Exploring Whole Transcriptome RNA Sequencing Techniques

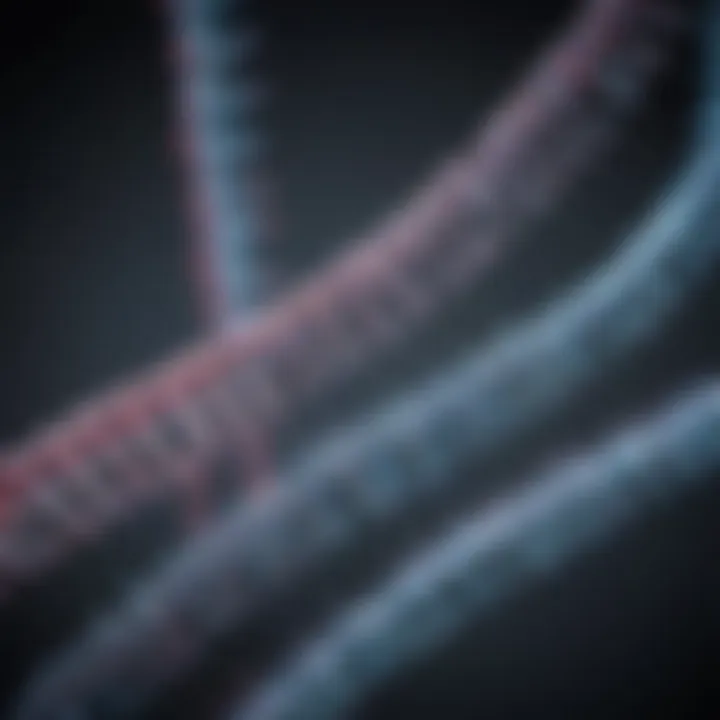
Intro
Whole transcriptome RNA sequencing serves as a remarkable tool in modern molecular biology. This approach allows for the comprehensive analysis of all RNA species in a given sample. The significance of RNA-seq lies in uncovering insights about gene expression, alternative splicing, and non-coding RNAs. As researchers delve deeper into the complexities of cellular processes, whole transcriptome RNA sequencing provides a formidable means to decode the transcripts that govern cellular function.
An array of methodologies is employed in RNA sequencing. Each technique holds unique strengths and presents specific challenges. Understanding these methodologies is crucial for researchers aiming to harness the full potential of this technology. Moreover, applications range widely. From cancer research to developmental biology, the capacity to capture the entire transcriptome enables scientists to explore biological phenomena in unprecedented detail.
In this article, we will examine core elements of whole transcriptome RNA sequencing. We will explore its techniques, diverse applications, and essential considerations for data analysis and experimental design. The aim is to forge a roadmap that provides clarity and depth to both novices and seasoned researchers in the field.
Research Highlights
Overview of Key Findings
Whole transcriptome RNA sequencing presents several key findings that can transform our understanding of gene expression. One major takeaway is the sheer volume of data generated through this sequencing method. Researchers now have access to thousands of transcripts, providing a wider landscape in the investigation of biological questions. Discoveries of novel transcripts and potential non-coding regions highlight the complexity of the transcriptome.
The technology also enhances the ability to capture differential expression patterns that lead to impactful biological discoveries. By analyzing expression levels across various conditions or treatments, scientists can identify potential biomarkers or therapeutic targets.
Significance of the Research
The implications of whole transcriptome RNA sequencing extend well beyond academic inquiry. It plays a crucial role in clinical diagnostics, where understanding gene expression profiles can inform treatment decisions, especially in cancer. Furthermore, the capability to compare transcriptomes across species lays the groundwork for evolutionary studies, providing insight into the fundamental genetic mechanisms that underpin life.
Whole transcriptome RNA sequencing also serves as a foundational approach in the realm of precision medicine. By tailoring treatments to individuals based on their unique transcriptomic profiles, the odds of success in therapies could increase dramatically.
"Whole transcriptome RNA sequencing revolutionizes the way researchers approach the understanding of biological systems, helping to identify novel therapeutic targets and biomarkers."
Prologue to RNA Sequencing
RNA sequencing, commonly known as RNA-seq, represents a transformative advancement in molecular biology. As researchers seek to understand the complexities of gene expression, RNA-seq serves as a fundamental tool for exploring the entire transcriptome. This method can provide unprecedented insights into the dynamic nature of RNA within a cell.
Understanding RNA sequencing is essential for anyone involved in genomic research. The technique allows for both qualitative and quantitative analysis, enabling scientists to observe not just the presence of RNA molecules but also their abundance in various biological contexts.
Defining RNA Sequencing
RNA sequencing refers to a high-throughput sequencing method that captures the entire RNA content of a sample. Unlike traditional methods, which often look at a select group of genes, RNA-seq evaluates all transcripts, including mRNAs, non-coding RNAs, and small RNAs. The process begins with the extraction of RNA, which is then converted into complementary DNA (cDNA) through reverse transcription. This cDNA is subsequently sequenced using various technologies, such as those employed by Illumina or Oxford Nanopore.
This comprehensive approach allows for a more detailed view of transcriptomic landscapes. Unlike microarrays, which rely on pre-existing knowledge of gene sequences, RNA-seq provides the flexibility to identify novel transcripts, splice variants, and unexpected gene expression patterns.
Importance of Whole Transcriptome Analysis
Whole transcriptome analysis is pivotal in understanding the intricacies of gene expression and regulation. This approach empowers researchers to:
- Identify novel transcripts that may play significant roles in cellular functions.
- Analyze differential gene expression across various conditions and diseases.
- Study post-transcriptional modifications that may affect RNA stability and translation.
The ability to sequence the entire RNA content of a sample fosters groundbreaking discoveries in fields such as developmental biology, cancer research, and neurobiology. By investigating variations in expression profiles, scientists can elucidate mechanisms underlying diseases, contributing to potential therapeutic targets.
"Whole transcriptome RNA sequencing opens up a vast array of possibilities for understanding gene function and regulation, providing insights beyond traditional methodologies."
Emphasizing the breadth of applications, whole transcriptome analysis serves as a bridge between our current understanding of molecular biology and the complexities inherent in gene regulation and expression. As the landscape of biomedical research continues to evolve, RNA sequencing remains a critical avenue for exploration.
Overview of RNA-Seq Techniques
The field of RNA sequencing (RNA-Seq) plays a crucial role in revealing the complexities of the transcriptome. Understanding the techniques involved in RNA-Seq is essential for any researcher seeking to leverage this powerful technology for various applications. The methodologies outlined here will inform best practices and highlight the considerations necessary for successful experiments.
Library Preparation
Library preparation is a foundational step in RNA sequencing that converts RNA into a form suitable for sequencing. This process is vital because the accuracy and quality of the sequencing results are heavily influenced by the library construction.
Methodologies for Library Construction
Several methodologies exist for library construction, each with unique attributes and applications. A common choice is the use of poly-A selection, which captures only polyadenylated mRNA. This method simplifies the complexity of the transcriptome and enriches for mRNAs of interest. Another approach, random priming, allows for the inclusion of non-polyadenylated RNAs, thus providing a more comprehensive view of the transcriptome.
The key characteristic of these methodologies is their ability to focus on specific RNA populations, which improves sensitivity and detection of low-abundance transcripts. The choice of method generally depends on the research objectives. The unique feature of poly-A selection is its efficiency in isolating mRNA, but this can inadvertently overlook important transcripts.
Advantages:
- Enhanced sensitivity for mRNA detection.
- Streamlined workflow for standard mRNA studies.
Disadvantages:
- Non-polyadenylated transcripts might be missed.
Quality Control Measures
Quality control measures are essential throughout the library preparation process to ensure accurate downstream results. This includes assessing the integrity and concentration of RNA prior to library preparation, as well as evaluating the final library. Methods like bioanalyzer or qPCR can be utilized to gauge the quality of the cDNA libraries.
The primary characteristic of quality control measures is their preventative nature. They help identify problems early, thus reducing costly mistakes in subsequent sequencing phases. These measures help ensure that only high-quality libraries proceed to sequencing.
Advantages:
- Reduces the rate of sequencing failures and invalid data.
- Provides confidence in the reliability of results.
Disadvantages:
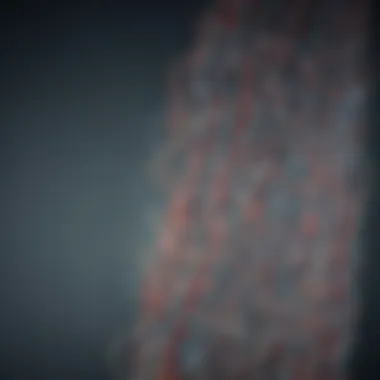
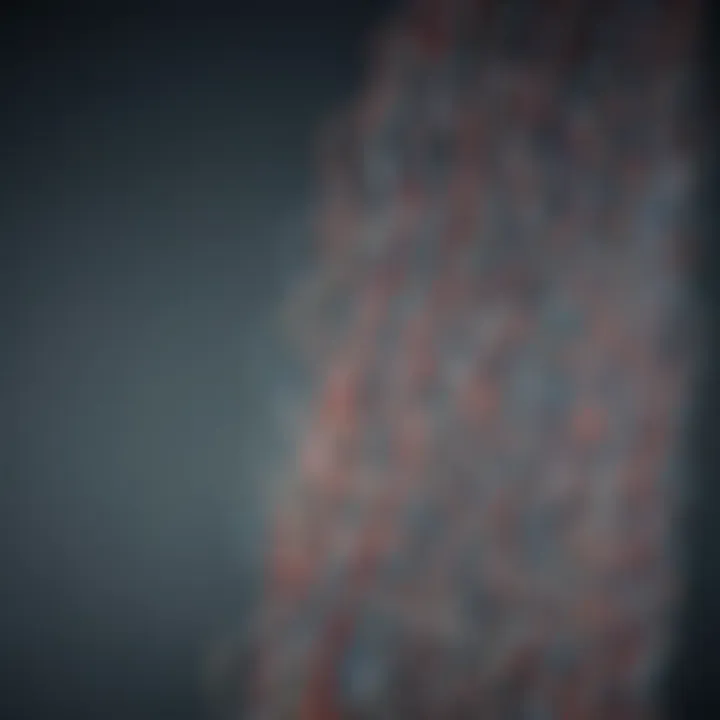
- Can add time and complexity to the overall workflow.
Sequencing Platforms
Selecting the right sequencing platform is a pivotal aspect of RNA-Seq studies. Different platforms offer various advantages and limitations that impact the quality and depth of sequencing.
Comparing Illumina and Oxford Nanopore Technologies
Illumina and Oxford Nanopore Technologies are two leading platforms utilized in RNA sequencing. Illumina is widely recognized for its high-throughput capabilities and accuracy. It can generate millions of reads, making it suitable for large-scale genomic studies. On the other hand, Oxford Nanopore allows for longer reads and real-time sequencing, which can capture full transcripts and structural variants more effectively.
The key characteristic of Illumina platforms is their established scalability and data consistency. In contrast, Oxford Nanopore's unique ability to produce long reads is advantageous for specific applications requiring detailed transcript analysis.
Advantages of Illumina:
- High data throughput.
- Cost-effective for large-scale studies.
Disadvantages of Illumina:
- Short read lengths limit the assembly of complex transcripts.
Advantages of Oxford Nanopore:
- Long read lengths capture full transcripts.
- Real-time sequencing allows for immediate data feedback.
Disadvantages of Oxford Nanopore:
- Higher error rates compared to Illumina.
Platform-Dependent Considerations
Platform-dependent considerations must be assessed to align sequencing technologies with research objectives. Key factors include read length, sequencing depth, and cost considerations, which can influence the choice of platform. The complexity of the transcriptome in the biological sample may also dictate specific needs, such as the ability to detect splice variants or novel transcripts.
A comparative analysis of these factors is crucial in designating the suitable platform. Technical specifications, such as data output and error rates, must also be factored into the decision-making process.
Advantages:
- Informed decisions based on project goals.
- Maximized efficiency and resource utilization.
Disadvantages:
- Misalignment between platform capabilities and project needs can lead to suboptimal results.
Data Analysis in RNA Sequencing
The process of data analysis in RNA sequencing is crucial for extracting meaningful insights from the generated data. Understanding how to interpret these data allows researchers to draw connections between gene expression patterns and biological functions. Data analysis encompasses various methodologies that contribute significantly to the overall understanding of transcriptional landscapes. It is important not just to obtain raw data but to ensure that the results are robust and reproducible.
In RNA-Seq, the primary steps involve data processing and quantification of gene expression. Each step holds its unique importance. Thorough data analysis can reveal information about not only the quantity of RNA present but also the nuances of the biological context that might be missed when relying on simpler approaches. This section will delve deeper into the critical steps involved in the data analysis process.
Data Processing Steps
Quality Control of Raw Data
Quality control of raw data is an essential first step in RNA sequencing analysis. It allows researchers to filter out low-quality reads that may skew the results. This process involves assessing the integrity of the sequencing reads and identifying any potential issues that could affect downstream analysis. Tools like FastQC provide valuable insights into quality metrics such as base quality scores and read lengths.
The key characteristic of quality control is its emphasis on ensuring data reliability. This fact makes it a necessary step in any RNA-Seq study, as poor-quality data can produce misleading results.
A unique feature of quality control processes is the application of trimming algorithms, which can remove adapters and low-quality sequences from raw reads. The advantages of implementing such measures include improved accuracy of read alignment and better quantification of gene expression levels. Without proper quality control, results may lead to erroneous biological interpretations, ultimately impacting research findings.
Read Alignment Methods
Read alignment methods play a pivotal role in the data analysis workflow for RNA sequencing. This step involves mapping the sequenced reads back to a reference genome or transcriptome. Accurate alignment is essential for precise quantification of gene expression levels.
One notable characteristic of read alignment methods is their ability to handle complex genomic regions, including splice junctions. This capacity makes them a popular choice for RNA-Seq studies, as they can accurately reflect the underlying transcript structure.
Unique features of widely used alignment tools such as STAR and HISAT2 include their speed and robustness in dealing with large datasets. Each method brings its advantages and disadvantages, such as computational resource requirements and sensitivity to read length variations. Analyzing these factors is crucial for optimizing the research project's efficiency.
Quantification of Gene Expression
The quantification of gene expression is a fundamental aspect of RNA sequencing analysis. Accurate quantification allows researchers to determine the expression levels of thousands of genes simultaneously. This is crucial for understanding the functional implications of gene regulation in various biological contexts.
Fragment Counting Techniques
Fragment counting techniques serve as a foundational approach in quantifying gene expression from RNA sequences. These techniques involve counting the number of sequencing reads mapped to specific genes or genomic regions. By representing the expression level as a count of fragments, researchers can make comparative analyses between different samples or conditions.
A key characteristic of this method is its straightforwardness; it provides a direct measurement of transcript abundance. This quality makes fragment counting a popular choice for many RNA-Seq studies.
The unique feature of this approach lies in its simplicity and speed. However, it can also present some drawbacks, such as biases related to gene length or sequencing depth. Properly addressing these limitations is essential for obtaining accurate and interpretable results.
Normalization Procedures
Normalization procedures are vital for ensuring that gene expression estimates are comparable across different samples. This process corrects for systematic biases that can arise from variations in sequencing depth, sample composition, and other technical factors. Accurate normalization facilitates meaningful biological comparisons.
A key characteristic of normalization is its ability to adjust for these variability factors while retaining true biological differences. This aspect makes it an indispensable part of data analysis in RNA sequencing.
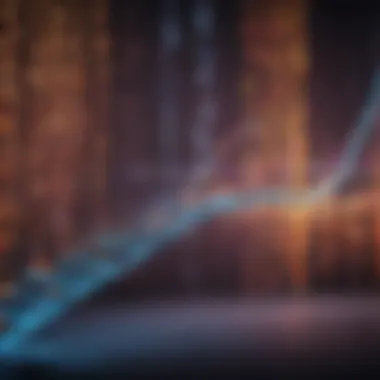
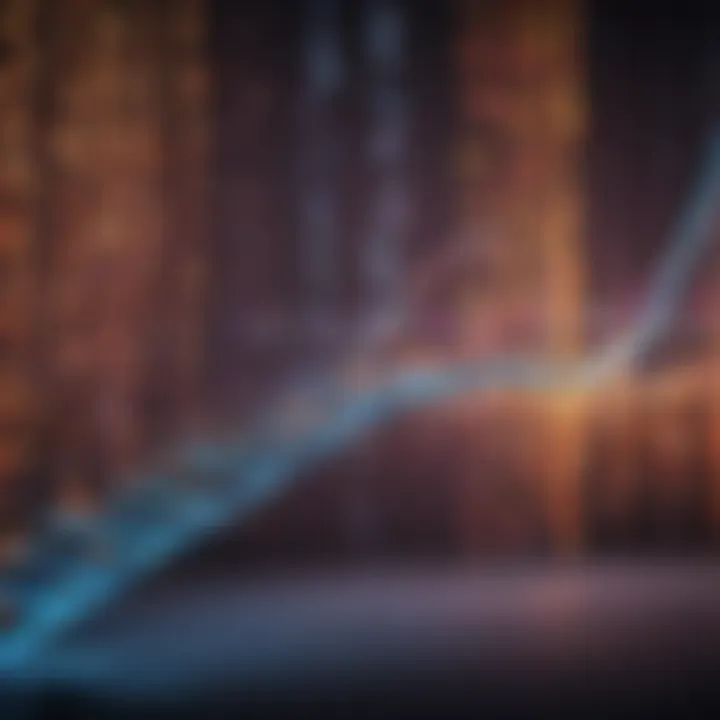
Unique features of common normalization techniques include methods like Reads Per Kilobase of transcript per Million mapped reads (RPKM) and Transcripts Per Million (TPM). While RPKM factors in gene length, TPM adjusts for both gene length and sequencing depth. Each method carries specific advantages and disadvantages regarding sensitivity and interpretability. Understanding these differences is critical for choosing the right normalization approach in a given study.
Applications of Whole Transcriptome RNA Sequencing
Whole transcriptome RNA sequencing (RNA-Seq) is more than a technique; it is an evolving platform with multiple applications that directly influence our understanding of biological processes. In this section, we will delve deeply into significant applications that leverage this powerful technology. By examining diverse areas of research and their implications, we can appreciate the transformative power of whole transcriptome RNA-Seq.
Identifying Novel Transcripts
The ability to identify novel transcripts is one of the most exciting aspects of whole transcriptome RNA sequencing. This application opens avenues for discovering previously unknown genes and regulatory elements. As researchers sequencing various tissues or organisms, RNA-Seq can reveal non-coding RNAs and novel splice variants that traditional methods might overlook.
The approach's sensitivity thus allows for capturing RNA not just from expressed genes but also from regions of the genome previously deemed silent. Importantly, by understanding these novel transcripts, scientists can better dissect the complexities of gene regulation and expression, leading to potential breakthroughs in functional genomics.
Differential Gene Expression Analysis
Differential gene expression analysis is another pivotal application of RNA-Seq, providing insights into how gene expression varies across different conditions or treatments. This analysis allows researchers to compare the transcriptomes of different samples to identify genes that are upregulated or downregulated in specific circumstances.
Something significant about RNA-Seq is its capacity to handle high-throughput datasets, which means it can process thousands of genes simultaneously. This efficiency provides a more comprehensive view of cellular responses, making it invaluable in scenarios such as drug response studies or environmental stress assessments.
Role in Disease Research
Cancer Genomics
Cancer genomics harnesses whole transcriptome RNA sequencing to explore the complex landscape of tumors. It analyzes the changes in gene expression that contribute to tumor development and progression. One major highlight of cancer genomics is its role in identifying biomarkers that might predict patient responses to therapy. Researchers can analyze tumor samples at different stages, providing crucial information about tumor heterogeneity.
Furthermore, the unique feature of this application is the ability to reveal gene fusions and mutations not evident through other methods. This characteristic strengthens its appeal within cancer research since treatment can be tailored based on individual tumor profiles. However, challenges remain due to the variability in tumor biology, which can complicate data interpretation.
Neurodegenerative Disorders
Neurodegenerative disorders pose distinct challenges, and RNA-Seq plays a vital role in dissecting the molecular mechanisms underlying these diseases. By comparing the transcriptomes of affected versus healthy tissues, researchers can pinpoint specific genes and pathways linked to neurodegeneration.
One of the key characteristics of studying neurodegenerative disorders through RNA-Seq is the focus on the subtle transcriptomic changes that occur long before clinical symptoms manifest. This advantage allows for the discovery of potential early biomarkers or therapeutic targets. However, the disadvantage lies in the complexity of biological systems involved, which may confound results resulting in challenges for reproducibility and interpretation.
By exploring these applications, we can see how whole transcriptome RNA sequencing bridges the gap between basic science and practical applications. Each advancement in addressing biological complexities enhances our demands for improved methodologies tailored for specific research needs.
Considerations in RNA Sequencing Studies
Considering various factors when conducting RNA sequencing studies is vital for the reliability of results. These factors can influence data accuracy, interpretation, and ultimately the outcomes of biological research. Proper attention to these considerations ensures that the findings are not just statistically significant, but also biologically relevant. This section focuses on key elements that researchers should address when planning and executing RNA-seq experiments.
Experimental Design
Sample Selection and Replication
The process of sample selection is crucial in RNA sequencing studies. Choosing the right samples is fundamental. Samples must represent the population from which they are drawn. This helps ensure the results are generalizable. Replication is another key aspect. Having multiple biological replicates increases the reliability of the data. Replication can identify variability and reduce technical errors.
Key features of sample selection and replication include:
- Ensuring a diverse representation of the biological conditions
- Increasing data robustness through biological replication
However, selecting samples can be challenging. Researchers must balance practical constraints with the need for representativeness. The potential limitation is that if the sample is not diverse enough, conclusions drawn may be biased or incorrect.
Controlling for Batch Effects
Batch effects arise when different batches of samples are processed under varying conditions. These effects can skew results, causing false positives or negatives. Thus, controlling for batch effects is essential. Researchers should randomize samples across batches and include replication within each batch. This action helps mitigate potential biases.
Key aspects of controlling for batch effects are:
- Randomizing sample processing
- Utilizing computational methods to adjust data post-sequencing
Incorporating these measures can prevent misleading interpretations. However, batch effects can still be a persistent concern. Some inherent variability in sequencing and processing may remain, potentially affecting results even with controls in place.
Interpreting RNA-Seq Results
Interpreting RNA-seq results requires a nuanced understanding of statistical significance. It is crucial not to overstate findings based on p-values alone. Researchers should consider the biological implications of their results rather than relying entirely on statistical thresholds. High-dimensional data can lead to overfitting models, making broad generalizations risky.
Understanding Statistical Significance
Statistical significance determines whether the observed results are likely due to chance. For RNA-seq studies, it is critical to employ appropriate statistical models. Utilizing methods like false discovery rate (FDR) control can help provide a clearer understanding of significant findings.
Features of understanding statistical significance include:
- Identifying true positive results
- Preventing erroneous conclusions based on p-values alone
Yet, solely focusing on statistical significance can be misleading. Researchers should always put results in a biological context. This holistic approach improves the interpretation of findings.
Biological Interpretation of Findings
Beyond mere statistical outputs, biological interpretation of findings underscores the relevance of discoveries. It connects the data back to the biological questions initially posed. Understanding biological significance helps elucidate the underlying mechanisms at play. Researchers must integrate their results with existing biological knowledge to derive meaningful conclusions.
Aspects of biological interpretation include:
- Linking findings to known biological pathways
- Considering the physiological relevance of statistically significant changes
Failure to appreciate the biological context may lead to superficial conclusions. This oversight constitutes one of the primary pitfalls in RNA sequencing analyses, ultimately affecting scientific progress.
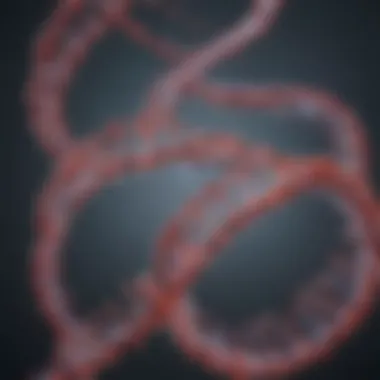
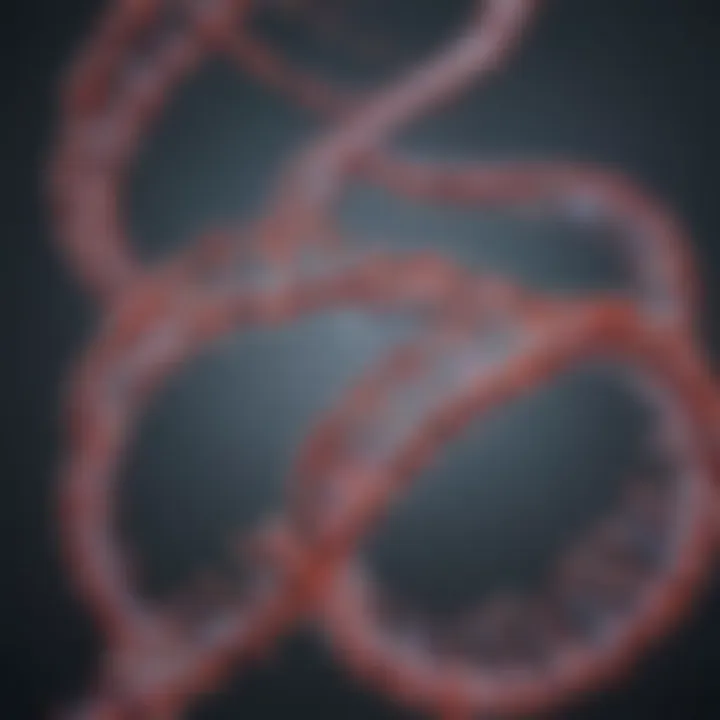
An effective RNA-seq study is defined not only by robust statistical methods but also by its biological relevance. The interplay between these aspects enriches the understanding of complex biological phenomena.
Challenges and Limitations
Considering the challenges and limitations of whole transcriptome RNA sequencing is imperative. This aspect of RNA sequencing can impact the data quality, interpretability, and ethical implications of research findings. Understanding these challenges helps researchers to design better experiments and make informed choices about the techniques they employ.
Technical Limitations
Coverage Bias Issues
Coverage bias refers to uneven representation of transcripts during sequencing. It can happen when some regions of RNA are more abundant than others, leading to more reads from those areas. This bias can distort conclusions about gene expression levels. A key characteristic of coverage bias is the potential to overlook low-abundance transcripts. Such an issue is significant as it skews the overall understanding of the transcriptome. Coverage bias may lead to missing crucial genes that could be relevant for certain conditions or responses.
The disadvantage of this bias is that it complicates the quantification of gene expression. Bisecting the transcriptome based on biased coverage can lead researchers to form incorrect hypotheses. By being aware of coverage bias, researchers can employ strategies to minimize its effects. For example, using deeper sequencing may help, but it often comes at a higher cost.
Impact of Noise in Data
Noise in sequencing data arises from technical variations during the sequencing process. This noise can obscure true biological signals, posing a challenge for accurate interpretation. A key characteristic of noise is its ability to mimic real biological variation, leading to false conclusions. This is a major concern in RNA sequencing as it can contribute to inaccuracies in measuring gene expression.
When noise is present, the significance of detected changes in expression can be misrepresented. Detrimental impacts of noise highlight the need for robust filtering and statistical methods during analysis. On the other hand, identifying true signals becomes more crucial. For this reason, considerable attention must be paid to noise reduction techniques to improve data quality.
Ethical Considerations
As RNA sequencing continues to evolve, ethical considerations are becoming increasingly important. These include privacy concerns and informed consent practices, both of which play a critical role in the responsible use of genomic data.
Privacy Concerns in Genomic Data
Privacy is one of the primary ethical concerns in RNA sequencing. Since genomic data can reveal sensitive personal information, it is essential to safeguard this information. A key characteristic of privacy issues relates to the risk of re-identification of individuals from data, especially when samples are from identifiable subjects.
This concern emphasizes the importance of data anonymization and encryption. Protecting the privacy of subjects is not only a legal obligation but also a moral imperative. Researchers need to understand the implications of mishandling genomic data. Failure to protect this information could have significant repercussions.
Informed Consent Practices
Informed consent is vital in any research involving human subjects. It is essential that participants fully understand what their samples will be used for, including RNA sequencing. A key characteristic of informed consent practices is transparency in communicating the risks and potential uses of genomic data. As such, participants should be fully informed of any potential implications.
The unique feature of informed consent practices is that they must evolve alongside advancing technologies. Researchers should regularly update their consent forms to reflect the specifics of current research methodology. While achieving this goal can be challenging, thorough informed consent practices are necessary to maintain ethical standards.
Understanding the challenges and limitations of RNA sequencing informs future research designs and ethical frameworks.
Navigating the complexities of technical limitations and ethical issues against the backdrop of whole transcriptome RNA sequencing is critical. Recognition of these factors will guide researchers to make well-informed decisions throughout their studies.
Future Directions in RNA Sequencing
The field of RNA sequencing is evolving rapidly, driven by technological advancements and a growing understanding of biological complexities. This section discusses the future directions in RNA sequencing, which are essential in shaping research in genomics and molecular biology. The continued refinement of techniques, along with the integration of diverse datasets, can lead to a more comprehensive understanding of gene expression and regulation.
Emerging Technologies
Single-Cell RNA Sequencing
Single-cell RNA sequencing represents a significant shift in how we study gene expression at an unprecedented resolution. Unlike traditional bulk RNA sequencing, which averages gene expression across many cells, single-cell methods allow researchers to analyze the transcriptome of individual cells. This granularity helps in uncovering the heterogeneity within tissues, revealing how different cells contribute to overall biological functions.
A key characteristic of single-cell RNA sequencing is its ability to identify rare cell populations that may play critical roles in development or disease. This technique has become a popular choice for researchers interested in cancer, immunology, and developmental biology. The unique feature of single-cell RNA sequencing is that it can provide insights into cell-type-specific expression patterns and regulatory mechanisms.
However, there are challenges involved. The sensitivity of the method can lead to increased noise in the data, making interpretation complex. Additionally, the cost and technical intricacies can be prohibitive for some labs.
Long-Read Sequencing Advances
Long-read sequencing technologies have also gained prominence in the landscape of RNA sequencing. These advancements allow for the production of much longer sequence reads compared to traditional methods, which can be vital for accurately assembling complex transcripts. In particular, long-read sequencing is adept at resolving genomic regions that contain repetitive sequences, as well as full-length isoforms of genes.
A primary advantage of long-read sequencing is its capacity to capture entire transcripts, which is critical for discovering novel splice variants and understanding genes' full diversity. This characteristic makes it a beneficial choice for comprehensive transcriptomic studies.
Despite these advantages, long-read sequencing technologies often come with higher error rates and costs per base, which can impact the overall reliability of the data. Researchers must weigh these factors when deciding on sequencing strategies.
Integration with Other Omics Data
The integration of RNA sequencing data with other omics technologies, such as genomics, proteomics, and metabolomics, will further enhance our understanding of biological systems. By combining transcriptomic profiles with genomic variations, proteomic data, and metabolic pathways, researchers can create a systems biology approach to study complex diseases.
In summary, the future of RNA sequencing is lined with innovative technologies and approaches that promise to significantly enhance our understanding of gene expression and its implications in health and disease.
Through the continuous development of single-cell methods and the adoption of long-read technologies alongside multi-omics integration, researchers are well poised to make groundbreaking discoveries in molecular biology.
Ending
Whole transcriptome RNA sequencing offers significant potential for advancing our understanding of gene expression and regulation. The importance of this technology cannot be overstated. It enables researchers to gain insights into the full range of RNA molecules present in a sample, enhancing the understanding of cellular functions and the dynamics of various biological processes.
Summarizing Key Insights
In summarizing the insights gained from our exploration, several key elements emerge:
- Broad Applications: Whole transcriptome RNA sequencing is applicable in various fields, from cancer genomics to neurodegenerative research, providing crucial data for therapeutic strategies and disease understanding.
- Technical Complexity: The methodologies and platforms differ widely, highlighting the importance of selecting the appropriate tools and techniques based on the specific research question and sample type.
- Data Interpretation: The nuanced nature of RNA sequencing data requires careful consideration of statistical analysis and biological relevance, as improper interpretation can lead to misleading conclusions.
A collaborative approach among biologists, bioinformaticians, and other specialists is essential to maximize the benefits of this technology and address its inherent challenges.
The Future of Whole Transcriptome Analysis
Looking ahead, the future of whole transcriptome analysis promises exciting developments. Emerging technologies such as single-cell RNA sequencing will likely revolutionize how researchers approach transcriptomics, offering insights into cellular heterogeneity that bulk RNA sequencing cannot provide. Moreover, advancements in long-read sequencing technologies will enhance the ability to analyze complex transcripts and alternative splicing variants.
Additionally, integrating RNA sequencing data with other omics technologies, such as genomics and proteomics, will yield a more comprehensive view of biological systems. This multi-omics approach will aid in addressing complex biological questions, ultimately facilitating the development of personalized medicine.
As researchers continue to refine methodologies and explore new applications, the potential for whole transcriptome RNA sequencing to contribute meaningfully to molecular biology and related fields remains promising.