Exploring Diverse Scientific Data Collection Methods
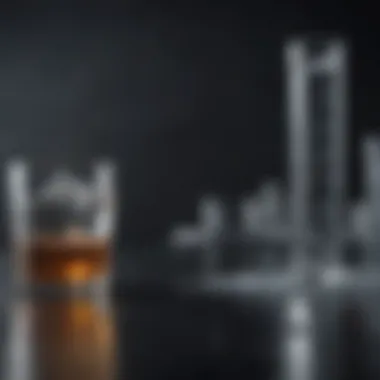
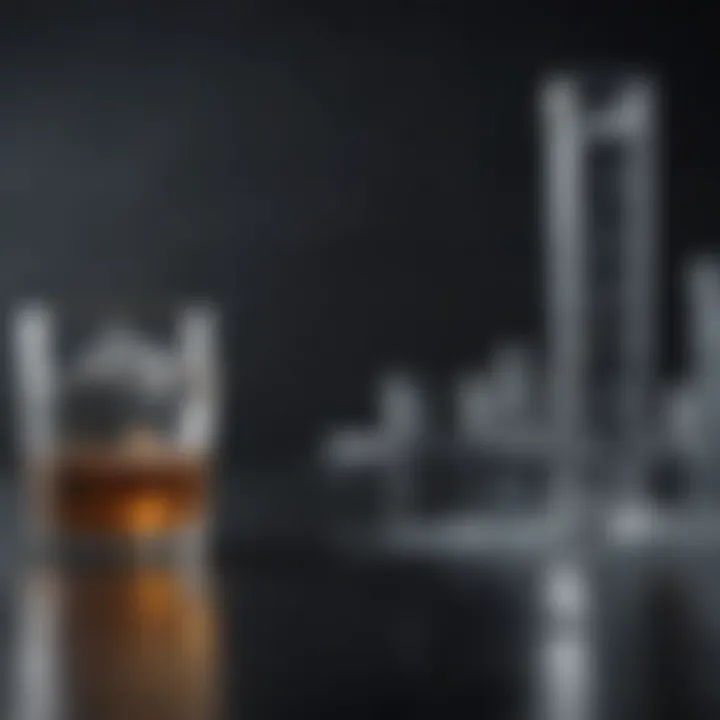
Intro
In the realm of scientific inquiry, the foundation upon which conclusions are drawn rests heavily on the methods employed to gather data. Selecting suitable data collection techniques is not merely a procedural formality but a critical aspect that shapes the integrity and outcome of research. An astute understanding of diverse approaches—qualitative, quantitative, and mixed methods—can significantly influence how researchers tackle pressing questions across various disciplines.
Advancements in technology and methodology have underscored the growing importance of rigorous data gathering in maintaining the scientific community's credibility. This article seeks to illuminate the intricacies of these collection methods by detailing their principles, advantages, and limitations. By examining the relevance of each approach in a rapidly shifting landscape, we aim to provide academics, industry professionals, and curious minds an insightful guide to navigating the vast sea of data collection strategies.
Research Highlights
Overview of Key Findings
- Diverse Methodologies: Each method of data collection brings a unique perspective, catering to different research objectives.
- Qualitative Insights: Emphasizes the exploration of complex phenomena through interviews and observations, luxurious in detail and context.
- Quantitative Rigor: Data collected via structured instruments such as surveys and experiments, pushing for clarity and measurable outcomes.
- Mixed Methods Synergy: A combination of both qualitative and quantitative approaches, acknowledging the richness of experience along with the precision of charts and statistics.
While different in their approaches, the ultimate goal remains consistent: to contribute substantively to the body of knowledge. According to various studies, understanding the strengths and weaknesses of each method can equip researchers with the insight needed to ensure their data reflects real-world conditions.
Significance of the Research
The significance of rigorous data collection methods extends beyond mere academic debate; it bears profound implications for everyday life and policy-making. Without reliable data, decisions made by governments, organizations, and individuals can lead to misinformed choices that might affect entire communities.
"In today's data-driven world, the ability to accurately collect and interpret data is vital for progress in any field."
Research integrity hinges on the selection of appropriate methodologies. By articulating their contributions, we can better appreciate the nuanced interplay between method, data, and the end results.
Original Research Articles
Summary of the Article
Author Contributions
Various contributors to this article have significant experience in diverse research fields. Their insights help underline the imperative nature of robust data collection methodologies in contemporary scientific discourse.
Preface to Scientific Data Collection
Understanding scientific data collection is akin to grasping the very foundation of research itself. It’s not merely a technical process; it's the bridge that links theoretical frameworks with real-world applications. Each method of data gathering plays a distinct role in uncovering truths about our environment, human behavior, and myriad other phenomena.
In this article, we will dive deep into various data collection methods, discerning how they function, why they matter, and their relevance to contemporary research. Selecting the right collecting technique isn’t just about choice; it influences the quality and integrity of the conclusions drawn. The accuracy of any scientific inquiry fundamentally lies in the data it rests upon. Therefore, embracing a thorough understanding of these methods can serve as an indispensable asset for researchers, educators, and practitioners alike.
Defining Data Collection in Science
Data collection in science encapsulates the processes of gathering, measuring, and analyzing accurate insights. This term often evokes images of surveys, experiments, and fieldwork; however, it extends far beyond these traditional views. At its core, data collection is the systematic approach to observe the world, gathering evidence to support or refute hypotheses.
The value of defining data collection is significant. It equips researchers with clarity, helping them understand the types of data needed for their investigations while also leading to more focused methodologies. Think of it as laying down the rules before a game. When researchers clarify what they seek to collect, it minimizes the likelihood of diverging paths or inaccuracies later on. Thus, in a tactical way, defining data collection helps to galvanize the study and its outcomes.
The Role of Data in Scientific Research
Data is the beating heart of scientific research, making its role indispensable. Whether qualitative or quantitative, the data acts as the evidence that drives the inquiry forward. It informs decisions, shapes theories, and provides context to findings. Without robust data collection, researchers might as well be sailing without a compass, lost in a sea of assumptions.
Several key functions of data in scientific research include:
- Informing Hypotheses: Data collection begins with questions, and the gathered data provides insights that help formulate and refine hypotheses.
- Guiding Methodology: Data influences the choice of methods used in research. Different data types may call for distinct analytical approaches, making data a guiding force.
- Supporting Conclusions: Ultimately, data backs the conclusions drawn in research. It stands as testimony to the validity of claims, allowing studies to have a solid foundation.
Whether it’s the raw numbers from a lab experiment or the nuanced stories revealed through interviews, data’s role cannot be overstated. The interplay between data and scientific inquiry is an ongoing conversation, one that grows richer as technology and methodologies evolve.
Qualitative Data Collection Methods
Qualitative data collection methods are fundamental in obtaining deep insight into complex phenomena. These methods focus on understanding human behavior, emotions, and experiences, adding a layer of richness to data that numbers alone often cannot provide. By delving into the "why" and "how" behind actions or beliefs, qualitative approaches help shape comprehensive narratives that quantitative data might overlook. Researchers often rely on these methods to investigate areas like community health, education practices, and social dynamics, where human interaction and perceptions play a significant role.
The real value in qualitative data collection lies in its ability to offer nuanced perspectives, helping to contextualize findings within specific environments or cultures. Whether through interviews, observation, or content analysis, every qualitative method lends its unique strengths to the research toolbox, making it indispensable in the quest for thorough scientific understanding.
Interviews as a Primary Method
Interviews stand out as a dominant method in qualitative data collection. They function as a bridge between researchers and participants, fostering a dialogue that is rich with detail and context.
Structured Interviews
Structured interviews are characterized by a fixed set of questions that guide the conversation. This consistency in questioning makes it easier to compare data across participants, ensuring the researcher gathers uniform information. In the realm of scientific data collection, structured interviews often serve as a strong foundation for producing quantifiable insights within qualitative frameworks.
One of the key characteristics of structured interviews is their efficiency. The clear-cut nature allows researchers to stay focused and minimizes tangents. This approach is beneficial when specific information is needed, making it a popular selection in fields like psychology or market research.
However, the rigid structure can also limit the depth of responses. Respondents might feel constrained, leading to missed opportunities for richer discussions, which is a notable disadvantage of using this method.
Unstructured Interviews
On the flip side, unstructured interviews offer a more flexible conversational style. Here, the focus is less on pre-defined questions and more on encouraging participants to share their thoughts freely. The goal is to explore complex topics where standard queries might stifle genuine responses. This method is often seen in ethnographic studies or sensitive subjects, where nuance is vital.

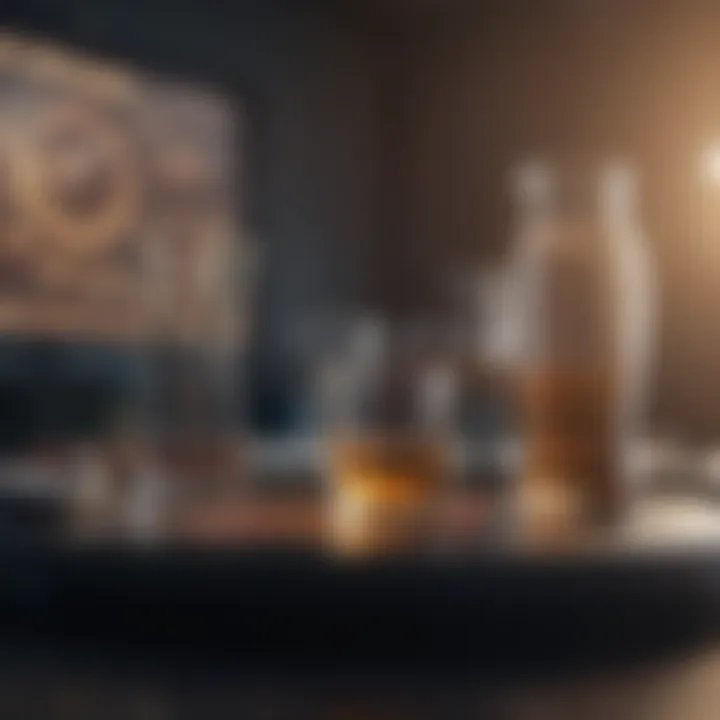
One reason unstructured interviews are favored in qualitative research is their ability to adapt as the conversation evolves. Researchers can pursue unexpected insights, digging deeper into interesting responses, which can lead to valuable findings. But, this open-endedness can be a double-edged sword; without a guiding structure, there's a risk of diverging too far from the main focus, potentially resulting in a disorganized data set.
Focus Group Discussions
Focus group discussions bring together a small group of people to discuss a particular topic, led by a moderator. This interaction sparks dialogue among participants, often generating insights that would remain unaddressed in individual interviews. It’s an effective way to gauge public opinion or explore collective attitudes about a subject.
The essence of focus groups lies in the group dynamics. Participants can bounce ideas off one another, leading to deeper insights and diverse perspectives. This collective exploration can uncover themes that individuals may not mention when interviewed one-on-one, making focus groups a valuable asset when examining social contexts.
However, the success of focus groups largely depends on the participants' comfort level and familiarity with each other. If some individuals dominate the conversation, it may drown out quieter members, resulting in skewed data. Moreover, managing group dynamics requires skill from the moderator, which adds another layer of complexity to the process.
Observation Techniques
Observation techniques are a powerful means of collecting qualitative data. They allow researchers to witness firsthand how subjects behave in their natural settings, offering invaluable context that structured inquiries may miss.
Participant Observation
Participant observation involves the researcher immersing themselves within the community or group being studied. This method encourages deep engagement, allowing observers to capture behaviors and contexts in real-time. An ethnographer studying parenting styles, for example, could attend family gatherings to see interactions unfold naturally.
The uniqueness of participant observation lies in its comprehensive perspective. Engaging with subjects adds depth to the findings, as researchers experience scenarios from an insider’s view. However, this intimacy can also introduce bias, as the researcher's presence might alter behaviors, challenging the authenticity of data.
Non-Participant Observation
Non-participant observation, in contrast, allows researchers to observe without directly engaging in the setting. This method ensures that interactions remain as natural as possible, minimizing the impact of the researcher’s presence. It’s most effective in contexts like classrooms or public events, where it’s essential to catch authentic dynamics without interference.
The strength here lies in the unobtrusiveness. Researchers can document behaviors accurately, preserving the integrity of the observed actions. However, the downside is that without interaction, there's limited opportunity to clarify motivations or meanings behind certain behaviors, which might leave data feeling somewhat surface-level.
Content Analysis
Content analysis is another significant method in qualitative data collection. This approach involves systematically evaluating communication artifacts, such as interviews, articles, or social media posts, to extract meaningful patterns and themes. By categorizing and interpreting these materials, researchers gain insights into the cultural and social contexts that shape public discourse.
Unlike methods that involve direct interaction with participants, content analysis relies on existing data, which can be its own advantage. This method allows for examining a larger corpus over time, making it easier to identify trends or shifts in perspectives. Collectively, these methods offered under qualitative data collection can deeply enrich our understanding of human experience and social complexity.
Quantitative Data Collection Methods
Quantitative data collection methods stand as pillars in the realm of scientific research, providing researchers with the means to collect, analyze, and interpret numerical data. This approach focuses on quantifying hypotheses and measuring outcomes, laying down a strong foundation for statistical analysis. It's all about capturing the numbers that tell a story, allowing for objective examination of trends and relationships. Without a doubt, these methods bring rigor and clarity to research, unlocking insights that qualitative methods alone might miss.
Surveys and Questionnaires
Designing Effective Surveys
Designing effective surveys is an essential component of quantitative data collection. The whole idea revolves around crafting questions that accurately capture the information needed. A key characteristic lies in the clarity of language; questions must be straightforward, avoiding jargon that could confuse respondents. This is particularly crucial as the quality of data hinges entirely on respondents understanding what is being asked. Well-designed surveys increase the likelihood of gathering reliable and valid data, which is vital for any research undertaking.
One unique feature of effective surveys is the balance between closed-ended and open-ended questions. While closed-ended questions are quick and easy to analyze, open-ended questions provide deeper insights and context. However, they can also lead to ambiguity and make data analysis more complex. Thus, the challenge of designing effective surveys lies in striking the right balance to suit the research objectives.
Sampling Techniques
Sampling techniques play a crucial role in quantitative data collection, directly influencing the reliability of the results. Selecting a representative sample ensures that the insights gained can be generalized to the broader population. A prominent characteristic of good sampling techniques is randomness; a random sample minimizes bias and enhances the validity of the findings. This makes it a popular choice for researchers aiming for robust conclusions.
In practice, there are unique features of sampling techniques like stratified sampling, where the population is divided into subgroups. This method ensures that specific groups are adequately represented, which can be advantageous to uncover insights that might be overlooked otherwise. On the downside, determining the strata requires additional planning and can be resource-intensive.
Experiments and Trials
Field Experiments
Field experiments are an interesting aspect in the tapestry of quantitative data collection. Here, researchers manipulate variables in a natural setting to observe the effects on the subjects involved. The beauty of field experiments lies in their real-world application; they go beyond the confines of the lab, capturing data in the context where it matters most.
One of the key characteristics of field experiments is their ecological validity, meaning the results can often be generalized to everyday situations. However, they also come with their own challenges, such as difficulty in controlling extraneous variables that might skew the results. But, this trade-off can often lead to richer data that displays true behavior.
Laboratory Experiments
Laboratory experiments, on the other hand, are characterized by control. Conducted in a controlled environment, they allow researchers to manipulate one or more variables while keeping others constant. This enables them to establish cause-and-effect relationships with greater confidence. The method is particularly beneficial when precision is paramount, making it a popular choice among researchers in fields like psychology and biology.
The unique feature of laboratory experiments is the reproducibility of results; the controlled nature allows experiments to be repeated under identical conditions. However, the downside is that results may not always translate well to real-world scenarios. The artificial environment can sometimes lead to behaviors that are not typical of the subjects outside the lab.
Secondary Data Analysis
Using Existing Databases
Using existing databases could be a game-changer for researchers seeking to save time and resources. This approach involves leveraging previously collected datasets for new analysis, making secondary data analysis a powerful avenue in quantitative research. One key characteristic of this method is its efficiency—researchers can quickly access large volumes of data without the time investment of primary data collection.
One unique feature is the variety of available databases from government institutions, universities, and research organizations. The broad spectrum of information can lead to innovative analyses, revealing trends and patterns that might have gone unnoticed otherwise. However, challenges arise in ensuring the quality and relevance of the datasets used, as outdated or poorly collected data can skew new findings.
Data Mining Techniques
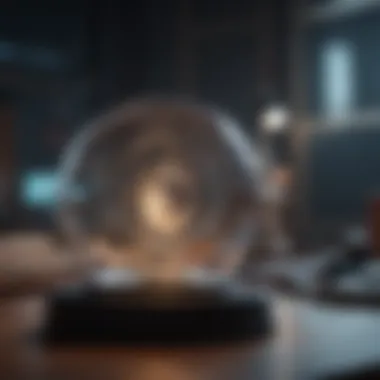
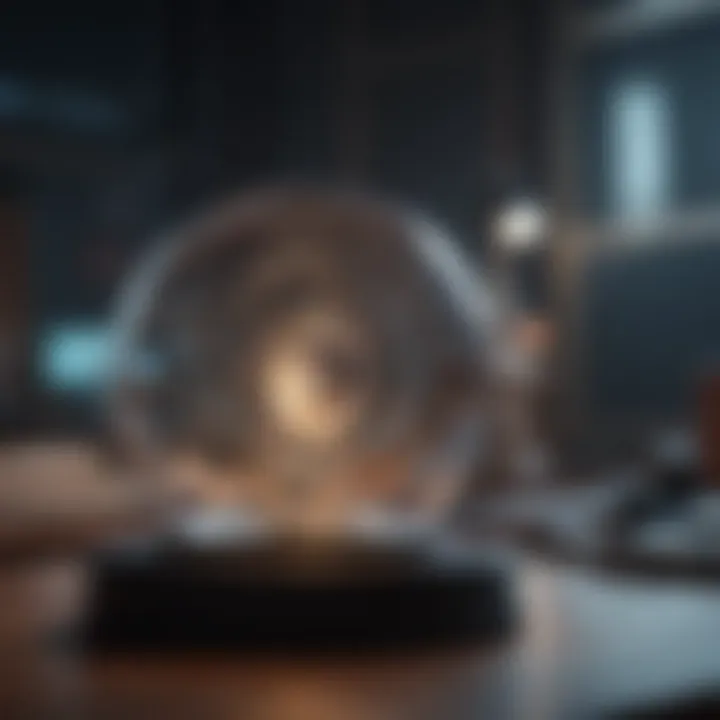
Data mining techniques stand at the crossroads of technology and quantitative research. This process involves analyzing large datasets to extract patterns or insights, driven by algorithms and computational power. A hallmark of data mining is its ability to uncover hidden relationships within the data, often leading to surprising discoveries.
The unique advantage of data mining lies in its scalability; vast datasets that were previously cumbersome to analyze can now be explored rapidly. However, a potential disadvantage includes the risk of misinterpreting correlations as causations, which can lead to faulty conclusions. This necessitates a careful approach and a strong understanding of statistical principles when interpreting findings.
Mixed Methods Approach
The mixed methods approach has earned a reputation as a powerful strategy for data collection, bridging the gap between qualitative and quantitative research. Rather than adhering strictly to one methodology, this approach allows researchers to draw on the strengths of both worlds, facilitating a more comprehensive understanding of complex phenomena. With its ability to harness the richness of qualitative insights alongside the rigor of quantitative analysis, mixed methods stand out in addressing research questions that demand depth and breadth.
Integrating Qualitative and Quantitative Methods
Sequential Explanatory Design
The sequential explanatory design relies on a two-phase approach—first collecting quantitative data followed by qualitative data. This strategy is significant because it lays the groundwork with measurable data and then delves deeper into the findings to explore underlying reasons and explanations. The key characteristic that makes this design particularly appealing is its structure, which systematically guides researchers through their analysis. Researchers can identify patterns or trends from initial data collection and subsequently enrich their findings with qualitative data, providing richer insights.
One unique aspect of the sequential explanatory design is its capacity to refine and clarify the initial quantitative results through participant feedback or further qualitative exploration. For instance, a study on student learning outcomes might start with a survey measuring performance scores and then follow with interviews to understand students' interpretations of their experiences. This combination of data is a beneficial choice for researchers looking for comprehensive insights as it validates and contextualizes numerical data. However, it can be time-consuming and may require a careful balance to ensure that both methods complement each other.
Concurrent Triangulation Design
Concurrent triangulation design is another pillar of the mixed methods framework where both qualitative and quantitative data are collected simultaneously but analyzed independently. This approach aims to corroborate findings across different methods, building a stronger case for research conclusions. A key characteristic of this design is its capacity for triangulation, where outcomes from various data sources reinforce each other, minimizing potential biases and offering a fuller picture.
The unique advantage here is that simultaneous data collection can save time, allowing researchers to address complex questions swiftly. For example, in a health study examining patient satisfaction, while surveys collect numerical ratings, focus groups can provide rich verbal contexts and explanations. This dual approach not only reinforces findings but also enriches the data interpretation process. On the flip side, challenges may arise concerning data integration and potential contradictions between the different sets of data, requiring researchers to engage in careful synthesis.
Benefits of Mixed Methods Research
Mixed methods research offers numerous benefits, making it an appealing choice for scholars across disciplines. Here are a few notable advantages:
- Comprehensive Insights: This approach enables deeper understanding by integrating diverse data sources.
- Flexibility: Researchers can adapt methodologies to fit their research question needs, allowing for tailored responses.
- Validity Boost: Triangulation of data lends credibility to findings by enabling cross-verification.
Ethical Considerations in Data Collection
In the realm of scientific research, ethical considerations play a pivotal role in shaping how data is collected, analyzed, and utilized. The integrity of scientific work is not simply a matter of gathering data; it extends into the principles of responsibility, transparency, and respect for individuals involved in the research process. The conversation around ethics has become increasingly crucial as methodologies evolve and as researchers face new realities brought about by advances in technology and data analysis techniques.
Ethics in data collection can be viewed as an umbrella term encompassing various essential elements:
- Respect for Participants: A fundamental ethical principle in conducting research is to ensure respect for individuals involved. Researchers must consider how their work impacts participants, whether in terms of time, emotional strain, or privacy.
- Integrity of Research: Ethical practices directly influence the credibility of results. Failing to adhere to accepted ethical guidelines can lead to dubious data, ultimately undermining the findings.
- Public Trust: An ethical approach to data collection fosters trust among the public and within the scientific community. Maintaining high ethical standards ensures that research findings are viewed as reliable and applicable.
The benefits of embedding ethical considerations into the core of data collection processes are manifold, enhancing the results' overall quality and applicability.
Informed Consent
Informed consent is the backbone of ethical data collection. This process involves clearly communicating the purpose, risks, and benefits of research to potential participants. They should fully grasp what they are participating in before agreeing. A well-structured informed consent process not only protects the rights of individuals but also builds a foundation of trust between researchers and participants.
Here are a few key points related to informed consent:
- Participants must voluntarily agree without coercion.
- Information provided should be comprehensive, covering all relevant aspects of the study.
- Consent should be documented, usually through a signed form, ensuring transparency.
Practically speaking, informed consent can' be a mere checkbox in the research process; it must be treated with the seriousness it deserves. It’s crucial that researchers revisit this conversation throughout the study, allowing participants the right to withdraw at any point.
Privacy and Confidentiality
Privacy and confidentiality are critical facets of ethical data collection that aim to protect personal information about participants. Researchers are responsible for ensuring that any data collected does not expose sensitive details that could harm participants if disclosed.
The following considerations are vital for maintaining privacy and confidentiality:
- Data Anonymization: Researchers can anonymize data to prevent identification of participants. This often involves removing names and any identifiable information that could link back to individuals.
- Limited Access: Only authorized personnel should have access to sensitive data, ensuring that confidentiality is upheld throughout the research process.
- Secure Storage: Employing secure data storage measures, such as encrypted files and robust data management systems, is key in protecting data integrity against breaches.
"In research, it’s not just about what is done, but how it’s done. Ethical considerations are the framework that guide responsible inquiry, ensuring the safety and rights of participants are at the forefront."
The nuanced balance between data collection and ethical considerations is crucial, not only for the integrity of the research but also for nurturing a respectful engagement with all participants involved. As data collection methodologies continue to transform with technological advancements, the commitment to ethical practices will remain paramount in upholding the integrity of scientific research.
Challenges in Data Collection
In the realm of scientific inquiry, data collection stands as a cornerstone. Yet, this process is often fraught with difficulties that can undermine the very integrity of the research. Recognizing challenges in data collection is not merely an academic exercise; it’s an imperative that allows researchers to be astute in addressing potential pitfalls.
The intricacies of these challenges extend beyond mere technicalities; they encompass philosophical considerations around the nature of knowledge itself. Awareness of these issues can guide researchers in honing their methodologies, ensuring that the conclusions they draw are not just significant but also robustly founded.
Sampling Bias
Sampling bias is a hurdle that can seriously skew results. It occurs when certain members of the intended population are less likely to be included in the sample than others, leading to an unrepresentative picture of reality.
For instance, imagine a researcher studying the effects of a new drug who solely surveys individuals attending a particular health forum online. This would likely exclude those who are not tech-savvy or who have differing health beliefs, thereby not representing the wider population. Such biases can manifest in various ways:
- Selection Bias: Choosing individuals based on convenience rather than randomness.
- Survivorship Bias: Focusing only on cases that have survived or succeeded, ignoring those that haven't.
- Nonresponse Bias: When a significant number of respondents fail to participate, altering the data landscape.
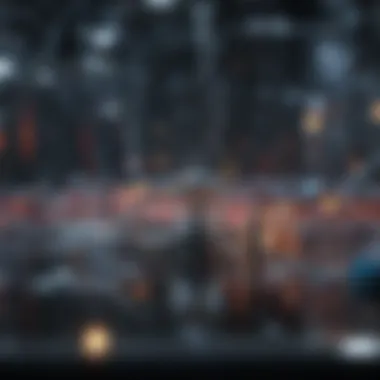
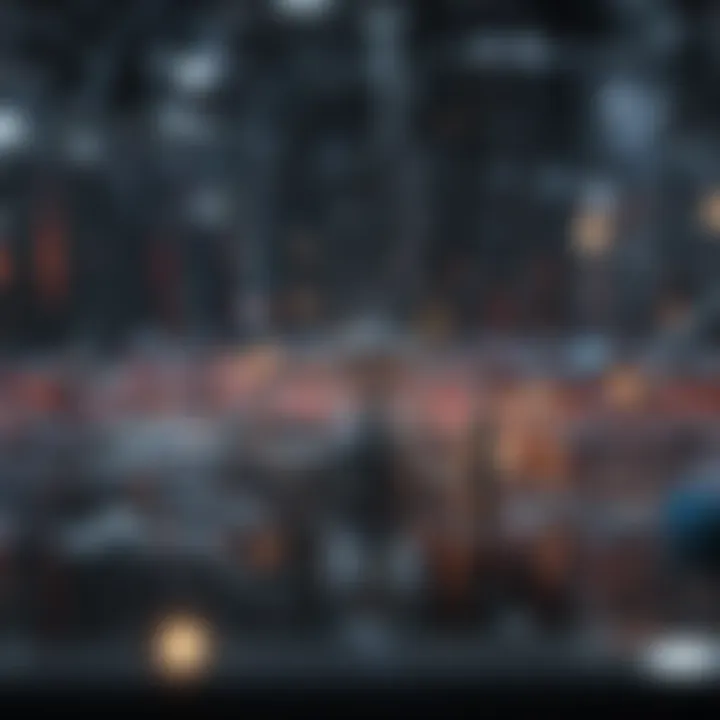
Addressing sampling bias could involve increasing outreach efforts, diversifying recruitment strategies, and employing stratified sampling techniques to ensure all demographic segments are equitably represented. By doing so, the richness of data becomes far more valid and meaningful.
Data Reliability and Validity
Validity and reliability form the backbone of effective data collection methods. Reliability refers to the consistency of a measure, while validity asks whether the tool accurately measures what it claims to measure.
To illustrate, consider a scale used to weigh individuals. If this scale consistently shows discrepancies each time a person steps on it, you have a reliability issue. On the flip side, if it measures weight accurately but does not capture body composition, it lacks validity in assessing health.
Ensuring high data reliability and validity can be accomplished through several methods:
- Standardized Procedures: Developing strict protocols can minimize variations that lead to unreliable outcomes.
- Pilot Testing: Conducting pilot studies allows researchers to refine their instruments before deploying them at full scale.
- Triangulation: Using multiple methods to evaluate the same phenomenon contributes to a more comprehensive understanding and enhances validity.
Addressing these aspects is crucial. If data is reliable, but not valid, decisions made on its basis can lead down a misguided path.
"The foundation of quality research builds itself upon the dual pillars of reliability and validity. One without the other is like a ship without a rudder."
Ultimately, the challenges of data collection are consistent reminders of the complexities inherent in research. For students, educators, or seasoned researchers, overcoming these barriers not only enhances scientific rigor but also contributes to a more nuanced understanding of the world.
Technological Advances in Data Collection
The landscape of scientific data collection has been reshaped tremendously by advancements in technology. As research pushes the boundaries of knowledge, utilizing cutting-edge tools and methodologies not only enhances the efficiency of data gathering but also improves the quality and scope of the information gathered. In an era where speed and accuracy are paramount, understanding these technological changes becomes essential for researchers and professionals alike.
In this section, we delve into two primary facets of these advancements: digital data collection tools and remote sensing and data acquisition. Each of these components plays a pivotal role in modern scientific investigations, allowing researchers to ask complex questions and obtain nuanced answers from diverse datasets.
Digital Data Collection Tools
Digital data collection tools have revolutionized how researchers gather information. These tools range from surveys administered via mobile applications to sophisticated software used for data analysis. Their integration into research processes not only streamlines the collection process but also aids in real-time data analysis, allowing quicker insights.
Some noteworthy tools include:
- Google Forms: A user-friendly platform for surveys, enabling real-time collaboration and data compilation.
- SurveyMonkey: Offers a robust set of options for creating, distributing, and analyzing surveys tailored to various research needs.
- Qualtrics: A more advanced tool that caters to comprehensive survey design and data analysis, often used in academic and market research.
The benefits of utilizing these digital tools are manifold:
- Efficiency: Data collection becomes faster, reducing time delays that can compromise research quality.
- Accessibility: Digital platforms allow for broader reach, gathering responses from diverse populations across geographies.
- Data Integrity: Automated processes reduce human error, ensuring that data collection is more reliable and valid.
However, there are also considerations to keep in mind. Notably, researchers must ensure the tools meet ethical standards concerning privacy and informed consent. This means being transparent about how data will be collected, stored, and utilized.
Remote Sensing and Data Acquisition
Remote sensing represents another remarkable advancement in data collection technologies. This method involves gathering data from a distance, often using satellite or aerial technologies, and it has become indispensable in fields like environmental science, urban planning, and emergency management. By analyzing satellite imagery and other remote data, researchers can gain insights into land use patterns, climate changes, and even disaster response strategies.
Key components include:
- Satellite Imagery: Provides large-scale views of earth conditions, useful for monitoring changes over time, such as deforestation or glacier melting.
- Drones: Equip researchers with high-resolution imaging capabilities, allowing detailed studies of specific locations without being intrusive.
- Geographic Information Systems (GIS): A crucial tool for analyzing spatial data, providing a platform to visualize and interpret various datasets efficiently.
The advantages of remote sensing are striking:
- Wide Coverage: Enables researchers to analyze large areas without the constraints of physical presence.
- Temporal Data: Can offer time-series data, revealing trends and changes that are pivotal for making informed decisions in research and policy.
- Cost-effectiveness: Often less expensive than traditional data collection methods that require extensive manpower and resources.
"The future belongs to those who prepare for it today." – Malcolm X
Understanding and integrating these technological advances can provide significant leverage, ensuring that one's research is not only relevant but also impactful in addressing contemporary challenges.
Closure
When we discuss the key elements of effective data collection, it becomes evident that understanding both qualitative and quantitative methods is essential. Each path has its own advantages and limitations that can significantly influence the research results. By conducting mixed methods research, professionals have the opportunity to synergize the strengths of both approaches, ultimately painting a fuller picture of the phenomena under investigation.
Moreover, the evolving nature of technology necessitates that researchers remain abreast of advancements in digital data collection tools and innovative methodologies such as remote sensing. This not only enhances the quality of the data but also broadens the scope of what is researchable within diverse disciplines.
As we summarize the key insights drawn throughout this article, it's evident that:
- Diverse approaches to data collection enrich the research landscape, providing robust methodologies for various scenarios.
- The implications of effective data collection methods extend beyond number crunching; they play a significant role in ensuring the ethical standards and reliability of research.
- Awareness and integration of new technologies propel the scientific community further into uncharted territories, revealing answers to questions we haven’t yet thought to ask.
Summarizing Key Insights
As we reflect on the most salient points, we see an overarching theme: the choice of data collection method is not merely a procedural step; it's a fundamental aspect of research design.
When we assess methods such as interviews, surveys, experiments, and observational techniques, it becomes clear that:
- Qualitative methods such as interviews bring rich, narrative-driven data that can uncover nuanced human experiences.
- Quantitative approaches like surveys provide numeric data that can be analyzed statistically for broader patterns and correlations.
- Mixed methods encourage a more comprehensive data analysis, capturing both richness of context and overall trends.
Through this multifaceted lens of examination, we can appreciate the essence of selecting appropriate methods, which directly correlates to research outcomes and their applicability in the real world.
Future Directions in Data Collection Methodologies
Looking ahead, the landscape of data collection will continue to evolve, particularly as technology advances. Future directions in this field may include:
- The incorporation of AI and machine learning to enhance predictive analytics in data collection, thereby facilitating more informed decisions.
- Greater emphasis on mobile technologies to capture real-time data in diverse settings.
- A push toward standardization of data collection practices across disciplines, which can enhance comparability and replication of research studies.
- Ethical considerations will remain a pressing issue, calling for a deeper understanding of data privacy, especially with the rise of big data.
In summary, acknowledging and adapting to these changes will serve to enrich the scientific community, ensuring that researchers can effectively tackle the contemporary challenges they face. The dialogue about data collection methodologies will not only continue but deepen, making it an exciting area for students, researchers, and professionals to engage with.