Understanding Synchrony RFS: Key Concepts and Applications
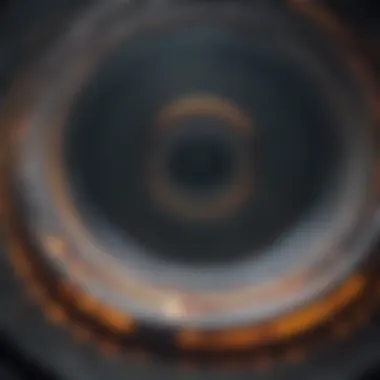
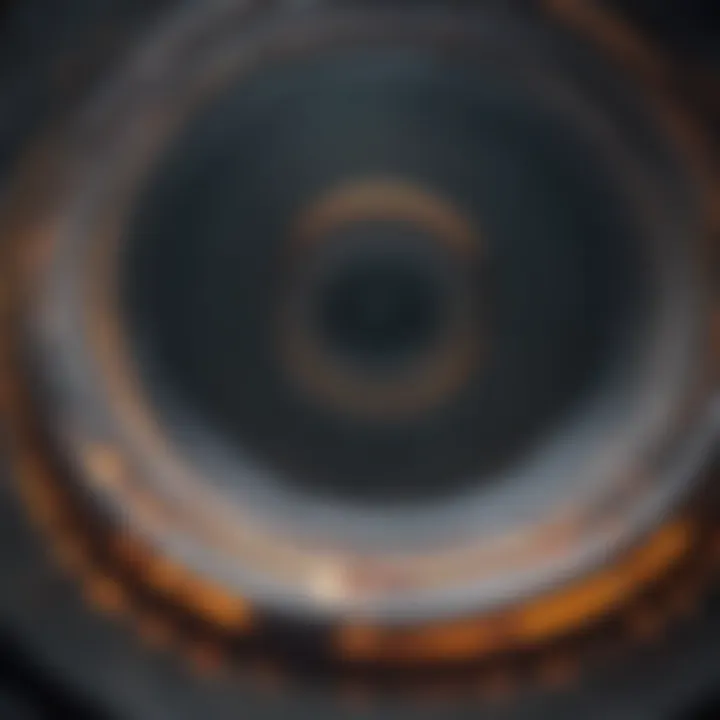
Intro
Synchrony RFS represents a significant advancement in the fields of research and technology. Understanding the core concepts, real-world applications, and future research possibilities of this system is crucial for professionals across various scientific disciplines. As the demand for innovative methodologies grows in biology, chemistry, and beyond, Synchrony RFS stands out for its potential to transform how research is conducted and applied. This section aims to provide an essential overview of Synchrony RFS, laying the groundwork for a deeper exploration of its implications and applications.
Research Highlights
Overview of Key Findings
The research surrounding Synchrony RFS has unveiled several important findings:
- Efficiency: Synchrony RFS enhances data processing speeds, crucial for time-sensitive studies.
- Versatility: The system is applicable across different scientific fields, showcasing its adaptability.
- Integration: It allows for the synthesis of data from various sources, improving the robustness of research outcomes.
These findings demonstrate the system's promise as a valuable tool in scientific inquiry.
Significance of the Research
Understanding Synchrony RFS contributes to a broader comprehension of modern research techniques. Its significance lies in:
- Bridging Gaps: Synchrony RFS may bridge complex data entanglements in research, enabling clearer insights.
- Enhancing Collaboration: By fostering collaboration between disciplines, it can lead to innovative solutions to existing problems.
- Driving Innovation: As new methodologies emerge, Synchrony RFS ensures that research remains relevant and impactful.
"With its capacity to adapt across fields, Synchrony RFS is positioned to change the landscape of scientific exploration significantly."
As this article progresses, readers will delve deeper into the technical specifications and methodologies integral to Synchrony RFS. This investigation will further illuminate its efficacy and potential limitations in various domains.
Prolusion to Synchrony RFS
The exploration of Synchrony RFS serves a critical role in modern scientific studies. Understanding its principles can lead to significant advancements across various fields, from biology to chemistry and physics. The importance of Synchrony RFS lies in its ability to provide a framework for data synchronization and analysis in real-time, enhancing the efficacy of research methods.
In this section, we will dive into granular details about its definition, core concepts, and historical context. These elements form the bedrock of every practical application and potential future directions in research. By comprehending the fundamentals of Synchrony RFS, researchers can identify its benefits and consider limitations that may arise.
Definition and Core Concepts
Synchrony RFS, or Synchrony Real-time Feedback Systems, refers to the methodologies and technologies that enable the instantaneous synchronization and analysis of data across multiple domains. At its core, Synchrony RFS is characterized by the following key concepts:
- Real-time Data Processing: The ability to process data as it is generated helps researchers instantly react to findings and phenomena observed during experiments.
- Feedback Mechanisms: These systems incorporate feedback loops that refine data collection and analysis, ensuring continuous improvement of results.
- Synchronization Across Platforms: Integrating various sources and formats of data allows for comprehensive analysis that can draw from multiple scientific disciplines.
Understanding these concepts is fundamental when applying Synchrony RFS in real-world scenarios, ensuring researchers can leverage its full capability while remaining aware of any inherent constraints.
Historical Background and Development
The historical evolution of Synchrony RFS provides insight into its present-day applications and challenges. Initially, data collection methods were mostly manual and time-consuming, limiting the scope of research. However, advances in technology have permitted the development of automated systems that integrate real-time data syncing.
Milestones in the development of Synchrony RFS include:
- The Advent of Computer Technologies (1970s): Early computer systems laid the groundwork for automated data collection and analysis, leading to the formation of the first synchronization methods.
- Emergence of Real-Time Feedback Systems (1980s-1990s): Researchers started developing feedback systems that could offer immediate insights during experimentation, significantly improving research efficiency.
- Integration with Modern Technologies (2000s-present): Recent advancements, such as cloud computing and machine learning, have transformed Synchrony RFS, presenting opportunities for its application across more complex experiments and larger data sets.
By studying its history, we can better appreciate Synchrony RFS’s transformative role in scientific inquiry. This understanding is crucial as we look ahead to future research avenues and the innovative approaches that may arise.
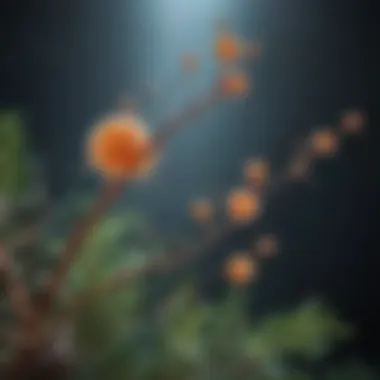
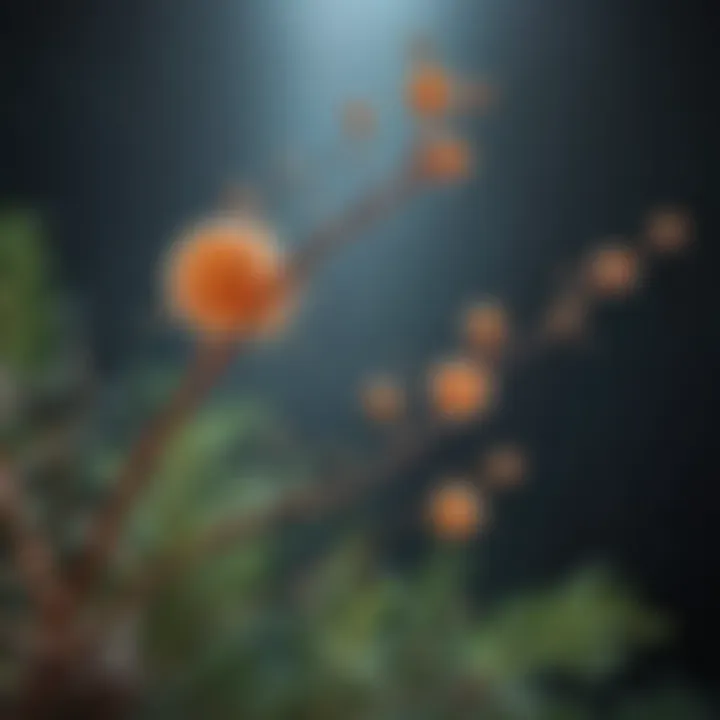
Technical Overview of Synchrony RFS
The technical overview of Synchrony RFS is essential for a profound comprehension of its role and capabilities in diverse scientific realms. Understanding this aspect not only elucidates how Synchrony RFS operates but also provides insights into its practical implications. By focusing on system architecture and the key components that contribute to the functionality of Synchrony RFS, we can appreciate its value in research and applications.
System Architecture
The system architecture of Synchrony RFS serves as the backbone supporting its various functionalities. Typically, it comprises multiple layers, each designed to address specific tasks. The layers work synergistically to ensure reliable synchronization of data across different systems. Here are some core features of this architecture:
- Modularity: Different components can be updated or replaced without overhauling the entire system.
- Scalability: As data demands grow, the system can adapt by increasing resources.
- Interoperability: Facilitates communication between diverse platforms and devices, enhancing its usability in multi-disciplinary contexts.
To illustrate, consider a biological research setting. The Synchrony RFS system architecture may integrate data from various laboratory instruments, ensuring coherent data flow and synchronization across the devices. This minimizes errors and enhances the accuracy of experimental results.
Key Components and Functionality
The functionality of Synchrony RFS is driven by several key components, each playing a specific role in the data synchronization process. These components are vital to ensuring that data remains coherent and accessible across platforms.
- Data Aggregators: These components collect data from various sources and prepare it for synchronization. They filter and organize the data to maintain quality and relevance.
- Synchronization Algorithms: These algorithms dictate how data is aligned and transmitted. Efficient algorithms are crucial for minimizing latency and ensuring that all systems operate on a consistent dataset.
- Feedback Mechanisms: Integrate checks that assess synchronization quality, allowing adjustments as necessary to respond to real-time conditions.
"By adopting a robust system architecture and integrating advanced components, Synchrony RFS enhances experimental capabilities across scientific fields."
Furthermore, these key components contribute significantly to the overall performance metrics. They enable researchers to track changes instantaneously and make informed decisions based on synchronized data. Their cohesive functionality dictates the system's efficiency and reliability, ultimately driving innovation and discovery.
Ultimately, the technical overview of Synchrony RFS augments the understanding of its intricate workings. This awareness allows scholars and professionals to leverage its capabilities effectively, aligning with their research needs and advancing scientific inquiry.
Methodologies Involved in Synchrony RFS
Understanding the methodologies involved in Synchrony RFS is crucial for grasping its broader implications in various scientific fields. This section elaborates on the specific techniques employed to achieve effective data synchronization and highlights the performance metrics used to evaluate these methods. By examining these elements, readers can appreciate the complexities and benefits inherent in Synchrony RFS, paving the way for its future applications.
Data Synchronization Techniques
Data synchronization in the context of Synchrony RFS is a multi-faceted issue, addressing the timely and accurate sharing of data between systems. Multiple techniques are employed within this framework, each contributing unique advantages. Methods can be broken down into several categories:
- Real-time Synchronization: This technique allows data to be updated concurrently across multiple locations. For example, live data streams ensure that alterations in one database are mirrored across others almost instantaneously.
- Batch Synchronization: Unlike real-time techniques, this method involves accumulating data changes over a specified period and transmitting them as a grouped package. This could be more efficient when dealing with large volumes of data, minimizing the load on network resources.
- Conflict Resolution Strategies: When working with distributed data sources, inconsistencies can arise. Strategies such as 'last write wins' or vector timestamps help to determine which data version should take precedence.
- Data Compression Techniques: These optimize bandwidth and enhance the speed of synchronization processes. Compression algorithms reduce the size of datasets before transmission, which is essential for networks with limited capacity.
Each of these techniques has its own set of strengths and weaknesses, making them suitable for different scenarios in data management. Selecting the appropriate synchronization strategy is critical for organizations looking to implement Synchrony RFS effectively.
Performance Metrics and Evaluation
Evaluating the effectiveness of data synchronization techniques is essential in determining their applicability in real-world situations. A few key performance metrics include:
- Latency: This measures the delay between when a change occurs and when it is reflected across systems. Lower latency indicates a more efficient synchronization process.
- Throughput: This metric looks at the amount of data successfully synchronized over a specific time frame. A higher throughput suggests that the system can handle larger data volumes without degradation of performance.
- Error Rate: Tracking the frequency of synchronization errors is vital for assessing the reliability of the system. A lower error rate signifies a more robust methodology.
- Scalability: The ability to scale the synchronization method as data volume increases reflects the long-term viability of the approach. Systems that gracefully manage growth tend to be more appealing for ongoing implementation.
- Resource Utilization: Monitoring how much computational power or bandwidth the synchronization process consumes helps in understanding its impact on existing systems. Low resource usage while maintaining performance is highly desirable.
These performance metrics are instrumental in guiding the further development and refinement of Synchrony RFS methodologies, ensuring they meet the evolving needs of various scientific disciplines.
The methodologies utilized in Synchrony RFS are not only fundamental but also transformative, opening pathways to greater efficiency and accuracy in data management.
Applications of Synchrony RFS
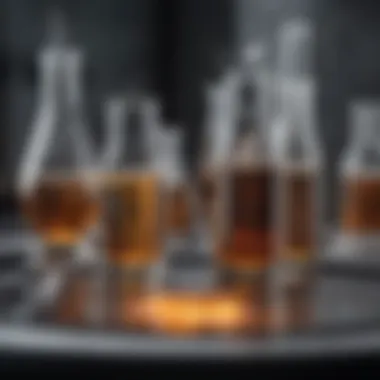
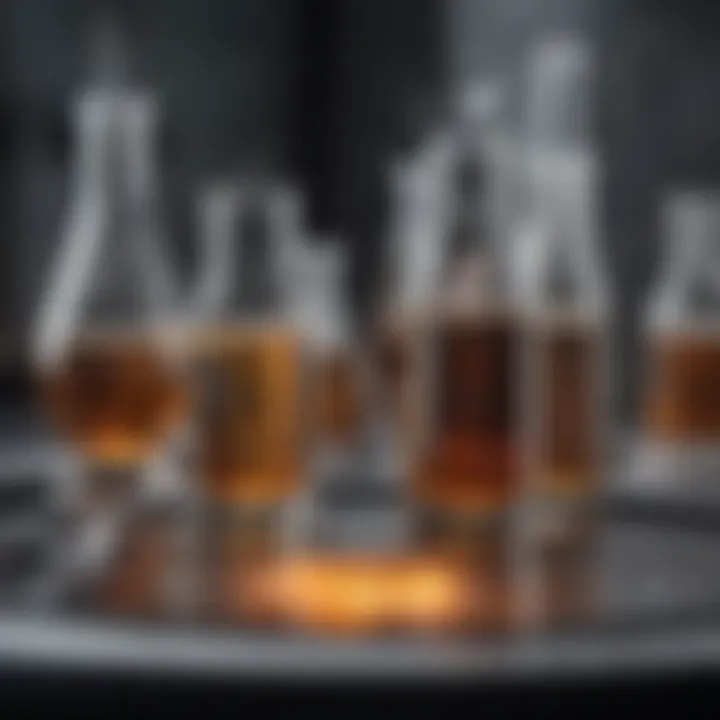
The applications of Synchrony RFS represent a significant aspect of its broader impact across various scientific domains. This section examines not only the applications themselves but also their importance and implications. Synchrony RFS finds its utility in areas that require precise timing and coordination, which can enhance measurement accuracy and facilitate better data integration. By exploring its applications, one can appreciate the flexibility and robustness of Synchrony RFS in both established and emerging fields.
Biological Systems and Processes
In biological research, Synchrony RFS plays a crucial role in understanding complex systems. The synchronization of data from multiple biological processes can lead to breakthroughs in areas like genomics and systems biology. For example, when studying gene expression, researchers can utilize Synchrony RFS to align temporal changes in gene activity across various conditions. This is invaluable for elucidating regulatory networks and identifying potential biomarkers.
Moreover, in physiological monitoring, Synchrony RFS can synchronize data streams from devices used in wearable technology. These devices collect continuous physiological metrics. When synchronized properly, the data provides more coherent insights into an individual’s health, facilitating early detection of anomalies.
Chemical Reaction Monitoring
The real-time monitoring of chemical reactions also benefits from Synchrony RFS. It allows for the acquisition and synchronization of data from different sensors during chemical experiments. For instance, when reactions occur, parameters such as temperature, pressure, and concentration evolve over time. Synchrony RFS ensures that data collected from various sources reflect these changes in a coordinated manner. This synchronized data leads to an improved understanding of kinetic processes in chemistry, allowing chemists to optimize conditions for desired reactions.
In addition, applications extend to the pharmaceutical industry where meticulous monitoring of reactions is imperative. Utilizing Synchrony RFS can enhance drug development, allowing for more efficient screenings of compounds through synchronized testing protocols.
Cross-disciplinary Uses in Physics
Traditionally, Synchrony RFS has shown potential in the realm of physics as well. In experimental physics, understanding phenomena such as particle collisions requires precise timing. Synchrony RFS can integrate data from various detectors. This level of synchronization permits researchers to reconstruct events occurring at minuscule timescales, revealing insights that would otherwise remain obscured in unsynchronized data.
In geophysical studies, this technology can synchronize data from seismic sensors during earthquake monitoring. When seismic events occur, multiple sensors record data. Using Synchrony RFS enhances the resolution of seismic data, aiding in more accurate modeling of seismic activities.
Synchronization in research not only enhances the quality of data but also enables collaborative efforts across disciplines, enriching the scientific inquiry process.
Case Studies of Synchrony RFS in Action
Understanding the real-world application of Synchrony RFS (Rapid Feedback Systems) is fundamental for grasping its role in diverse scientific contexts. Case studies enable us to evaluate practical implementations, revealing both the efficacy and any obstacles faced during adoption. By examining specific uses, we can extract lessons and insights that drive future innovations.
Real-world Applications in Research
The application of Synchrony RFS spans a variety of research fields, each with unique demands and expectations. For instance, in the realm of biomedical research, Synchrony RFS has been instrumental in developing real-time monitoring systems for various biological processes. Researchers can utilize this technology to observe cellular activities as they occur, allowing for more dynamic and immediate feedback. This can lead to more accurate hypotheses and more timely adjustments in experimental designs.
Moreover, in environmental science, Synchrony RFS supports ongoing assessments of ecosystem changes. By implementing this system, scientists can synchronize data from multiple sources, including sensors deployed at different locations. This real-time data collection facilitates better understanding of factors influencing climate change and habitat destruction. Consequently, decisions made based on these insights can be more informed and effective.
Success Stories and Outcomes
The success stories surrounding Synchrony RFS are numerous and varied. A notable example involves a cross-disciplinary project combining chemistry and biology. Researchers successfully monitored dynamic interactions between different drug compounds and biological tissues. The ability to receive immediate feedback on reactions allowed them to modify compounds on the fly, enhancing the efficacy of drug development.
Another exemplary success story can be attributed to a study in physics that used Synchrony RFS for collating and analyzing big data from particle collisions. The system’s capability to synchronize data points in real-time improved the accuracy of particle detection and analysis, leading to significant breakthroughs in understanding fundamental particles.
In summary, these case studies not only illustrate the versatility and importance of Synchrony RFS but also highlight its direct impact on driving innovation in research.
"The real power of Synchrony RFS lies in its ability to transform raw data into actionable insights in real-time, facilitating innovation across disciplines."
Through these case studies, we see that to leverage Synchrony RFS effectively, researchers must navigate both the technical capabilities and the specific contexts in which they operate. This dual consideration is essential for overcoming challenges and maximizing the potential of Synchrony RFS.
Challenges and Limitations
Understanding the challenges and limitations associated with Synchrony RFS is crucial for researchers and practitioners in the field. Addressing these aspects allows for informed decision-making and realistic expectations regarding the utility and effectiveness of this technology. Recognizing potential drawbacks can guide future improvements and innovations.
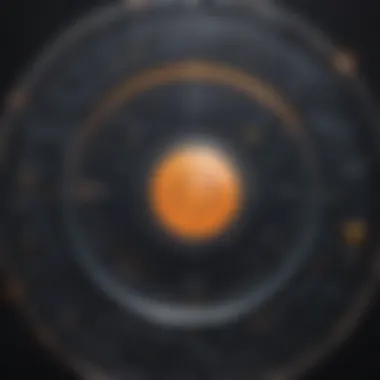
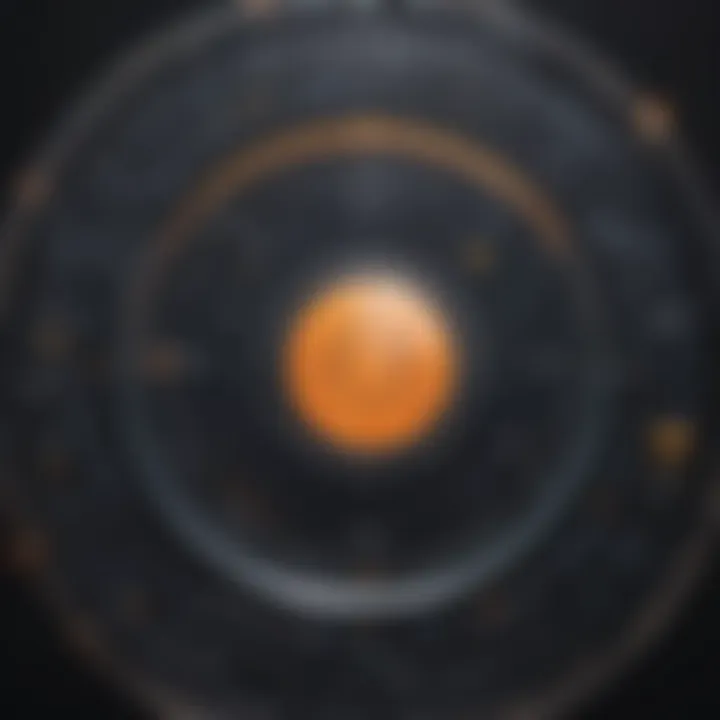
Technical Constraints
One of the primary challenges of Synchrony RFS lies in its technical constraints. These limitations can stem from several factors:
- Scalability: As the amount of data increases, maintaining synchronization across multiple data sources can become complex. Systems may face latency issues during high-volume operations.
- Latency and Reliability: Real-time data processing often requires minimal latency. Any delays can hinder results, especially in fields like biology and chemistry where time-sensitive data is critical.
- Compatibility: Integration with existing infrastructure may present difficulties. Legacy systems may lack the capacity or flexibility required to effectively run Synchrony RFS.
Researchers continue to investigate ways to overcome these drawbacks. This includes improving algorithms and hardware capabilities.
Issues in Practical Implementation
Implementing Synchrony RFS in real-world scenarios brings its own set of challenges:
- User Training and Expertise: Professionals using Synchrony RFS must have a certain level of expertise. The complexity of the technology can make it difficult for those without specialized training to utilize it effectively.
- Resource Allocation: Adequate resources, both in terms of technology and personnel, must be available. Budget constraints can limit the ability of organizations to fully leverage Synchrony RFS.
- Maintenance and Support: Ongoing maintenance is essential to ensure that the system operates smoothly over time. This requires not only regular updates but also technical support to resolve issues as they arise.
Addressing these implementation issues is vital. Without proper strategies, the advantages of Synchrony RFS may not be realized in practice.
In summary, the challenges and limitations of Synchrony RFS are significant. They require ongoing attention as the technology evolves. By identifying these technical constraints and practical issues, stakeholders can develop better models and frameworks for future applications.
Future Directions in Synchrony RFS Research
The exploration of future directions in Synchrony RFS research is crucial for the ongoing evolution of this field. As scientific inquiry shifts towards more interdisciplinary and integrative approaches, the ability to adapt Synchrony RFS to diverse applications presents a significant opportunity. One of the most salient aspects is the integration of advanced technologies, which can help streamline processes and enhance data accuracy. Understanding these future directions can benefit researchers and professionals aiming to leverage Synchrony RFS in their respective domains.
Innovative Approaches and Technologies
Innovative approaches in Synchrony RFS encompass a variety of emerging technologies. These advancements aim to improve synchronization accuracy and processing speed. For instance, the application of machine learning algorithms can enable systems to recognize patterns in synchronization data more efficiently. By employing tools like Python's TensorFlow or Scikit-learn, researchers can develop predictive models that optimize performance.
Another significant trend is the incorporation of Internet of Things (IoT) technologies. IoT devices can gather data in real-time from biological or chemical processes, allowing for dynamic updates within Synchrony RFS systems. This integration leads to smarter systems that can react instantaneously to changes in their environment.
Potential improvements also lie in the area of cloud computing. Centralized data storage and processing can make data more accessible and facilitate collaboration across different research groups. This capability allows for enhanced data sharing and pooling of resources, which are vital for large-scale studies.
Potential Contributions to Scientific Inquiry
The potential contributions of Synchrony RFS to scientific inquiry are wide-ranging. It can significantly enhance the robustness of experimental designs across multiple disciplines. One critical advantage is its ability to synchronize complex datasets, which is essential in fields like systems biology. By bringing together diverse data sources, Synchrony RFS helps uncover relationships that might otherwise remain hidden.
Moreover, as research in synchronicity evolves, it paves the way for novel applications in drug development and environmental monitoring. For example, Synchrony RFS could provide a comprehensive framework for monitoring chemical reactions in real-time, thus facilitating quicker iterations during the development phase.
"The future of Synchrony RFS is not merely about technology; it's about transforming the way we understand connections in our data."
This makes Synchrony RFS not only a tool for data management but also a key component in hypothesis generation and testing. It encourages researchers to frame new questions and investigate emerging phenomena, further propelling scientific knowledge.
End
The conclusion serves as a critical juncture in understanding Synchrony RFS, offering a necessary synthesis of the discussed concepts and their implications. It is not merely a recap; it emphasizes the significance of the insights gathered throughout the article. Documenting the core findings displays the benefits and considerations surrounding Synchrony RFS, particularly its execution within various scientific fields.
One primary element highlighted in this article is the remarkable versatility of Synchrony RFS across different domains, such as biology, chemistry, and physics. Each application showcases different aspects of its underlying technology, reinforcing its relevance in modern research. Moreover, acknowledging the technical constraints and challenges faced in the practical implementation provides a balanced viewpoint necessary to foster a productive discourse on future improvements.
Additionally, the importance of innovative approaches and potential contributions to scientific inquiry cannot be understated. By examining future directions, researchers and students are encouraged to explore untapped potentials within Synchrony RFS. As such, the conclusion encapsulates the essence of pursuing continuous advancement in this field, ultimately fostering a culture of innovation.
Summary of Key Insights
In summary, the key insights extracted from this article include:
- Core Concepts: Understanding the foundational principles of Synchrony RFS reveals its operational mechanics and theoretical underpinnings.
- Applications: The diverse applications across multiple scientific fields affirm the technology's relevance and adaptability.
- Challenges: Identifying the technical constraints and practical implementation issues provides context for current limitations.
- Future Directions: The exploration of innovative approaches underscores the necessity for ongoing research and development, which is essential for unlocking further applications and reducing existing challenges.
The continual exploration of Synchrony RFS presents an opportunity for not only enhancing existing methodologies but also for defining new avenues in scientific research and collaboration.