trustworthy systems.
Case Studies of Black Box AI in Action
The landscape of black box AI has been rapidly transforming industries and redefining standard practices across various sectors. Examining real-world case studies provides invaluable insights into the operational dynamics of these complex systems. Understanding both the successes and failures associated with black box AI enhances our understanding of its potential and pitfalls, guiding future developments in technology.
Successful Implementations in Industry
One of the significant aspects of black box AI lies in its widespread adoption across diverse industries, yielding substantial benefits. For instance, companies such as Google and Amazon have harnessed black box algorithms to optimize their recommendation systems. These systems analyze user preferences and behaviors, thereby improving customer experience. Millions of users rely on these recommendations daily, resulting in increased sales and customer loyalty.
In the healthcare sector, IBM's Watson has demonstrated noteworthy capabilities as a diagnostic tool. By analyzing vast amounts of medical data and treatment options, Watson provides recommendations that assist doctors in making informed decisions. Such a system enhances the accuracy of diagnoses while enabling healthcare professionals to access information that was once too cumbersome to sift through manually. Another case in point is the use of black box AI in predicting patient readmissions, which has enabled hospitals to implement proactive intervention protocols, ultimately improving patient outcomes.
These examples underline the transformative potential of black box AI when implemented thoughtfully and responsibly. It highlights that while these models may lack transparency, their ability to process and analyze large datasets efficiently is unmatched. The significant positive impact on operational efficiency and decision-making across industries cannot be overstated.
Failures and Lessons Learned
However, not all implementations of black box AI have gone smoothly. A prominent instance is the case of COMPAS—a risk assessment tool used in the criminal justice system. Designed to evaluate the likelihood of a defendant re-offending, COMPAS came under scrutiny for its opaque decision-making process and perceived biases against certain demographic groups. Critics highlighted that the lack of transparency in how it derived its assessments led to ethical concerns regarding fairness and accountability.
"Learning from failures is just as important as celebrating successes; controversy surrounding black box systems emphasizes the necessity for transparency and ethical decision-making."
Another noteworthy example involved an AI system deployed by Amazon for hiring. This system analyzed resumes to identify the most qualified candidates. However, it became evident that the model tended to favor male candidates, reflecting biases that were present in the data it learned from. Consequently, Amazon scrapped the project altogether, realizing that reliance on such black box technologies without proper checks can lead to discriminatory practices.
These cases illuminate critical lessons for organizations seeking to implement black box AI responsibly. First, transparency should be approached as a non-negotiable condition. If stakeholders do not understand how decisions are made, eroded trust and accountability inevitably follow. Additionally, ethical considerations around biases inherent in the training data must be rigorously addressed.
In essence, the journey of integrating black box AI into practical applications reveals a double-edged sword. It showcases remarkable capabilities but necessitates a confluence of ethical reasoning, transparency, and vigilant assessment to prevent missteps that can undermine the technology's potential.
Future Directions for Black Box AI
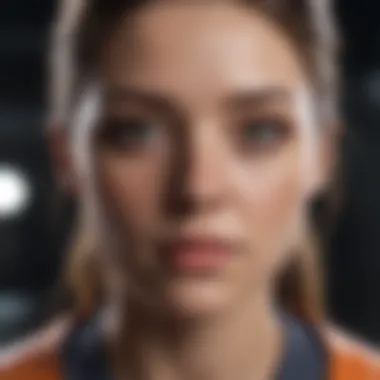
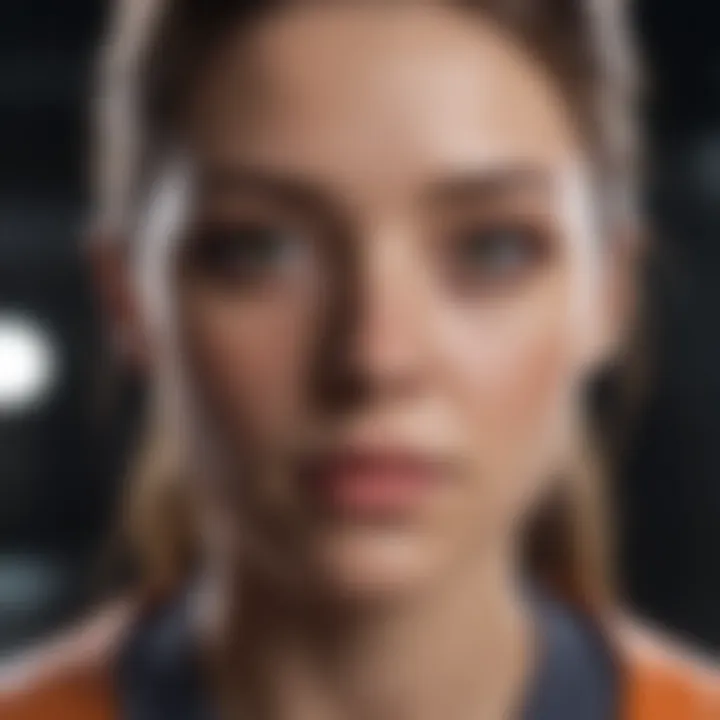
The future of black box artificial intelligence holds enormous potential, yet it is fraught with challenges that demand robust dialogue and action across multiple disciplines. As we look ahead, it’s crucial to understand how emerging trends and regulatory frameworks will shape the way black box models evolve.
Trends Shaping the Evolution of AI Models
In the rapidly changing tech landscape, several trends are crystallizing and paving the way for the next generation of AI models. Here are a few noteworthy developments:
- Increased Demand for Explainability: Organizations are beginning to realize that understanding how AI reaches its conclusions isn’t just advantageous, it’s necessary. Models that can elucidate their decision-making processes are becoming a competitive advantage.
- Focus on Integration with Human Skills: Rather than standing alone, future AI models will likely augment human abilities. This means designing systems that not only perform tasks but also collaborate intelligently with human operators.
- Interdisciplinary Research: Collaboration between fields such as computer science, sociology, and philosophy will lead to more comprehensive frameworks for developing AI that respects ethical guidelines while still pushing technological boundaries.
"The question isn't whether black box AI will progress, but how we will steer its transformation to align with societal needs."
Each of these trends demonstrates a conscious shift towards creating AI systems that prioritize transparency and reliability, responding to stakeholders' calls for ethical and social responsibility.
The Role of Regulation and Policy in AI Development
As society becomes increasingly entwined with technology, the role of regulation can’t be overlooked. Policymakers will play a critical part in shaping the future of black box AI by providing frameworks that support innovation while also protecting users. Some considerations include:
- Establishing Standards for Transparency: Governments may need to establish guidelines that require companies to disclose key information about how their models work. This could help mitigate risks associated with automated decision-making.
- Fostering Research on Ethical AI: Public investment in rigorous research focused on the ethics of AI could develop methodologies that lead to more responsible AI use, encouraging a culture of responsibility among developers.
- Promoting Accountability: Regulations could enforce accountability measures for organizations deploying black box AI, ensuring that there are recourses available for those affected by AI decisions.
In tandem with these measures, a thoughtful approach to education will be essential—teaching developers about the implications of their work, as well as guiding users in understanding AI systems will create an ecosystem where black box AI can thrive responsibly.
As we navigate this future, it remains imperative that technologists, ethicists, and policymakers engage in ongoing conversations, balancing innovation with an unwavering commitment to ethical standards. The complexities of black box AI are not just a technical challenge; they necessitate a multifaceted response that honors human values.
Interdisciplinary Insights
In today's rapidly evolving landscape of artificial intelligence, the interplay between various fields of study cannot be overlooked. Interdisciplinary insights serve as the cornerstone for a deeper understanding of black box AI. This dialogue among different disciplines enriches the conversation by merging technical expertise with ethical considerations, social implications, and real-world applicability. By harnessing diverse perspectives, we can tackle the profound challenges posed by black box AI head-on.
Collaboration Between Computer Science and Ethical Philosophy
The junction where computer science meets ethical philosophy is pivotal in interrogating the moral landscape of AI. While computer scientists focus on algorithms, optimization, and functionality, ethical philosophers ask critical questions about the ramifications of these technologies on society. For instance, when a black box model makes a life-altering decision—be it for credit lending or medical diagnostics—it is imperative to evaluate whether these decisions uphold fairness and justice. This collaboration transcends traditional boundaries, fostering an environment that nurtures responsible AI development.
- Key Benefits of Collaboration:
- Promotes the design of ethical frameworks that influence AI development.
- Encourages transparency and accountability in AI applications.
- Stimulates regulatory discussions surrounding AI usage.
Embracing this interdisciplinary approach allows for a richer grasp of the ethical nuances surrounding AI technology. Together, professionals from both fields can draft guidelines that ensure algorithms reflect societal values and priorities, rather than merely being technical feats devoid of human consideration.
Integration of Social Sciences in AI Research
The addition of social sciences into the AI research narrative opens doors to insights that purely technical examination might miss. Understanding user behavior, societal norms, and cultural contexts is crucial for the responsible deployment of black box AI systems. For example, how users engage with AI-driven tools varies dramatically across demographics, influencing how we assess both risks and benefits.
Incorporating social science methodologies—like surveys, interviews, and focus groups—helps to illuminate these variables. This holistic view can:
- Identify Biases: Uncover underlying biases that may affect algorithm performance.
- Enhance User Trust: Cultivate user trust through transparent development processes.
- Shape Policy Decisions: Inform policymakers about the societal impact of AI.
Accordingly, fostering the integration of social sciences in AI research not only improves the technological aspect but also fortifies the societal fabric within which these systems operate.
"AI is not merely a collection of algorithms; it reflects the cultural and ethical frameworks of the society that creates it."
By bridging the gap between disciplines, we pave the way for innovations that are not only groundbreaking but also fair and just.
Epilogue
Summary of Key Findings and Insights
In our exploration of black box artificial intelligence, several key takeaways emerge. Firstly, the phenomenon of black box AI is not simply a technical challenge but a multifaceted issue that intertwines technology with ethics. As it stands, these models, widely used across various industries, often act behind the scenes, making decisions that affect our lives without clear transparency. The complexity of algorithms contributes to this opacity, raising significant concerns regarding accountability and trust. Moreover, the applications range from healthcare, where diagnostic errors can have severe consequences, to financial services, where bias can lead to unfair treatment of clients.
Another important aspect we discovered is the growing need for collaboration across disciplines. The merging of computer science with ethical philosophy and social sciences is not just beneficial but paramount in creating frameworks that enhance understanding while addressing ethical dilemmas. By incorporating diverse perspectives, we can begin to forge pathways toward more interpretable models that do not sacrifice performance for clarity.
"Transparency in AI isn’t merely a good practice; it is an essential element for ethical technology deployment."
Reflections on the Balance of Complexity and Clarity
The realm of black box AI requires a nuanced approach to find the delicate balance between complexity and clarity. On one hand, sophisticated models yield powerful insights and capabilities; on the other, their intricate nature can significantly obscure understanding. This dichotomy puts practitioners in a challenging position: how to leverage advanced models while ensuring that stakeholders remain informed and empowered.
To achieve this balance, numerous recommendations emerge. For instance, adopting model-agnostic strategies allows for greater interpretability, fostering trust without dismantling the predictive strengths of these systems. Moreover, explainable AI frameworks act as bridges, connecting deep learning with comprehensible outcomes.
In navigating this complex landscape, educators and policymakers must prioritize tailored strategies that promote transparency, not just in technology but also in its implications on society. Ultimately, guiding efforts towards a clear articulation of AI processes can help demystify the technology, paving the way for responsible use in our increasingly automated world.