Exploring Synthetic Health Data in Modern Healthcare
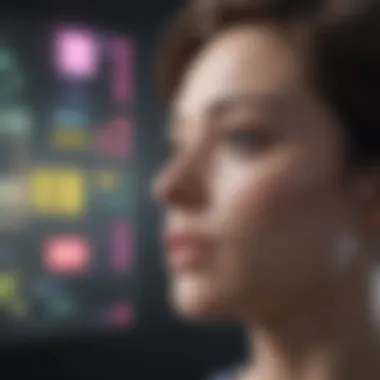
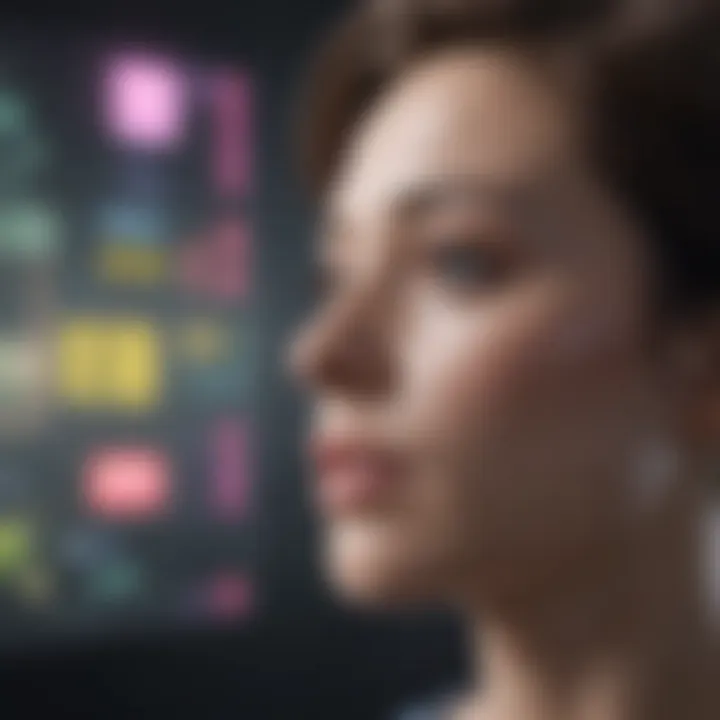
Intro
The landscape of healthcare is transforming rapidly, driven by advancements in technology and the growing importance of data. Among these innovations is the emerging field of synthetic health data. By utilizing sophisticated algorithms, this data type is generated from existing patient information while ensuring privacy. This not only raises questions of efficacy but also emphasizes the ethical considerations surrounding patient data handling.
In this article, we will take a closer look at synthetic health data, its applications, and its potential impact on healthcare today and in the future. We aim to offer insights for students, educators, researchers, and professionals who are keen to understand the nuances of this vital development in modern healthcare.
Prologue to Synthetic Health Data
The emergence of synthetic health data represents a significant shift in the way healthcare research can be conducted and implemented. As the healthcare landscape evolves, the need for more robust, secure, and ethically sound data practices has become apparent. Synthetic health data, which simulates real patient information while protecting individual privacy, has the potential to transform research methodologies and enhance patient care by providing a wealth of insights without compromising sensitive information.
This section serves as a foundation to understand the implications of synthetic health data in modern healthcare. It highlights not only the definition and historical context of the topic but also its importance within the context of ongoing developments in medical technology and research. By exploring these elements, we can appreciate how synthetic health data facilitates advancements in various healthcare domains, thus ensuring more effective treatments and interventions.
Definition and Overview
Synthetic health data refers to artificially generated datasets that emulate real patient data but do not contain any identifiable information. This data is created using algorithms that analyze actual patient records and generate new, yet statistically similar, health information. The primary advantage of synthetic data lies in its ability to maintain the statistical properties of the original data while eliminating the risk associated with data breaches or unauthorized disclosures.
The use of synthetic health data can minimize barriers for researchers who require access to large datasets for their studies, while simultaneously upholding stringent privacy standards. This ensures that innovations in healthcare can continue to progress without infringing on patientsβ rights.
Historical Context
The concept of synthetic data is not new, but its application in healthcare has gained momentum in recent years due to growing concerns about data privacy. In the early days of health data management, sensitive patient data was often used in research without adequate consideration for privacy. As technology developed, so did the recognition of the need for secure data handling practices.
The 21st century brought landmark legislation focused on data protection, such as the Health Insurance Portability and Accountability Act (HIPAA) in the United States. These regulations heightened awareness and reshaped how patient data was accessed and utilized in research. The transition from using real patient data to synthetic health data became a logical response to these challenges. Researchers and technologists began to explore ways to generate synthetic datasets that could still offer valuable insights into health trends, potential trials, and varying treatments without compromising patient confidentiality.
As a result, the landscape of healthcare research has transformed; synthetic health data now stands as a key component in advancing knowledge while addressing ethical concerns prevalent in previous years. Ultimately, understanding the definition and historical evolution of synthetic health data is crucial for grasping its role in modern healthcare practices.
The Process of Generating Synthetic Health Data
The generation of synthetic health data is a fundamental process that enables researchers and practitioners to utilize realistic datasets without compromising individual privacy. As the healthcare industry increasingly relies on data-driven insights, understanding the mechanisms involved in creating synthetic data is crucial. It allows for innovation while addressing stringent ethical considerations that arise from working with real patient information. Here, we explore key elements of this process, the advantages it offers, and some considerations that need to be kept in mind.
Data Source Identification
Data source identification serves as the foundational step in generating synthetic health data. This process involves pinpointing reliable datasets that can inform the creation of substitute information. Sources typically include electronic health records (EHR), public health databases, and clinical trial datasets.
Choosing the right data is not trivial. The sources should have sufficiently representative data to ensure that the synthetic data mirrors real-world distributions and patterns. It is essential for the generated data to maintain the statistical properties of the original datasets while ensuring individual identities are obscured.
Some strategies for effective data source identification include:
- Comprehensive analysis of existing datasets to understand their breadth.
- Ensuring compliance with data protection regulations before utilizing any real patient data.
- Collaboration with healthcare institutions to gain access to anonymized data, enhancing the integrity of the synthetic dataset.
Algorithmic Frameworks
Once data sources are identified, the next step is employing algorithmic frameworks to generate synthetic health data. Various algorithms can be used, depending on the desired characteristics of the synthetic dataset. Commonly used frameworks include Generative Adversarial Networks (GANs) and probabilistic models.
These algorithms work by learning the underlying distributions of the target data. For example, GANs consist of two competing networks, one generating synthetic data and the other evaluating its authenticity. This process continues until the generated data is sufficiently indistinguishable from real data.
Key considerations when selecting an algorithm include:
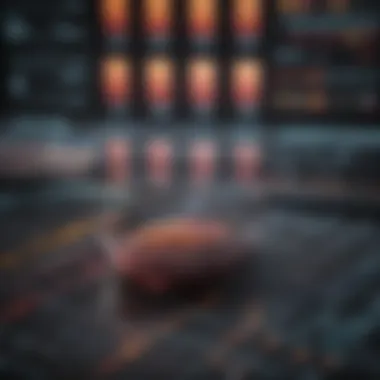
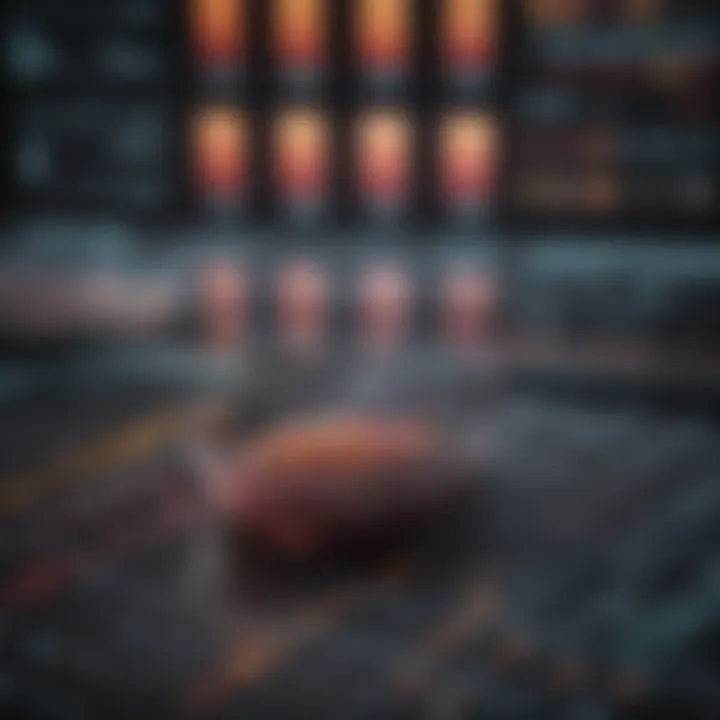
- The complexity of the healthcare data being modeled.
- The computational resources available, as some algorithms require significant processing power.
- The intended application of the synthetic data, which can dictate the specific requirements of the algorithm.
Validation Techniques
Validation techniques are critical in ensuring that the synthetic health data generated is fit for purpose. This involves assessing how well the synthetic data corresponds to the original dataset in terms of statistical properties and predictive capabilities.
Common validation techniques include:
- Statistical tests to compare distributions and variances between original and synthetic datasets.
- Model performance metrics when using synthetic data for predictive modeling tasks, evaluating metrics like accuracy and recall.
- Domain expert reviews, integrating insights from healthcare professionals to validate the plausibility of the generated data.
Applications in Healthcare Research
The application of synthetic health data in the field of healthcare research is a significant highlight of this modern approach. This area continuously evolves, driven by the need for data solutions that address privacy concerns while enabling robust research methodologies. Synthetic health data provides a unique opportunity to utilize comprehensive datasets created from real patient data without exposing individual identities. This is particularly crucial for a society increasingly aware of data privacy issues.
Clinical Trials
Synthetic health data plays a vital role in clinical trials, which are crucial for testing new treatments and interventions. Traditional clinical trials often face time constraints and limited participant pools. With the introduction of synthetic data, researchers can simulate diverse patient populations and predict various outcomes without compromising patient confidentiality. By mimicking real-world scenarios, synthetic data facilitates the evaluation of treatment efficacy across different demographics and health conditions. This optimization not only shortens the trial phases but also enhances the overall quality of the evidence generated.
"Synthetic health data can revolutionize the way we approach clinical trials by providing scalable options that respect patient privacy."
Epidemiological Studies
Epidemiological studies seek to understand the patterns, causes, and effects of health and disease conditions in defined populations. Utilizing synthetic health data can significantly enhance these studies by allowing researchers to model disease outbreaks or public health interventions without relying solely on sensitive real-world datasets. This flexibility provides a broader understanding of disease transmission and risk factors. Additionally, researchers can explore hypothetical scenarios, which can lead to valuable insights about population health dynamics and potential intervention strategies.
Personalized Medicine
The shift towards personalized medicine revolutionizes how healthcare is delivered. Synthetic health data supports this trend by enabling researchers to analyze diverse genetic and phenotypic data from large populations. This analysis can identify specific patient profiles that may respond favorably to tailored treatment options. By leveraging synthetic data, clinicians can simulate varied treatment regimens and predict individual responses, thus enhancing the customization of patient care. This capacity for personalization is pivotal in achieving more effective and efficient health outcomes across populations.
Ethical Considerations
The integration of synthetic health data into modern healthcare brings crucial ethical considerations to the forefront. Navigating these considerations is vital for maximizing the benefits of this innovative approach while minimizing potential risks. In this section, we delve into three specific elements: patient privacy and data security, informed consent, and bias and fairness. Each plays a significant role in shaping a responsible framework for the use of synthetic health data.
Patient Privacy and Data Security
Synthetic health data is often seen as a solution to patient privacy issues. By generating data that mimics real patient records without revealing personal identifiers, it aims to alleviate concerns related to data breaches. However, the protection of sensitive information remains paramount. Ensuring data security involves robust encryption methods and strict access controls. Institutions must adopt regulations such as the Health Insurance Portability and Accountability Act (HIPAA) to safeguard patient privacy in the digital health landscape.
In practice, organizations must be diligent. Proper training for personnel handling synthetic data on security protocols can further fortify defenses. Audit trails and regular assessments of data access can detect unauthorized usage. Thus, while synthetic data offers benefits, security must remain a top priority, ensuring that the integrity of patient information is not compromised.
Informed Consent
Informed consent is a foundational aspect of medical ethics. In the context of synthetic health data, the dialogue shifts. Traditional consent processes may not apply since the data is not directly linked to identifiable individuals. Thus, institutions must find ways to educate patients about how their data contributes to research while ensuring transparency.
This can take the form of clear communication strategies. Patients should understand how synthetic data is generated, what it entails, and its potential implications. The development of guidelines is necessary to establish standards for obtaining consent when utilizing real patient data to generate synthetic datasets. Engaging patients through informational sessions or written materials can enhance understanding and reinforce trust in the research process.
Bias and Fairness
Bias in synthetic health data can lead to significant implications in healthcare research. If the algorithms used to generate synthetic data are trained on biased datasets, the resulting information will perpetuate those biases. This can distort research findings, particularly in studies related to personalized medicine or clinical trials.
Detection and correction of bias must be prioritized throughout the data generation process. This involves careful analysis of training datasets to ensure diversity is represented. Incorporating fairness principles into algorithm development is essential for creating equitable healthcare outcomes. Engaging diverse stakeholders during the design phase can enhance the objectivity and reliability of synthetic health datasets.
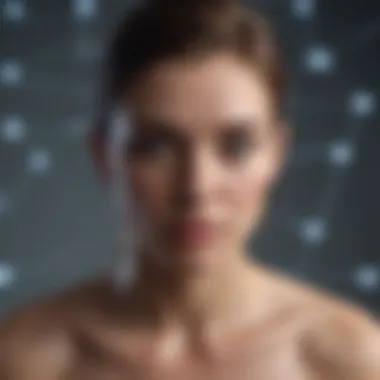
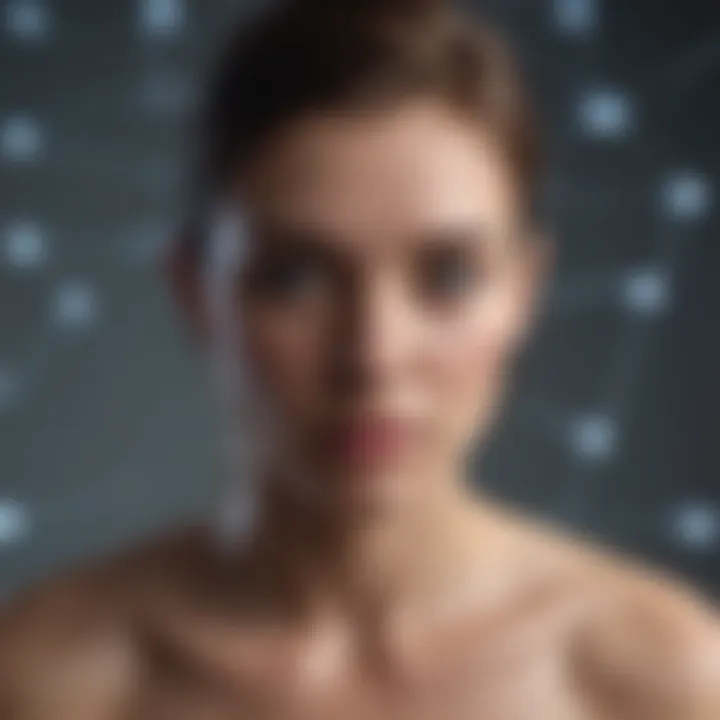
"Ethical lapses in data handling can undermine years of research and damage public trust in healthcare systems."
Recognizing and addressing bias also furthers the establishment of standards for fairness in synthetic health data usage. Clear metrics to evaluate equity in outcomes should be the goal of researchers and healthcare providers.
Challenges and Limitations
The utilization of synthetic health data introduces numerous challenges and limitations that warrant careful consideration. Understanding these factors is essential for maximizing the effectiveness of such data in healthcare applications. As synthetic datasets become more prominent in research and decision-making, addressing these challenges can define the future trajectory of healthcare innovation.
Data Quality and Reliability
Ensuring high-quality synthetic health data is pivotal. If the underlying algorithms produce data that lack accuracy, the conclusions drawn from this data may end up misleading. Data quality includes criteria such as completeness, consistency, and accuracy.
Organizations must prioritize thorough validation processes to enhance reliability. This involves comparing synthetic datasets against real-world datasets to ensure representativeness. Techniques such as statistical validity checks can expose potential discrepancies. Without rigorous validation, the utility of synthetic data can be severely compromised.
Technical Limitations
The technology behind generating synthetic health data is sophisticated, but it is not without limitations. Algorithmic approaches can be constrained by computational capabilities and the complexity of the data structures involved. Often, the intricate dependencies found in real-world health data are hard to replicate precisely in synthetic models.
Moreover, techniques like machine learning and deep learning may struggle when trained on synthetic data that lacks diversity. A lack of varied examples can limit the effectiveness of AI applications, making them less capable of generalizing. Continuous improvements in algorithms and techniques are necessary, but the speed of advancements can vary significantly across different fields of healthcare.
Regulatory Hurdles
Navigating regulatory environments poses another significant challenge. Synthetic health data must satisfy various legislative frameworks which aim to protect patient information. Regulations like the Health Insurance Portability and Accountability Act (HIPAA) in the United States introduce constraints on data usage and sharing practices.
Adherence to regulations is paramount. Organizations involved in synthetic data generation must remain agile to adapt to evolving legal standards. Ensuring compliance while fostering innovation requires balancing act, as excessive regulations could stifle the beneficial uses of synthetic health data.
Ultimately, confronting these challenges is crucial for harnessing the benefits of synthetic health data fully. Only through careful management of quality, technical constraints, and legal obligations can the healthcare sector unlock its true potential.
The Role of Synthetic Health Data in Artificial Intelligence
The intersection of synthetic health data and artificial intelligence (AI) is a burgeoning area that holds promise for modern healthcare. Synthetic health data serves as a critical foundation for training AI models and enhancing predictive analytics. The benefits of utilizing synthetic data are evident, but it also throws up several considerations that must be meticulously navigated.
One of the foremost advantages of synthetic health data in AI is that it allows researchers and developers to circumvent the ethical and logistical challenges associated with using real patient data. In an era where data breaches are prevalent, generating synthetic data mitigates privacy risks, enabling the exploration and analysis of health trends. As synthetic datasets resemble biological realism without containing any identifiable information, they become perfect candidates for building robust AI solutions.
Moreover, the ability to access vast, diverse datasets enhances the robustness of AI algorithms. This enables machine learning models to uncover insights that may not be visible within limited real-world datasets. In this way, synthetic health data not only propels innovation but also addresses some of the constraints posed by traditional data collection methodologies.
Training AI Models
The training of AI models relies heavily on the availability of vast amounts of high-quality data. Synthetic health data creates a rich reservoir from which data scientists can draw. By using synthetic datasets, developers can train algorithms to identify patterns and make predictions more effectively. For instance, when sufficient data is available, models can learn to detect diseases earlier, evaluate treatment efficacy, and tailor healthcare interventions.
Another important feature of using synthetic data in training is the flexibility to generate specific scenarios or conditions. For example, researchers can create datasets that include rare diseases or unique patient demographics that are often underrepresented in traditional health data. This capability to customize datasets leads to the development of AI models that are better suited to addressing real-world complexities.
Despite these clear advantages, challenges remain. The model's effectiveness hinges on the quality of the synthetic data produced. If the data lacks the necessary variability or does not accurately reflect the complexities of real patient data, the efficacy of the AI model might be compromised. Thus, maintaining a delicate balance between realism and ethical considerations is essential.
Predictive Analytics
Predictive analytics is another area where synthetic health data proves invaluable. Healthcare institutions are increasingly employing predictive modeling to forecast health outcomes based on prior information. By integrating synthetic health data into these models, healthcare providers can make informed decisions and implement preventative strategies.
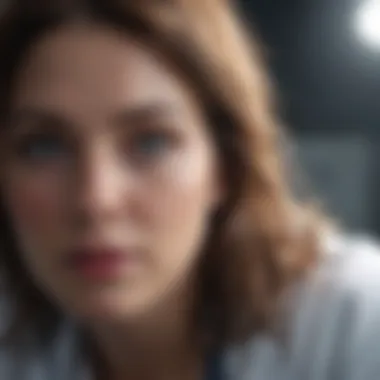
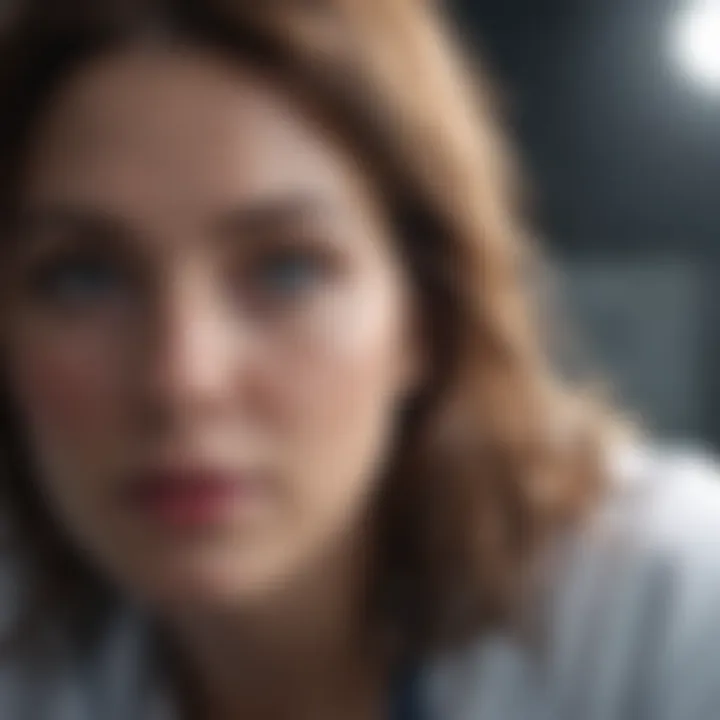
One critical application is in the management of chronic diseases. Synthetic data allows for the simulation of patient journeys and potential outcomes based on various factors such as lifestyle, medication adherence, and socio-economic status. This enhances the predictive power of the analytics, providing a more comprehensive view of how different interventions might impact patient health.
Furthermore, predictive analytics powered by synthetic health data can be instrumental in resource allocation. Hospitals can better forecast demand for services based on the patterns established in synthetic datasets. This not only streamlines operations but also ensures that patient care is optimized in a resource-constrained environment.
However, like training AI models, predictive analytics fueled by synthetic health data is not without risks. If predictions are based on flawed synthetic data or if the algorithms themselves are not rigorously tested, it could lead to poor decision-making, ultimately affecting patient care.
Future Directions
The landscape of synthetic health data continues to evolve rapidly. Understanding the future directions in this field is vital for maximizing its benefits while addressing inherent challenges. Future advancements are critical as they will ultimately determine how effectively synthetic health data can be utilized in modern healthcare. Emphasizing technological advancement and seamless integration with existing healthcare systems are two significant areas poised for exploration.
Advancements in Technology
Technology is the backbone of synthetic health data generation. Advances in artificial intelligence and machine learning will enable even more sophisticated models capable of producing highly accurate synthetic datasets. As algorithms become more refined, the generated data will increasingly reflect real-world complexities. For instance, better natural language processing capabilities may improve the representational quality of clinical narratives, enriching the synthetic datasets.
Moreover, integration of blockchain technology could ensure data integrity and traceability throughout the synthetic data lifecycle. The use of decentralized systems will provide an additional layer of security, ensuring that patient information remains confidential while retaining high-quality data characteristics.
Key areas of focus could include:
- Enhanced Algorithm Development: Continuous refinement of the algorithms used for data generation to ensure they mimic real-world patient data more effectively.
- Data Enrichment Techniques: Integrating new data sources, such as genomics, may provide deeper insights and create richer datasets.
- Interdisciplinary Collaborations: Engaging with various fields such as computer science, ethics, and healthcare will streamline technological advancements.
Integration with Existing Systems
For synthetic health data to truly revolutionize healthcare, it must seamlessly integrate with current electronic health records (EHR) and other healthcare systems. This integration should not just be a technical requirement but a fundamental strategic approach.
A primary consideration is ensuring that synthetic data is compatible with existing databases. Efforts should include developing specific APIs that allow easy manipulation and interaction with real-time data systems. Furthermore, fostering partnerships between synthetic data developers and healthcare organizations could streamline the adoption of these technologies more effectively. The potential benefits of successful integration are substantial:
- Improved Analytical Capabilities: Integrated synthetic data can enhance predictive analytics across various domains, from patient outcomes to resource allocation.
- Facilitated Research and Development: When synthetic data merges with actual patient data, it creates ample opportunities for innovative clinical research, substantially benefiting biotechnology and pharmaceutical companies.
- Real-time Decision Making: Combining real-time data with synthetic datasets will provide clinicians and healthcare professionals with tools that support informed decisions immediately.
"The synthesis of technology and healthcare is not merely a trend; it's a fundamental shift toward optimizing patient care and operational efficiency."
Culmination
The exploration of synthetic health data is vital in contemporary healthcare discussions. This article highlights several key elements surrounding this topic, emphasizing the benefits and considerations involved in utilizing synthetic datasets.
One of the primary insights is the capacity of synthetic health data to enhance research capabilities while ensuring patient privacy is maintained. With advancements in technology, researchers can generate realistic yet non-identifiable datasets that enable thorough analysis. This charged environment opens doors to various applications, from clinical trials to personalized medicine, each carrying significant implications for medical research and practice.
"Synthetic health data has the potential to revolutionize how we approach healthcare research, providing insights without compromising individual privacy."
However, ethical considerations remain a pressing concern. Issues such as bias in data generation and the need for informed consent require ongoing scrutiny. Addressing these ethical dilemmas is paramount for wide acceptance among researchers and practitioners alike. Engaging discussions and further research into these aspects will only heighten the efficacy of synthetic health data's integration into healthcare practices.
Summary of Insights
In summary, synthetic health data offers an innovative approach to resolving current challenges in healthcare data management. It allows:
- Enhanced patient privacy protections
- Improved data access for research
- Greater availability of diverse datasets
Despite its potential, the use of synthetic health data must be approached with caution. Discussions about bias, fairness, and regulatory compliance must take center stage to ensure that the benefits are fully realized without compromising ethical standards. Future advancements would ideally focus on refining algorithms and validation techniques that mitigate inherent risks.
Call to Action for Researchers
To capitalize on the benefits of synthetic health data, researchers are encouraged to:
- Engage in interdisciplinary collaborations: Working with technologists, ethicists, and legal experts can lead to more robust frameworks for data usage.
- Invest in education and training: Researchers should seek to understand the intricacies of synthetic data generation and its ethical ramifications fully.
- Publish findings and best practices: Sharing insights with the wider community can foster a culture of knowledge and innovation that harnesses the full potential of synthetic health data.
As the field evolves, researchers must remain vigilant and proactive, ensuring that advancements in synthetic health data reshape healthcare positively and ethically.