Big Data Analytics: Challenges and Opportunities
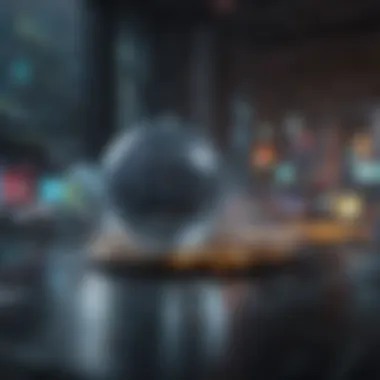
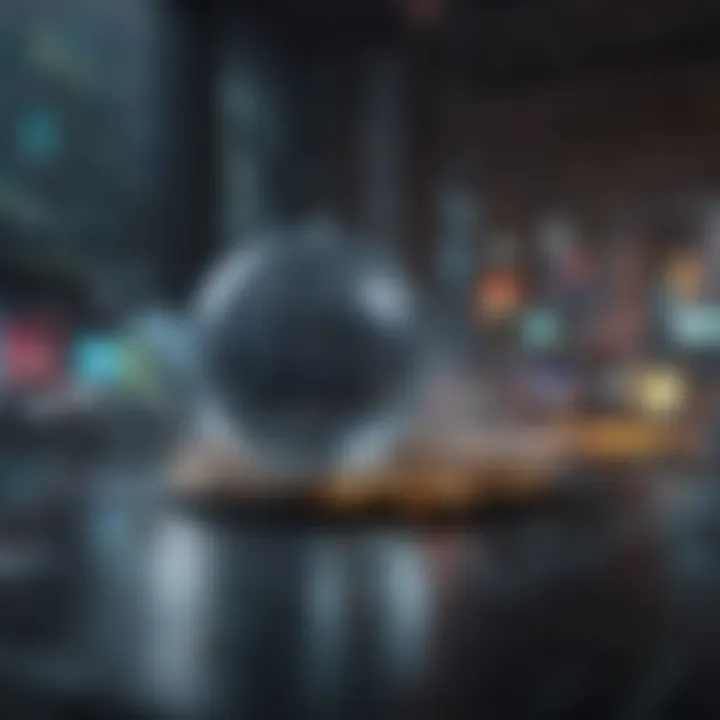
Intro
In the constantly evolving landscape of information science, big data analytics has emerged as a critical player, influencing decision-making across a myriad of sectors. With the proliferation of data generated daily, organizations face a dual-edged swordāopportunity and complexity. This complexity is not merely rooted in the sheer volume of data but also in its variety, velocity, and veracity. Understanding how to navigate these waters is essential for professionals and researchers alike.
Big data analytics allows one to sift through oceans of information, extracting valuable insights that propel organizations forward. Whether it's in healthcare, finance, retail, or any other industry, the ability to analyze large datasets can translate to strategic advantages. From predicting consumer behavior to enhancing operational efficiencies, the implications are profound.
Moreover, big data analytics is intertwined with ethical considerations. As organizations harness this wealth of information, they must also grapple with privacy concerns and data governance. The power to analyze does not come without responsibility.
As we delve deeper into this topic, we'll illuminate the key components of big data analytics, explore the methodologies employed, and examine real-world applications that highlight its transformative power.
Preamble to Big Data Analytics
Big data analytics is todayās clarion call in the information age, steering towards a profound transformation in decision-making processes across various industries. Itās not merely a buzzword; itās a catalyst that can potentially unravel the complexities inherent in vast data sets while illuminating the path for informed choices and strategic advancements. Whether in healthcare, finance, or marketing, understanding the frameworks for managing and analyzing big data is essential.
Definition and Scope
Big data can be a rather sprawling concept. At its core, it refers to data sets that are so large or complex that traditional data processing software cannot manage them effectively. However, it goes beyond just sizeāit encompasses the velocity at which data is generated, the variety of data types, and the veracity regarding its accuracy and reliability. A variety of industries have now recognized that these massive volumes of information offer unobstructed insights that can drive significant value when harnessed correctly.
The scope of big data analytics stretches far and wide. It includes:
- Descriptive Analytics: This aspect takes a look back to explain what has happened in the past using historical data. Simple statistics can reveal patterns and trends that are essential in drawing conclusions.
- Predictive Analytics: This anticipates future outcomes based on past data. Using algorithms and machine learning, businesses can forecast trends and prepare for upcoming events.
- Prescriptive Analytics: This evaluates past and present data to recommend actions. It often involves complex algorithms and advanced analytics techniques, transforming predictions into actionable strategies.
In outline, excelling in big data analytics demands not just a grasp of the techniques utilized but also a deep understanding of a business's specific objectives, thereby fostering data-driven decision-making.
Historical Context
Tracing back the roots of big data, one can see an evolution shaped by technological advancements. The journey began long before the term ābig dataā hit the books. Initially, data collection was a tedious and manual process. As computers emerged, data began to be gathered electronically, leading to a massive upswing in the volume and complexity of data generated.
In the 1990s, companies like Google pushed the envelope with innovative storage and processing solutions. The development of Hadoop in the mid-2000s marked a significant milestone in big data history. This technology enabled the storage of massive amounts of data across distributed systems, making data processing more accessible than ever.
Fast forward to the present and we find ourselves at a critical juncture. As we delve deeper into big data analytics today, thereās no denying its influence, visible in how businesses interact with customers, manage operations, and engage in strategic planning.
"In a world full of data, the ability to analyze and derive insights is what sets successful organizations apart."
Understanding the historical framework of big data analytics not only serves to underscore its significance but also prepares us for the future. As technology continues to develop, the tools and methods of big data will evolve, shaping our relationship with information in ways we are yet to fully comprehend.
The Significance of Big Data in Contemporary Society
In our fast-paced world, big data has grown from a buzzword into an integral part of how organizations drive success and make informed decisions. The sheer volume of data generated dailyāfrom social media interactions to consumer transactionsāhighlights its inexorable impact on various facets of life. At its core, big data analytics offers the framework to sift through this mass of information, illuminating patterns and trends that were once as elusive as finding a needle in a haystack. The significance of big data goes beyond mere statistics, influencing everything from corporate strategies to societal changes.
Impact on Industries
Big data analytics is not confined to a single sector; rather, its influence spans numerous industries, acting as a catalyst for innovation and efficiency. Consider healthcare, where patient data is analyzed to identify disease outbreaks or personalize medicine. The powerful capabilities of big data allow for rapid response to public health crises, leading to quicker diagnoses and treatments.
In retail, companies such as Amazon utilize big data to tailor customer experiences. By analyzing purchasing patterns and preferences, they can predict future buying behavior. This not only boosts sales but also enhances customer satisfaction, creating a loop of loyalty and repeat business.
Here are some ways big data is reshaping industries:
- Manufacturing Efficiency: Predictive analytics helps foresee machine maintenance needs, reducing downtime.
- Telecommunications: Providers utilize data to optimize network performance and customer service.
- Agriculture: Farmers employ big data to monitor crop health and optimize resource usage, increasing yields.
As industries adapt to the realities of big data, those that can leverage it effectively enjoy a significant edge over competitors.
Influence on Decision Making
In the realm of decision making, the role of big data analytics cannot be overstated. It transforms raw data into actionable insights, allowing organizations to make informed choices based on factual evidence rather than intuition alone. For example, banks use big data to assess credit risk more accurately, which allows them to extend loans responsibly and minimize defaults.
Moreover, marketers now rely on comprehensive data sets to tailor campaigns to focus on audiences that are more likely to convert. This not only saves money but also enhances the effectiveness of marketing efforts.
The benefits of data-driven decision making include:
- Increased Agility: Organizations can pivot strategies swiftly in response to emerging trends.
- Enhanced Customer Insights: A deeper understanding of customer behaviors and preferences leads to better product development.
- Operational Improvements: Data analytics helps identify inefficiencies, enabling companies to streamline operations.
"In the digital age, those who master the complexities of data are the ones who will steer the ship toward success."
As decision-makers become more adept at interpreting data, the reliance on intuition diminishes, creating a culture where strategic planning is firmly anchored in analytical evidence.
Data Management Techniques
Data management techniques are the backbone of any successful big data analytics initiative. In a world where data is generated at an unprecedented rate, it's essential to implement effective methods for collecting, storing, and processing this information. By mastering data management, organizations can harness insights that drive strategic decision-making and foster innovation. This section distills the core components of data management techniques, emphasizing their impact on data integrity, accessibility, and overall usability.
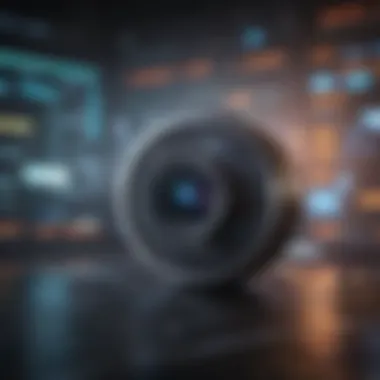
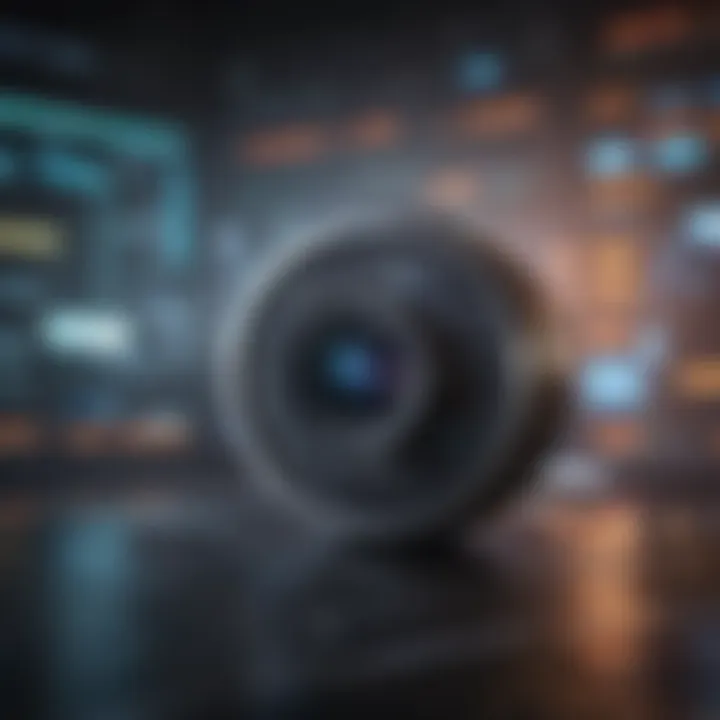
Data Collection Methodologies
Data collection methodologies refer to the systematic approaches employed to gather information from various sources. With big data coming from diverse channelsālike social media, sensors, transactionsāunderstanding how to collect this data effectively is critical.
Different methodologies may be employed, such as:
- Surveys and Questionnaires: Often used to collect qualitative and quantitative data from target populations. They can offer valuable insights into consumer preferences and behaviors.
- Observational Techniques: This involves directly observing subjects in their natural environment to gather unfiltered data, which is particularly useful in social sciences and market research.
- Web Scraping: Involves extracting large amounts of data from web pages, providing insights into market trends, competitor strategies, and customer sentiments.
One of the main benefits of a robust data collection methodology is the ability to ensure high-quality data that can be analyzed effectively. If the data collected is flawed or biased, the resulting analysis may lead to inaccurate conclusions.
Data Storage Solutions
Once data is collected, the next crucial step involves selecting appropriate data storage solutions. The sprawl of big data necessitates systems that can handle vast amounts of information while ensuring security and quick retrieval.
Popular data storage solutions include:
- Data Warehousing: This method consolidates data from multiple sources into a central repository. It simplifies analytics and reporting across the organization. For instance, platforms like Amazon Redshift or Google BigQuery are prominent options.
- Cloud Storage: With the rise of remote work and digital transformation, cloud solutions like Microsoft Azure and Dropbox offer scalable storage that is accessible from anywhere.
- NoSQL Databases: Unlike traditional relational databases, NoSQL systems such as MongoDB and Cassandra are designed for unstructured data, allowing for agile data modeling and retrieval.
Selecting the right storage solution depends on multiple factors, including scalability, data type, and access speed. More so, organizations must remain vigilant about security protocols to protect sensitive information against breaches.
Data Processing Frameworks
Data processing frameworks are indispensable for transforming raw data into actionable insights. This involves structuring and analyzing data to derive meaningful conclusions. The choice of data processing framework heavily influences performance and scalability.
Key frameworks to consider include:
- Apache Hadoop: A popular open-source software that enables distributed processing of large data sets across clusters of computers using simple programming models.
- Apache Spark: Known for its speed and efficient processing, Spark can handle batch and real-time data processing, making it versatile for modern applications.
- ETL Tools: Extract, Transform, Load (ETL) tools like Talend or Informatica are designed to integrate various data sources and prepare them for analysis.
Using efficient data processing frameworks allows organizations to accelerate their analytics cycles, enabling rapid response to market shifts or operational issues.
In summary, effective data management techniquesācomprising robust data collection methodologies, secure and efficient storage solutions, and powerful processing frameworksāare essential for leveraging big data analytics to its fullest potential. Engaging with these components not only ensures informed decisions but also fuels innovation across sectors.
Methods in Big Data Analytics
Big data analytics is not just a buzzword; it's at the heart of modern decision-making and competitive advantages across many industries. The methods used in big data analytics form the backbone of how organizations derive insights and make informed decisions. Understanding these methods is crucial, as being able to interpret and apply them dictates the effectiveness of the analytics process. In this section, we will dive deep into the three key methodologies utilized in big data analytics: descriptive, predictive, and prescriptive analytics. Each of these methods serves a different purpose, and grasping their unique benefits is essential for navigating this complex field.
Descriptive Analytics
Descriptive analytics plays a vital role in providing a clear picture of historical data. It focuses on summarizing past events to help organizations understand what has happened and the underlying causes. For instance, looking at sales data from previous years can inform a retailer about trends, seasonal impacts, and customer preferences.
Some key aspects include:
- Data Visualization: Tools help create graphs and charts that concretely represent data findings.
- Statistical Analysis: Basic statistical methods are used to analyze data sets to uncover patterns.
- Business Intelligence: Organizations leverage descriptive analytics to refine strategic decisions and enhance overall performance.
"Descriptive analytics can be thought of as a story whereby past events are captured to help explain the context of current circumstances."
Predictive Analytics
Predictive analytics takes a step further by using historical data to forecast future outcomes. It's akin to peering into a crystal ball of sorts; organizations evaluate past data trends to predict what might happen in the future. Imagine a financial institution using customers' past spending data to estimate their future credit risks.
Key components of predictive analytics include:
- Machine Learning Algorithms: These algorithms adapt from data over time, becoming increasingly accurate in their predictions.
- Statistical Modelling: This involves identifying relationships in data and constructing models that forecast future behavior.
- Risk Assessment: Predictive analytics is pivotal in mitigating risks, ensuring that informed decisions are made based on anticipated outcomes.
Prescriptive Analytics
The final ingredient in the analytics trifecta is prescriptive analytics. This method not only predicts what might happen based on historical data but also provides recommendations on how to act upon those predictions. It helps organizations take proactive steps by answering the question of how to optimize strategies based on various scenarios.
Important elements include:
- Optimization Techniques: These are applied to find the best possible solution among a range of choices, such as resource allocation.
- Simulation Models: Organizations can utilize complex simulations to explore different scenarios and their outcomes, paving the way for better strategic decisions.
- Decision Analysis: This aspect evaluates the potential consequences of various actions, allowing businesses to weigh their options thoroughly.
Key Analytical Tools and Technologies
In the expanding field of big data analytics, utilizing the right tools and technologies is crucial. With vast amounts of data pouring in from various sources, it becomes imperative to have analytical tools that can sift through the noise and extract meaningful insights. Employing these tools not only enhances data effectiveness but also drives informed decision-making across industries. Without them, businesses may as well be sailing a ship without a compass in the roaring seas of data.
Overview of Popular Tools
Different industries leverage various big data tools to suit their specific needs. Hereās a glimpse into some of the mainstream tools that have cemented their place in the analytics landscape:
- Apache Hadoop: A key player in the big data space, Hadoop offers distributed storage and processing of large data sets across clusters of computers using simple programming models. Itās open-source, enabling adaptability and widespread usage.
- Cloudera: Providing a versatile data platform, Cloudera integrates machine learning, analytics, and data warehousing. Its on-premise or cloud solutions make it particularly useful for enterprises looking to harness big data capabilities effectively.
- Tableau: Specializing in data visualization, Tableau helps professionals observe their data in comprehensible formats with interactive dashboards. It simplifies complex data interactions, turning insights into visual narratives.
- Apache Spark: Known for its speed and efficiency in processing data, Spark works seamlessly with Hadoop. It supports real-time analytics, which is increasingly critical in various applications like fraud detection.
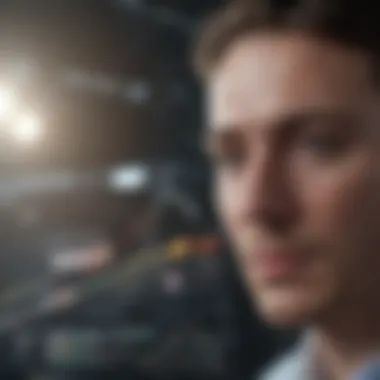
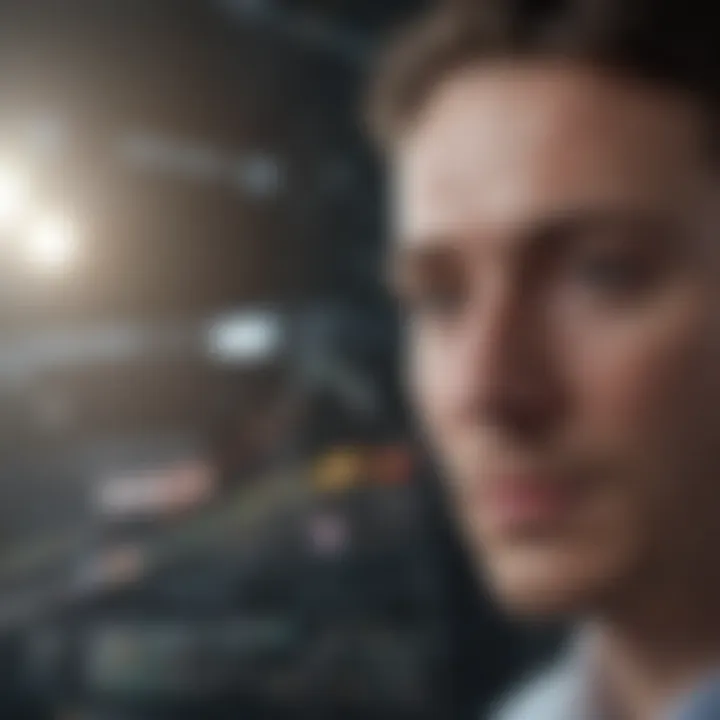
These tools represent just the tip of the iceberg. Each tool boasts a unique set of functionalities, collaboration, and integration potential that can significantly bolster analytics processes across sectors.
Emerging Technologies in Data Analytics
As technology progresses, the scope of data analytics continues to evolve, with new players entering the scene. Staying abreast of these emerging tools is crucial for stakeholders wanting to ensure their data strategies remain relevant. Noteworthy technologies include:
- Machine Learning: This branch of artificial intelligence enables systems to learn from data and improve predictions over time. Tools that implement machine learning can tailor results to specific user behaviors, enhancing personalized experiences.
- Natural Language Processing (NLP): As the interface between humans and computers, NLP helps in extracting insights from textual data, making it invaluable in sentiment analysis and customer feedback evaluation. Tools that utilize NLP are increasingly popular for their ability to derive meaning from unstructured data.
- Cloud-based Solutions: More companies are opting for cloud analytics tools. They offer flexibility, scalability, and cost-effectiveness. Solutions like Microsoft Azure and Amazon Web Services enable businesses to store and analyze data without the hefty investment in infrastructure.
"The true value of data analytics lies not in the data itself but in the ability to interpret and act upon insights derived from it."
- Data Lakes: Unlike traditional databases, data lakes allow for the storage of large volumes of unstructured data. This facilitates machine learning and big data analytics, enabling organizations to keep all their data in one place for deep analysis.
These emerging technologies reflect a shift towards more agile and responsive analytical frameworks, which are vital given the sheer volume and complexity of modern data environments. Companies embracing such innovations can uncover new opportunities and maintain competitive advantages in their respective industries.
Applications of Big Data Analytics
Big Data Analytics is not just a buzzword thrown around in boardrooms; it has practical applications that drive real change across various industries. The integration of vast datasets, sophisticated algorithms, and powerful computing systems has unlocked new avenues for innovation, efficiency, and insight. Particularly, understanding the applications of big data analytics is essential for comprehending its relevance in today's fast-paced environment. It's all about making sense of the chaos and turning data into actionable knowledge.
Some specific elements that highlight the importance of these applications include:
- Enhanced Efficiency: Businesses leverage data analytics to streamline operations, reducing waste and optimizing processes.
- Informed Decision-Making: Organizations utilize data-driven insights to guide strategic decisions, from resource allocation to market entry.
- Innovative Solutions: Big data enables the development of new products and services tailored to meet the evolving preferences of consumers.
In this section, we delve into three significant areas where big data analytics is making a significant impact: healthcare, finance, and marketing. Each of these fields showcases unique benefits and considerations that arise from the integration of big data technologies.
Healthcare Innovations
The healthcare sector has seen profound transformations with the adoption of big data analytics. From patient care to operational efficiency, the applications here are both groundbreaking and essential. The ability to analyze patient data in real-time can enhance treatment outcomes and streamline operations.
For example, predictive analytics assists healthcare providers in foreseeing potential outbreaks or emergency situations by examining data patterns. This was especially evident during the COVID-19 pandemic, where analytics played a crucial role in tracking infection rates and resource allocation.
Moreover, the integration of wearables and mobile health applications has led to vast amounts of health data that, when analyzed, can provide insights into individual health trends.
"With data, we can not only treat illnesses better but also prevent them. Itās a game changer for how we think about health management."
Financial Sector Applications
In finance, big data analytics not only helps in risk management but also enhances investment strategies and customer service. Financial institutions are now making data-driven decisions, which can lead to improved profit margins and customer satisfaction.
Risk assessment tools analyze vast financial histories to predict defaults and manage portfolios more effectively. Additionally, algorithmic trading has become a cornerstone of modern finance; automated systems analyze market trends in seconds, allowing for rapid and informed trading decisions.
Another interesting aspect is fraud detection. Using advanced analytics, institutions can identify unusual patterns that may signal fraudulent activity, enabling them to react quickly.
Marketing and Consumer Insights
Lastly, big dataās impact on marketing and consumer behavior is profound. Marketers today can collect data from multiple channelsāsocial media, web traffic, in-store purchasesāto create comprehensive consumer profiles.
These analytics help businesses understand buying patterns and preferences, enabling targeted advertising campaigns that resonate with specific demographics. For instance, using customer segmentation models, companies can tailor their messaging to match the interests of different consumer groups.
In Summary
The applications of big data analytics extend well beyond the confines of a single industry. From improving patient outcomes in healthcare to enhancing investment strategies in finance and refining marketing tactics, the scope is vast and varied. Understanding these implementations not only reveals the potential of big data analytics but also prepares professionals to navigate the increasingly data-centric world.
Challenges in Big Data Analytics
In the ever-evolving landscape of big data, navigating the casual complexities presents a host of challenges that can significantly impact the effectiveness of analytics efforts. While the prospects of harnessing vast troves of information can seem enticing, itās crucial to tread carefully through the minefield of potential issues that could arise. Understanding these challenges allows organizations to equip themselves with strategies for better data management, informed decision-making, and ethical considerations.
Data Privacy Issues
When it comes to big data, one cannot overlook the paramount concern of data privacy. As organizations collect and analyze personal dataāfrom browsing habits to purchasing historiesāthe risk of compromising sensitive information escalates. This concern is not just a mere footnote; it can lead to profound consequences if not managed correctly.
With laws like the General Data Protection Regulation (GDPR) in Europe, the landscape of data privacy is becoming more stringent. Companies now face the daunting task of ensuring that they not only comply with local regulations but also maintain consumer trust. Failing to do so can result in hefty fines and reputational damage. For example, in 2021, Facebook faced intense scrutiny over its handling of user data, which impacted user confidence and opened a floodgate of legal challenges. Organizations must tread carefully, ensuring robust protocols around data collection and defining clear consent processes to mitigate such risks.
Data Quality Concerns
Data quality is another pressing challenge that those in big data analytics face. Garbage in, garbage out, as the saying goes, captures this issue perfectly. When making decisions based on inaccurate or incomplete data, organizations risk misguided strategies and costly mistakes. Poor data quality can arise from various sources: manual entry errors, inconsistent formats, or outdated information can all degrade the integrity of the data set.
To address these pitfalls, extensive data validation measures are essential. This includes clear standards for data entry, ongoing audits for consistency, and employing data cleansing tools or techniques. For instance, using technologies like data profiling can help identify anomalies before they wreak havoc on analysis processes. Organizations that prioritize data quality not only improve the reliability of their insights but also enhance their overall operational effectiveness.
Skill Gaps in the Workforce
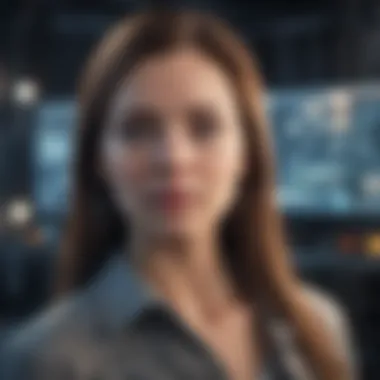
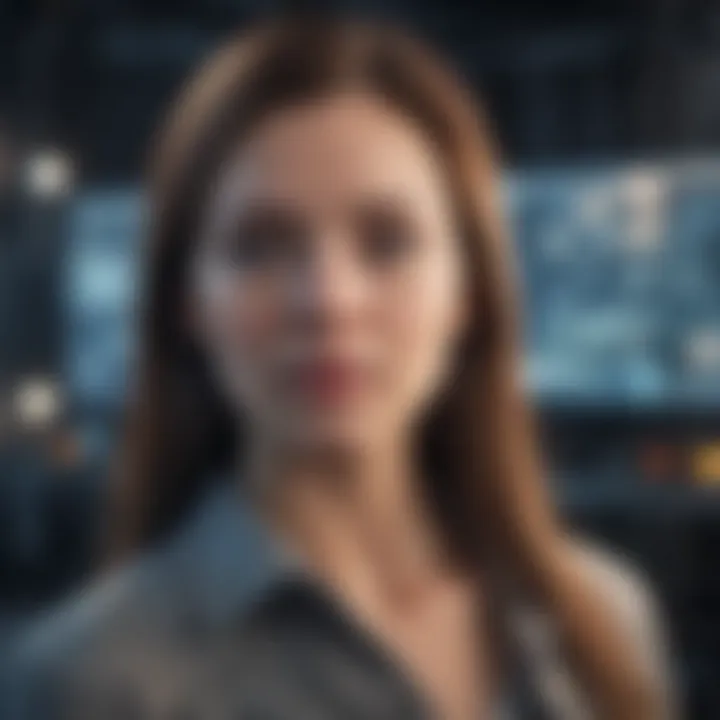
The rapid pace of technological advancement has led to a noticeable disconnect in skills among the workforce. Despite the increasing demand for professionals skilled in data analytics, a considerable gap persists in the labor market. Many graduates emerge with foundational knowledge but lack the practical expertise required to handle the complexities of big data. This gap can severely hinder an organization's ability to leverage analytics effectively.
Organizations in search of data-savvy individuals are often left scrambling to find suitable candidates. To bridge this divide, companies can foster a culture of continuous learning by investing in training programs, partnerships with educational institutions, and encouraging employees to upskill. For example, offering workshops on emerging analytical technologies or certifications in data management can significantly enhance skill levels within the organization. Moreover, leveraging mentorship programs can also help nurture a new generation of data analysts.
"The challenges of big data analytics must not be sidelined but embraced as opportunities to innovate and grow instead."
By addressing these challenges head-on, companies can enhance their analytical capabilities and position themselves ahead of competitors in the data-driven landscape.
Ethical Considerations in Big Data
The realm of big data analytics does not exist in a vacuum; it intertwines itself deeply with ethical principles that govern data usage, privacy, and accountability. As the volume of data at our fingertips expands, so too does the responsibility that comes with it. The ethical considerations in big data play a crucial role in ensuring that the benefits of data analytics are harnessed without infringing on individual rights or societal norms. This section explores the critical elements of responsibility in data usage and managing bias, which are pivotal to navigating the ethical landscape.
Responsibility in Data Usage
In todayās data-driven world, the concept of responsibility in data usage takes center stage. Organizations must not only handle data with care but also possess a moral compass guiding their actions. A few significant components of this responsibility include:
- Transparency: Organizations should provide clear information regarding data collection methods, purpose, and the intended usage of data. It fosters trust and allows individuals to make informed decisions about their own information.
- Informed consent: Obtaining consent from individuals before collecting their data should be standard practice. This means educating users about what they are consenting to, ensuring they are not in the dark about how their data will be employed.
- Data minimization: Collect only the data necessary for the intended purpose. Avoiding excess prevents misuse and reduces potential harm to individuals.
"With great power comes great responsibility. In the world of big data, this adage rings truer than ever."
By fostering a culture of accountability, organizations not only protect individuals but also build a sustainable framework for future data analytics practices.
Managing Bias in Data
Bias in data is a double-edged sword. If uncontrolled, it can lead to unintended discrimination, perpetuating stereotypes, or yielding misleading insights. Thus, managing bias in data emerges as an ethical imperative. Key strategies for addressing bias include:
- Diverse data sources: Utilize a wide range of data that reflects the diversity of the real world. This approach minimizes the risk of reinforcing existing biases.
- Bias detection tools: Employ technologies designed to identify and rectify bias in datasets. Regular assessments should be part of the data management lifecycle.
- Ethical training: Equip teams with knowledge about bias and its implications. An informed workforce is better prepared to recognize and mitigate biases before they cause harm.
The fight against bias is ongoing and requires constant vigilance. Addressing bias not only enhances the integrity of data analytics but also contributes to more equitable outcomes in decision-making.
In summary, the ethical considerations in big data analytics are paramount. By approaching data usage responsibly and actively managing bias, organizations can ensure their practices align with moral standards. As big data continues to reshape industries, embedding ethics in the analytical process becomes not just beneficial but essential.
Future Trends in Big Data Analytics
The field of big data analytics is rapidly evolving, presenting new opportunities and imposing unique challenges. Understanding future trends is crucial for stakeholders ranging from businesses to researchers and students alike. These trends give us a glimpse into not just whatās possible, but also how to strategically leverage data for enhanced decision-making and operational effectiveness.
Artificial Intelligence Integration
As artificial intelligence becomes an integral part of data analytics, the potential for generating insights multiplies. AI algorithms can quickly sift through massive datasets, recognizing patterns that may not be evident to human analysts. This is not just a hobbyhorse; businesses that deploy AI combined with big data are already experiencing significant advantages. They gain faster processing times, predictive capabilities, and a deeper understanding of customer behavior.
Benefits to Embrace
- Enhanced Predictions: Businesses can forecast trends more accurately by analyzing historical data in tandem with current behaviors. This proactive approach can significantly outpace competitors stuck in traditional analytics models.
- Automation of Analytics Processes: AI automates repetitive tasks, thereby freeing analysts to focus on strategic initiatives. For instance, platforms like IBM Watson provide organizations tools to automate report generation, making insights accessible almost in real-time.
- Personalized Experiences: From Netflix recommending shows to Amazon suggesting products, AI personalization drastically improves customer engagement and retention.
However, despite these advantages, the integration of AI in data analytics also raises concerns. Issues surrounding data ethics, especially regarding privacy and bias, need cautious navigation. As we incorporate more AI-driven processes, scrutiny of data sources becomes imperative, highlighting the importance of responsible data usage.
Real-Time Analytics Capabilities
Real-time analytics is another avenue that is fast gaining traction in the big data landscape. In a world where every second counts, organizations can no longer afford to wait for insights to trickle in. Technologies that facilitate real-time data processing are changing the game, offering data-driven insights at the speed of thought.
The Impact of Real-Time Data
- Immediate Decision-Making: For sectors like finance, where market fluctuations occur by the minute, real-time analytics is not just beneficial; it's essential. Companies are employing advanced dashboard tools to monitor market trends and react swiftly.
- Operational Efficiency: In industries such as supply chain management, real-time data allows for immediate adjustments based on consumption patterns, significantly reducing waste.
- Improved Customer Interaction: Retailers are using real-time data to gauge customer sentiment and engagement levels, adjusting promotions and product displays even while customers are in-store.
Real-time analytics transforms the decision-making landscape, allowing companies to adapt and respond to market dynamics without delay.
Real-time capabilities, however, also demand robust infrastructure. Organizations must consider the investment in technology and the potential need for skilled personnel to interpret and act upon dynamic data streams. Striking a balance between agility and stability is essential for implementing such systems effectively.
Closure
In an era where the deluge of data is unprecedented, the topic of Big Data Analytics holds paramount importance. This article has journeyed through the intricate realm of information science, shedding light on both foundational concepts and various implications that come with it. The significance of mastering big data cannot be understated, as it impacts not only technological advancement but also broader societal change.
Summation of Insights
Throughout our exploration, several key points have emerged. First, the transformative power of big data has become evident, as sectors including healthcare, finance, and marketing tap into this resource to refine their operations and make informed decisions. By harnessing such analytics, organizations are not merely keeping pace; they are frequently setting the benchmark for innovation. A notable insight is how predictive and prescriptive analytics are reshaping strategies, allowing businesses to forecast outcomes and chart a more precise path forward.
Moreover, this journey has underscored the challenges inherent in big data analytics. We have addressed concerns surrounding data privacy and the quality of data insights. Addressing these issues is critical, as stakeholders must remain vigilant in ensuring ethical practices in their data usage. In doing so, organizations can create a more trustworthy environment that ultimately benefits everyone.
Incorporating advanced tools and technologies, organizations can amplify their capacity for real-time analytics, which leads to more agile response mechanisms to shifting market dynamics. By adopting these methods, industries can surge ahead of competitors and remain relevant in a fast-moving world.
Call for Continued Research
The field of big data analytics is far from static; it is in a constant state of evolution. Consequently, continuous research is essential for keeping pace with emerging trends, such as the integration of artificial intelligence. As we dive deeper into artificial intelligence's capabilities, researchers must examine how these technologies can enhance data processing and interpretation. This intersection is ripe for exploration and promises substantial advancements in decision-making capabilities.
Furthermore, there is a pressing need to address the skill gaps in the workforce. As big data analytics becomes more integral to businesses, training programs and educational initiatives must evolve in tandem to equip future professionals with the necessary expertise. Ongoing research into teaching methodologies could offer insights into how best to prepare students and employees for this dynamic field.
Ultimately, our journey through big data analytics reveals an expansive landscape full of possibilities. Future research will not only illuminate paths to enhanced understanding and application of big data but will also drive the innovation needed to tackle todayās complex challenges.