Data Analytics Transforming Healthcare Practices
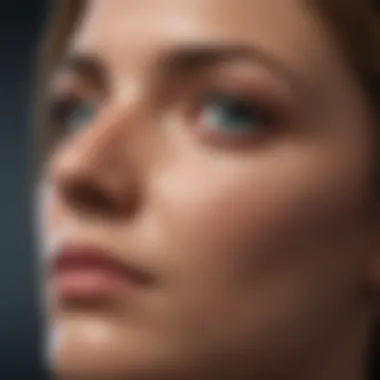
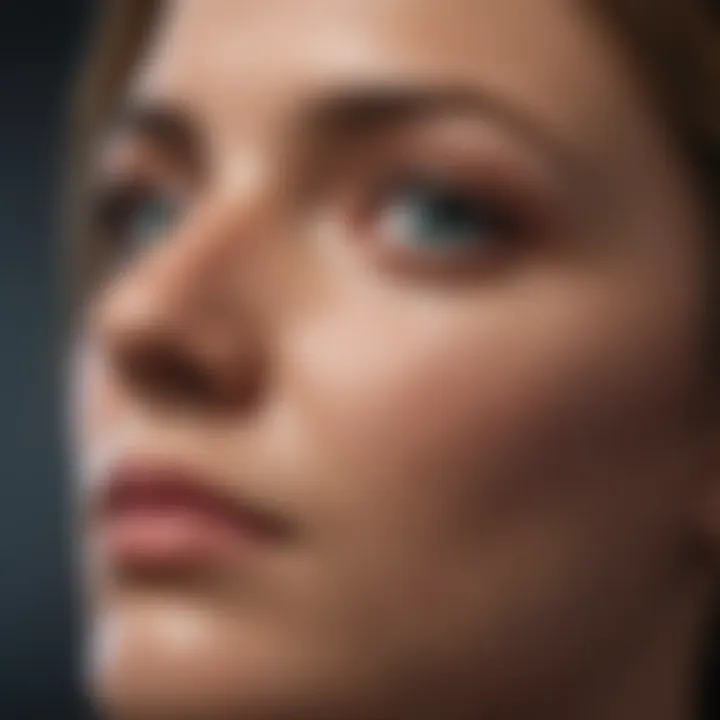
Intro
In today's dynamic world, data analytics has become a cornerstone in various industries, particularly in healthcare. The capability to extract meaningful insights from vast amounts of data not only enhances patient outcomes but also streamlines operational processes. The intersection of data and healthcare is no longer just a topic of discussion among professionals; it has transformed into an essential practice for improving the quality of care delivered at all levels.
The use of data analytic techniques in healthcare touches on everything from patient management and treatment design to financial and operational efficiencies. Specialists in healthcare are looking at data analytics as a means to inform their decisions and transform their practices. But it's not all sunshine and rainbows; ethical considerations and data privacy issues emerge as significant hurdles that demand attention.
This article will explore the various applications of data analytics in healthcare, offering keen insights into successful case studies that demonstrate its far-reaching implications. By dissecting the applications and potential future paths of this field, we aim to inform an audience that includes students, researchers, educators, and seasoned professionals about the vital role of analytics in revolutionizing healthcare.
Research Highlights
Overview of Key Findings
Data analytics stands out in healthcare for its transformative capabilities. Our exploration will underline several key findings:
- Enhanced Patient Care: Predictive analytics enables healthcare providers to tailor treatments based on individual patient data, improving outcomes significantly.
- Operational Efficiency: Real-time data analytics helps hospitals streamline workflows, reducing wait times and increasing patient satisfaction.
- Research Advancements: Leveraging large datasets, researchers can identify trends that inform clinical studies and public health initiatives.
These findings paint a clear picture of how critical data analytics has become in shaping modern healthcare practices.
Significance of the Research
Understanding the implications of data analytics in healthcare has far-reaching significance. It is not merely about numbers and statistics; it’s about enhancing the overall patient experience and operational workflows in healthcare. As we delve deeper into the applications of data analytics, the goal is to show not only how these tools are being used but also the ethical considerations that come with them. Recognizing these factors is crucial in shaping a future where data serves humanity rather than the opposite.
"Data analytics in healthcare is like a compass; it guides us through the vast sea of information to find the path that leads to better care."
Through a synthesis of the information presented and a look at future trajectories, we aim to equip our readers with knowledge that is both impactful and actionable.
Prologue to Data Analytics in Healthcare
The healthcare landscape is rapidly evolving, primarily driven by the transformational power of data analytics. Understanding this domain is paramount in today’s world, particularly as we navigate complexities in patient care, service delivery, and health outcomes. The integration of data analytics into healthcare not only enhances decision-making processes but also offers innovative approaches to improve overall health services.
Definition and Scope
Data analytics in healthcare refers to the systematic computational analysis of data related to patient care, treatment outcomes, operational efficiency, and public health trends. This encompasses a wide range of techniques and methodologies, from basic descriptive analytics to advanced predictive and prescriptive analytics. The scope is vast, impacting various aspects including but not limited to:
- Clinical decision support: Helping healthcare professionals make informed choices about patient care.
- Resource management: Streamlining operations to ensure optimal use of facilities and staff.
- Patient engagement: Analyzing behaviors and preferences to foster a stronger relationship between providers and patients.
With the increase in electronic health records and health information exchanges, the volume of data available has skyrocketed. This influx allows for a more granular understanding of patient needs and healthcare pathways, which ultimately leads to tailored healthcare solutions.
Historical Context
To appreciate the evolution of data analytics in healthcare, it’s crucial to look back at its roots. The journey began with rudimentary data collection—think paper records and simple statistical analysis. As technology burgeoned, healthcare data management shifted significantly.
The introduction of computers in the 1960s and 1970s laid the groundwork for patient information systems. A landmark moment occurred with the implementation of electronic health record systems in the late 1990s and early 2000s. These systems not only digitized patient information but also paved the way for comprehensive data analytics practices.
Today, the use of machine learning and artificial intelligence is steering this field in new directions. For instance, predictive modeling looks at past health data to forecast future outcomes, an approach that is becoming increasingly prevalent. The healthcare sector is thus witnessing a data revolution, where insights from analytics can potentially save lives and improve system efficiencies.
Applications of Data Analytics in Patient Care
The role of data analytics in patient care is not merely a buzzword in today’s healthcare ecosystem; it’s becoming a cornerstone that shapes how healthcare services are delivered. This section will dive into the transformative impact of analytics—delving into tangible benefits, practical considerations, and specific innovations that make a difference in patient outcomes. The ability to harness data effectively allows healthcare professionals to anticipate needs, tailor treatments, and improve overall care experiences.
Predictive Analytics for Risk Assessment
Predictive analytics stands as a powerful tool in the arsenal of healthcare providers, surfacing potential risks before they materialize. Using historical data and sophisticated algorithms, healthcare systems can assess patients’ likelihood of developing complications or serious conditions. For instance, consider the patient who has a history of cardiovascular issues. By identifying patterns from similar cases, predictive models can flag this patient for targeted interventions, oftentimes before symptoms appear. This proactive approach can lead to earlier treatment, which in turn can save lives.
Furthermore, predictive analytics can extend beyond individual patients. Hospitals can identify trends in specific populations, which allow public health officials to allocate resources more efficiently. As one can imagine, having a pulse on potential risks also empowers the clinical staff to be more strategic and less reactive, ultimately improving patient safety.
Personalized Treatment Plans
Data analytics is reshaping how treatment plans are created, transitioning from a one-size-fits-all method to a more personalized approach. Each patient is unique, carrying their own medical history, genetic factors, and lifestyle choices. By utilizing comprehensive data-driven insights, healthcare providers can tailor their treatments to match the distinct characteristics of each patient.
For example, the emergence of pharmacogenomics—the study of how genes affect a person’s response to drugs—enables much finer precision in prescribing medications. When a doctor can determine a patient’s genetic makeup through data analysis, they can choose medications that are more likely to be effective and less likely to cause adverse reactions. This not only enhances treatment efficacy but also boosts patient adherence to medications, ultimately leading to better health outcomes.
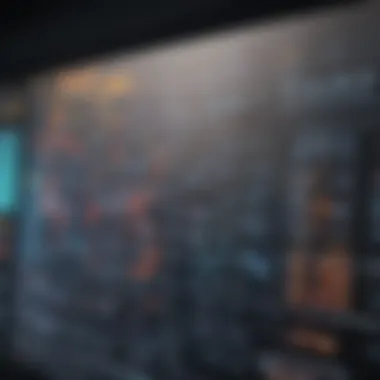
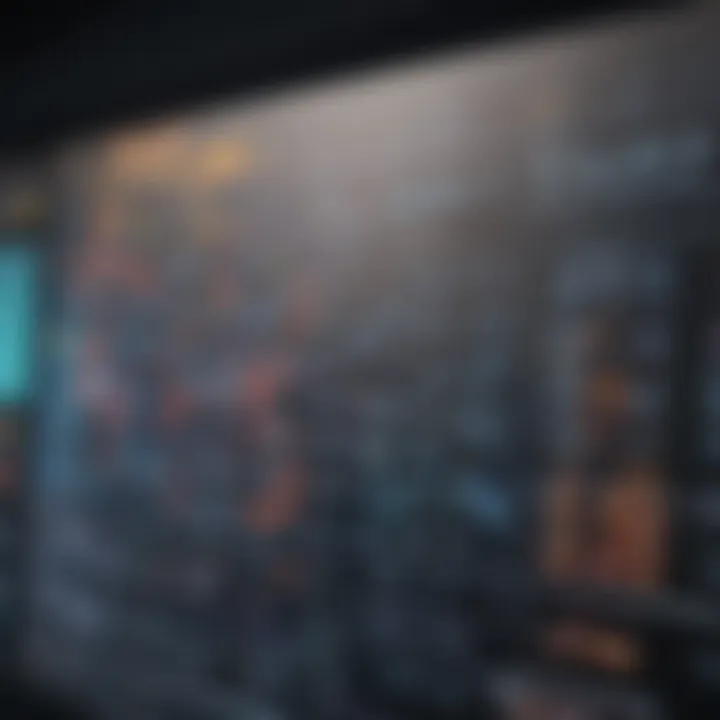
Real-Time Monitoring and Alerts
With the advent of wearable technology and advanced monitoring systems, real-time data collection has entered the healthcare landscape. Imagine a situation where a patient with chronic heart disease is equipped with a smartwatch that continuously monitors their heart rate and blood pressure. Data analytics plays a crucial role here, as it analyzes the incoming data on the fly, detecting anomalies that may indicate a health crisis.
When such a deviation occurs, alerts can be triggered automatically, notifying both the patient and their healthcare team. This kind of immediate feedback can be critical; a timely intervention may prevent hospital admissions or even save a life. Hospitals now utilize similar systems to monitor various vital stats of patients, integrating data from different sources to establish comprehensive profiles that inform clinical decisions at lightning speed.
"Real-time monitoring doesn’t just empower patients; it also enriches physician insights, making proactive care a reality rather than a goal."
Data Analytics in Operational Efficiency
The realm of healthcare is akin to a bustling city, where efficiency can often be the deciding factor between life and death. Data analytics is transforming this city into a well-oiled machine, ensuring that each cog works in harmony with the others. This section elucidates how analytics serves as a linchpin for operational efficiency, spotlighting its pivotal role in optimizing resources, streamlining administrative tasks, and enhancing workflow efficiency.
Optimizing Resource Allocation
In healthcare facilities, resources – whether human, technological, or financial – are perpetually parachuting under constraints. Data analytics offers a compass in these tumultuous seas, guiding institutions to allocate resources smartly. By analyzing historical data and current trends, healthcare providers can pinpoint peak patient admission times and allocate staff accordingly. For example, a hospital might find that patient volumes spike during flu season, enabling them to ramp up staffing in anticipation, which ultimately leads to improved patient satisfaction and reduced wait times.
Moreover, predictive analytics is invaluable here. It can forecast supply needs for medication and equipment based on past usage patterns. Imagine a surgical unit that uses past patient data to ensure that the right tools and materials are available for the upcoming week’s surgeries, reducing waste and costs significantly.
Streamlining Administrative Processes
Bureaucratic hang-ups in healthcare can feel like navigating through molasses. Administrative processes are often bogged down by redundant paperwork and inefficient practices. Here, data analytics acts as a lighthouse, illuminating pathways to streamline these convoluted systems. By deploying software systems that are driven by data, hospitals can automate billing processes, manage appointment scheduling, and optimize patient flow.
Consider the impact of an efficient patient management system that integrates with electronic health records. It can reduce the time staff spends on paperwork by allowing real-time updates and access to patient information. As a result, this frees up human resources to engage in more meaningful interactions with patients, fostering a sense of care while also enhancing operational throughput.
Enhancing Workflow Efficiency
Every healthcare professional knows that time is of the essence. Just like a symphony needs every instrument to play their part seamlessly, so does a healthcare institution need its workflows to flow without a hitch. Through data analytics tools, workflows can be studied and improved in real time. By mapping out the journey of a patient through the healthcare system, from admittance through discharge, organizations can identify bottlenecks.
Deploying analytics not just provides the ability to review historical performance, but also enables continuous monitoring. For instance, if a particular department consistently shows delays in processing, stakeholders can dig deeper into the data to uncover the root causes. This might pave the way for restructuring processes or redistributing personnel, thus ensuring a smoother patient experience.
"Healthcare systems that adopt data-driven practices will not only save time and resources but also improve the quality of care delivered."
By integrating analytics at this level, patients experience shorter wait times, staff feels less overwhelmed, and management can rest assured knowing they are incrementally improving their operations.
Ultimately, the integration of data analytics within operational efficiency of healthcare is a game changer. No more shooting in the dark; instead, it’s about taking calculated steps backed by evidence. As the healthcare landscape evolves, those who harness the power of analytics will find themselves ahead of the curve.
Data Analytics in Public Health Initiatives
Data analytics plays a pivotal role in shaping public health initiatives by fostering informed decision-making and targeted interventions. This realm epitomizes the versatility of data analytics, as it can be employed across numerous applications—from tracking disease outbreaks to driving public health campaigns. By tapping into diverse datasets, healthcare professionals glean insights that are nothing short of revolutionary for safeguarding communities.
In essence, the importance of data analytics in public health lies in its capability to:
- Improve Health Outcomes: By analyzing health trends, professionals can better understand and anticipate the needs of the population.
- Optimize Resource Allocation: Targeting interventions where they are needed most ensures that funds and resources are utilized efficiently.
- Enhance Preventive Measures: Analytical insights can aid in devising strategies to prevent diseases before they proliferate.
Epidemiological Studies
Epidemiological studies rely heavily on data analytics to explore the distribution and determinants of health-related states within populations. These studies often synthesize vast amounts of data from medical records, healthcare surveys, and case reports to form a comprehensive picture of public health trends.
To ensure that this data is valuable, researchers utilize statistical tools to:
- Identify risk factors associated with various diseases.
- Forecast future outbreaks based on historical data patterns.
- Inform public health policies that are evidence-based, thereby ensuring that resources are allocated effectively.
For instance, by scrutinizing historical data on smoking rates and lung cancer incidences, public health officials can tailor anti-smoking campaigns with the aim of reducing lung cancer prevalence in certain demographics. The multiply effects of such tailored campaigns underscore the necessity of data-driven epidemiological studies.
Disease Surveillance Systems
Disease surveillance systems are another cornerstone of public health initiatives. These systems collect, analyze, and interpret health data to monitor the occurrence and spread of diseases in real time. By harnessing data analytics, health agencies can:
- Detect outbreaks and trigger rapid responses to mitigate further transmission.
- Provide timely information for the development of vaccines and treatments.
- Foster collaborative efforts between public and private sectors for more comprehensive healthcare strategies.
An example of successful disease surveillance is the FluView system in the United States, which uses data analytics to gauge flu activity across the country. By compiling information from hospitals, laboratories, and doctors, the CDC can provide timely updates and inform citizens about precautions and preparedness measures.
Covid-19 Response Analytics
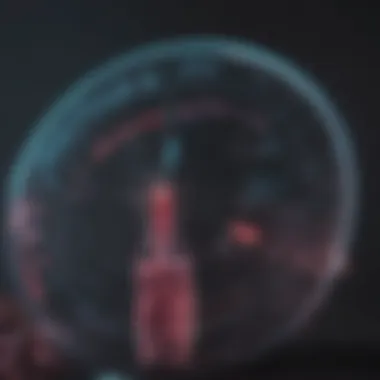
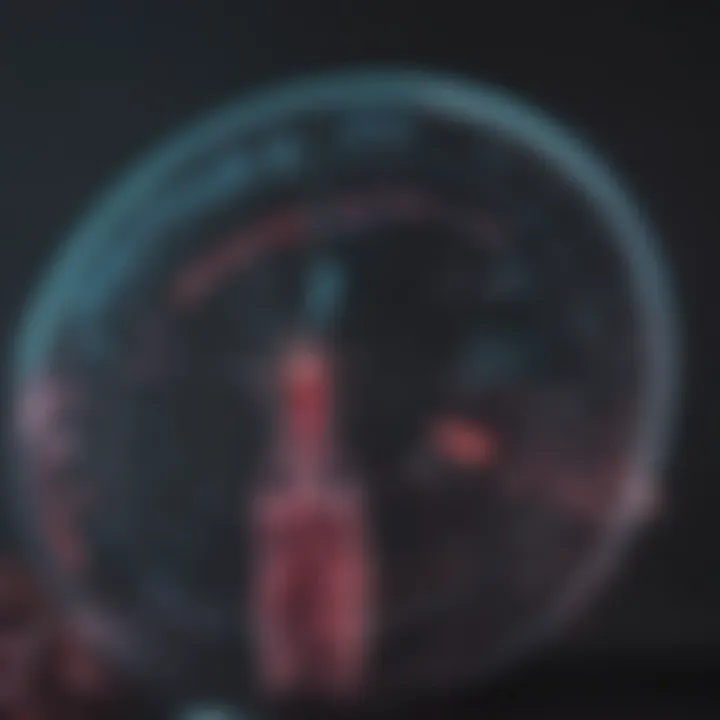
The Covid-19 pandemic has vividly illustrated the critical importance of data analytics in public health initiatives. Health organizations faced unprecedented challenges, and leveraging analytics proved to be a game changer. Key analytics employed during this crisis include:
- Case Tracking: By analyzing case data, public health officials could identify hotspots and implement localized health measures.
- Vaccination Rollouts: Data analytics enabled the efficient administration of vaccines by optimizing supply chains and identifying priority groups based on risk factors.
- Public Sentiment Analysis: Analyzing social media trends surfaced attitudes towards public health measures, guiding communication strategies to address public concerns and misinformation.
"A well-informed society can mobilize swiftly; empowered by data analytics, we can combat health threats effectively."
Case Studies of Data Analytics Impact
Analyzing the impact of data analytics in healthcare isn't just a numbers game; it's about real change in practice and patient outcomes. Case studies bring to light how this analytical approach reshapes the healthcare landscape. Each story reflects practical applications and significant benefits, coupling theory with tangible results. This exploration is essential for educators, researchers, and healthcare professionals alike, as it illustrates crucial shifts that can change the nature of care delivery.
Case Study: Predictive Algorithms in Diabetes Management
One of the more compelling examples of data analytics is found in diabetes management. Predictive algorithms can sift through heaps of patient data for patterns that might otherwise go unnoticed. For instance, using machine learning, clinicians can identify which patients are at greater risk for complications by examining their historical health records, lifestyle choices, and even geographical data.
The results speak volumes: patients at higher risk are flagged for intervention long before they show any troubling symptoms. This proactive approach allows for tailored treatment options, reduced complications, and ultimately, improved patient outcomes. The savings in both health costs and emotional toll on patients—combined with the benefit of timely interventions—underscore the importance of predictive analytics in chronic disease management.
Case Study: Reducing Readmission Rates
Readmission rates are a persistent issue in healthcare, with significant financial implications. Through data analytics, hospitals are using sophisticated models to analyze patient data and pinpoint the factors leading to unnecessary readmissions. This data can include everything from the patient’s clinical history to their discharge instructions and even social determinants of health.
By identifying risk factors, health systems can develop targeted discharge plans that address patients' unique needs. For example, hospitals can partner with community organizations to provide follow-up care and support for patient navigation, making sure patients have what they need to stay healthy post-discharge. When hospitals successfully implement these data-driven strategies, readmission rates can drop significantly, illustrating a clear win-win situation: better health outcomes for patients, and significant cost savings for healthcare providers.
Case Study: Enhancing Patient Safety through Analytics
Patient safety stands as a cornerstone of healthcare quality, and data analytics plays a vital role in this regard. Hospitals are now adopting analytics tools to predict potential safety incidents by examining trends in medication errors, infections, and other adverse events. For example, data from previous patient records can reveal patterns that signal increased risk levels in certain departments.
By identifying these risk patterns, healthcare facilities can make proactive adjustments. For instance, they might increase training for staff in higher-risk areas or implement more rigorous monitoring of patient vitals. According to studies, hospitals that leverage these analytical approaches can reduce the incidence of adverse events significantly. Ultimately, this not only fosters a safer environment for patients but also boosts staff morale and trust in the system.
"In an era where data reigns supreme, these case studies show that the right analytical tools can translate complex information into actionable insights that save lives."
Each of these cases illustrates how data analytics does not merely enhance operational efficiency, but also reveals its true potential as a tool for improving patient care, safety, and overall outcomes. As the healthcare industry moves forward, these case studies provide a roadmap for integrating data analytics into everyday practice.
Challenges in Implementing Data Analytics
The integration of data analytics in healthcare faces significant hurdles that cannot be overlooked. While the potential advantages are vast, understanding these challenges helps in strategizing solutions and ensuring efficient implementation. The journey into data analytics may seem straightforward, but navigating through the complexities requires attention to specific elements such as data privacy, system compatibility, and human resources.
Data Privacy and Security Concerns
Data privacy stands as one of the most pressing issues in healthcare analytics. With sensitive patient information at stake, any breach can have severe repercussions. According to regulations like HIPAA in the U.S., patient data must be handled with utmost care. Organizations need to adopt rigorous security measures to prevent unauthorized access and ensure compliance. Data encryption, access controls, and regular audits are essential.
- Key points to consider:
- Patient confidentiality must be the priority.
- Regular training on data security for staff is crucial.
- Implementing advanced technologies like blockchain could enhance data integrity.
When patients are aware that their data is protected, they may be more willing to share information, leading to better analytics outcomes.
Integration with Legacy Systems
Many healthcare facilities still operate on outdated systems that were not designed for modern data analytics. Integrating new analytical tools with legacy systems can be akin to fitting a square peg in a round hole. Often, these older systems lack the flexibility needed to cooperate with newer technologies, resulting in inefficiencies and loss of valuable data insights.
- Complications in integration may include:
- Data silos that prevent comprehensive insights.
- High costs of overhauling legacy systems.
- Resistance from staff accustomed to older technologies.
Transitioning gradually while maintaining operational efficiency is essential. Phased implementation might be a way to bridge the gap without overwhelming the staff or resources.
Staff Training and Resource Allocation
The human element in data analytics cannot be emphasized enough. Even the most advanced systems are useless if the staff isn’t trained adequately to use them effectively. Investing in education and resources is as critical as the technology itself. Healthcare organizations must prioritize staff training to ensure they understand data tools and methodologies.
- Training initiatives should focus on:
- Tailored programs for different staff levels.
- Continuous education to keep pace with evolving technologies.
- Developing a culture of data literacy within the organization.
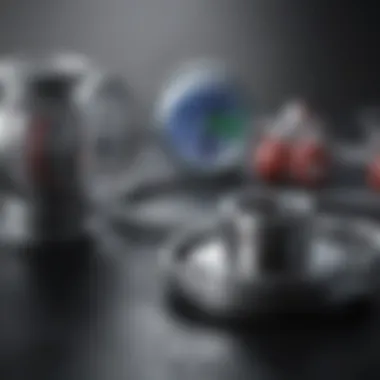
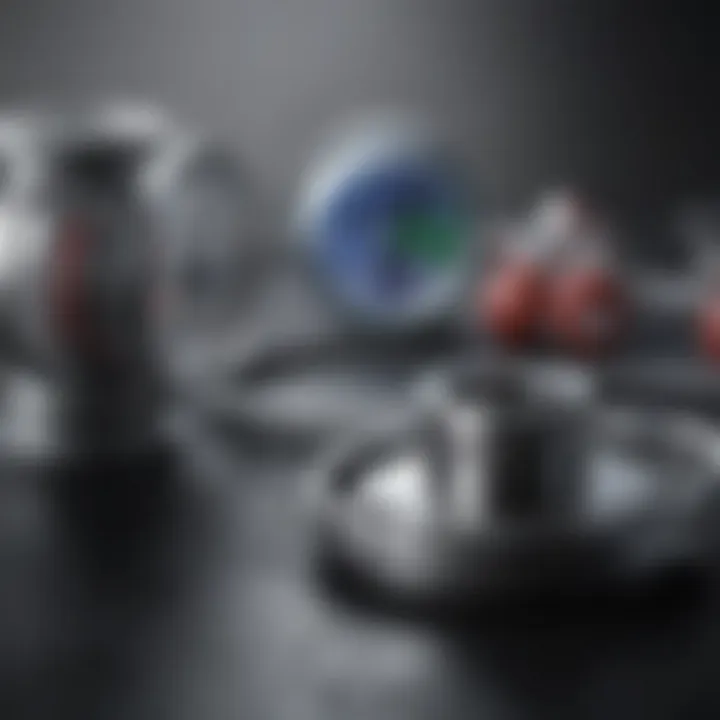
Also, the proper allocation of resources to train personnel directly impacts the long-term success of data analytics. Without this investment, the full benefit of analytics may never be realized, and the organization could fall behind competitors who prioritize staff education.
"In a world where data is the new oil, ensuring the workforce is equipped to refine it is essential for success in healthcare analytics."
Understanding and addressing these challenges is crucial for healthcare institutions aiming to leverage data analytics effectively. Only by mitigating these issues can the healthcare sector truly harness the power of data to improve patient care and operational efficiency.
Future Trends in Healthcare Data Analytics
The landscape of healthcare is rapidly evolving, and tapping into data analytics is poised to be a transformative force. Future trends in healthcare data analytics not only reshape how healthcare practitioners and institutions operate but also enhance patient outcomes through insightful data usage. It's essential for stakeholders, from researchers to educators, to grasp the full weight of these evolving trends.
One significant trend is the integration of artificial intelligence (AI) and machine learning (ML), which are now becoming cornerstones in data analytics. These technologies allow healthcare providers to analyze vast amounts of patient data, generating insights that can track disease progression and predict outcomes. But it’s not just about crunching numbers; it’s about improving the quality of care while reducing costs. Moreover, as AI continues to advance, the potential for personalized medicine increases, tailoring treatments specific to each individual’s genetic makeup.
"Data is the new oil. It’s valuable, but if unrefined it cannot really be used. It's only when it's refined that it can actually be used to create insights and innovations."
The rise of blockchain technology for data integrity is another crucial trend to watch. In a sector often hampered by data silos and privacy concerns, blockchain offers a transparent and secure method for storing health information. By providing a decentralized ledger, patient records can be immutably shared across systems, enhancing trust and enabling seamless interoperability between different healthcare entities. This is especially important in maintaining data integrity during critical care situations or in emergencies.
Expanding telehealth services is already showing its value, but with the infusion of robust analytics, it can reach new heights. Advanced analytics can optimize telehealth by analyzing data to identify physician workloads, patient demand trends, and treatment effectiveness. Furthermore, analytics can predict who might benefit from virtual care vs. in-person visits, ensuring that resources are allocated efficiently. Thus, telehealth can become more than just a stopgap solution; it can evolve into a comprehensive care model that better serves patient needs extending well beyond geographical constraints.
In summary, here are some key points concerning future trends in healthcare data analytics:
- Artificial Intelligence & Machine Learning: Enhances patient management through predictive analytics and personalized treatments.
- Blockchain for Data Integrity: Ensures secure and transparent sharing of health information, enhancing trust.
- Expanding Telehealth: Optimizes care delivery by analyzing patient data and predicting future healthcare needs.
Together, these trends signify a bright future for healthcare analytics, suggesting not merely an increment of efficiency, but a substantial evolution in how care and research intersect with data.
Ethical Considerations in Data Analytics
Data analytics has become a cornerstone in the healthcare industry, drastically transforming how care is delivered and operations are managed. Yet, amid its many advantages, ethical considerations in data analytics cannot be overlooked. As the use of large datasets grows to inform everything from patient treatments to administrative decisions, it brings a host of ethical dilemmas that must be navigated carefully. This section delves into the intricacies of ethical considerations in data analytics, particularly focusing on informed consent and potential bias in data-driven processes.
Informed Consent and Data Usage
Informed consent is a fundamental principle of medical ethics, yet it becomes particularly nuanced in the realm of data analytics. Patients are often asked to share their medical data for research and analytical purposes, but understanding what this entails can be as clear as mud for many. It's crucial that individuals not only consent to the collection of their data but also grasp how it will be used, who will access it, and what measures are in place to safeguard it.
- Transparency is key. Patients should be made aware of the objectives behind data collection—whether it's to improve individual health outcomes, enhance service delivery, or contribute to broader medical research.
- Clarity around data sharing practices also matters. For instance, if data is to be anonymized and shared with third parties, patients should know this in advance.
- Revocation Rights should be communicated clearly, allowing patients the ability to withdraw their consent at any point, yet also understanding the implications of that decision.
"Informed consent is not merely a procedural formality; it embodies respect for patient autonomy."
This acknowledgment fosters trust, encouraging patients to engage actively in their healthcare while also protecting their personal data. The lack of adequate informed consent practices raises pressing issues about privacy breaches and potential exploitation of sensitive information. Without a robust framework supporting informed consent, the integrity of data analytics applications in healthcare could be compromised.
Bias in Data Analytics
Bias in data analytics is another significant ethical challenge. Data is often viewed through the lens of objectivity, but this assumption can be misleading. Various factors can introduce bias into healthcare data, leading to skewed analyses that could adversely affect patient outcomes.
- Historical Bias: If data is collected from historical precedences, it may reflect past inequalities in healthcare access, thereby perpetuating existing disparities. For example, algorithms designed to predict patient outcomes might disproportionately favor certain demographics over others, inflating accuracy for groups that have historically received more attention in studies.
- Sample Bias: The sample from which data is drawn can significantly influence analytics. If the patient sample does not represent the entire population, conclusions drawn might not be applicable universally. This raises questions about the fairness and equity of proposed treatments or programs.
- Algorithmic Bias: Algorithms often reflect the biases of their developers or the data they are trained on. For example, a decision-support tool created predominantly by a homogenous group may unintentionally reflect their biases, leading to unjust conclusions about patient care paths.
Addressing bias requires deliberate action.
- Ongoing Monitoring of algorithms and analytics processes are essential to identify potential biases.
- Diverse Input in the development of analytical tools can help bridge gaps in representation and ensure broader perspectives are included in the decision-making process.
- Transparency around analytics methodologies should be the norm, allowing for scrutiny and adaptation where necessary.
The End
In this article, the exploration of data analytics in healthcare has brought to light its growing significance and multifaceted applications. An increasingly digitized health system is one where data is not just collected but is utilized to enhance both patient outcomes and operational efficacy. By making sense of vast amounts of data, healthcare professionals can tailor interventions, foresee potential health risks, and streamline processes alike.
Summary of Key Findings
Delving into the research, we observed several key takeaways:
- Predictive Analytics empowers providers to anticipate potential complications, allowing for preventative measures before issues arise.
- Resource Allocation sees a striking improvement through data insights, helping healthcare institutions maximize their assets where they are needed most.
- Implementing Disease Surveillance Systems has fostered timely interventions in public health, particularly evident during the COVID-19 pandemic.
Furthermore, ongoing innovations in artificial intelligence and telehealth services show promising avenues for enhancing healthcare reach and eventually patient care.
"Quality healthcare relies on timely data. Analytics is the key that unlocks doorways to better health outcomes."
Implications for Future Research
The implications stemming from our findings present numerous avenues for future investigation:
- Ethical frameworks: As the use of data in healthcare continues to expand, defining clear ethical standards remains crucial.
- Bias mitigation: Future studies should scrutinize data sources for biases, ensuring that analytics leads to equitable health solutions for all demographics.
- Integration and interoperability of systems: Research aimed at effective communication between new and legacy systems can facilitate smoother transitions and wider data usage without disrupting ongoing practices.
To sum it up, as we look towards the future, it's vital to keep a keen eye on how data can be harnessed responsibly and effectively. The journey into the intertwined worlds of analytics and healthcare will continue, promising enhanced patient experiences and improved health outcomes while navigating a landscape that's ripe with ethical challenges.