Exploring Survey Levels: Insights for Researchers
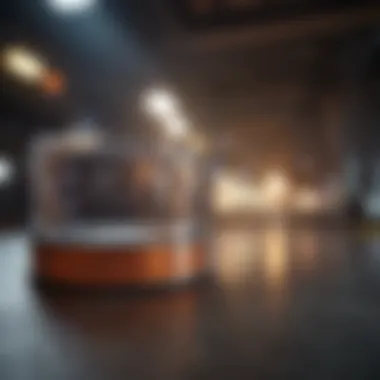
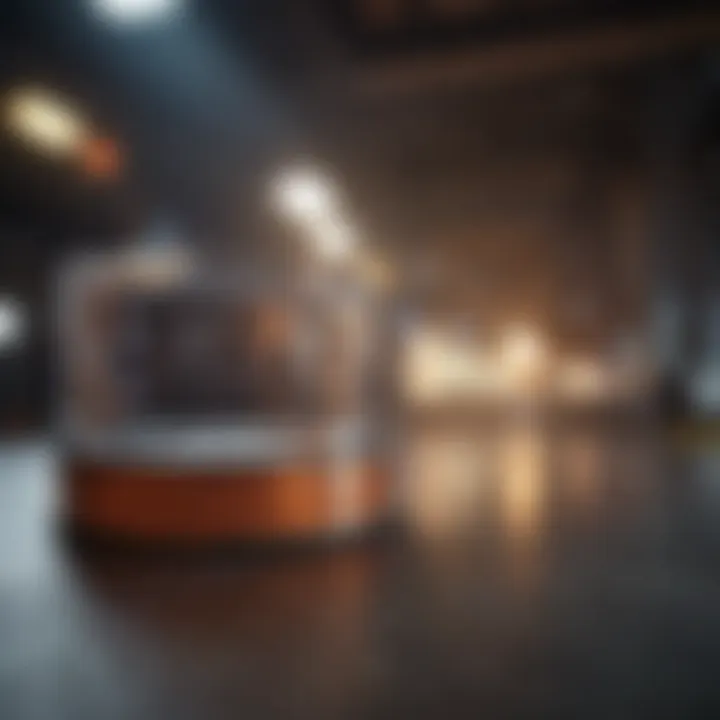
Intro
The concept of survey levels is foundational in understanding how data is collected and analyzed in various fields, particularly within scientific research. By framing survey methodologies around these levels, researchers can gather insights more precisely and with greater relevance. This section will provide a solid footing for exploring the multifaceted nature of survey levels, helping readers grasp their significance in data collection and research practices.
Understanding Survey Levels
Survey levels refer to the different stages or classifications in survey design, each characterized by unique attributes and objectives. These levels offer a structured approach to gathering information, making it easier for researchers to achieve clear and valid results. Understanding these distinctions can help students and professionals alike enhance their ability to conduct effective surveys, ultimately leading to more reliable data.
Importance of Survey Design
A well-structured survey design can significantly influence the quality of insights derived from data collection. Poorly constructed surveys may lead to biased or incomplete data, skewing results and impacting the reliability of conclusions. Therefore, understanding the implications of survey levels is crucial in striving for accurate and relevant findings.
Target Audience
This article aims to serve students, researchers, educators, and professionals who seek a deeper understanding of survey methodologies. By delving into the linguistic and practical elements of survey levels, readers will become better equipped to engage with research protocols.
"Properly understanding survey levels is not just an academic exercise, but a vital skill for extracting meaningful insights from complex data."
This exploration begins with the significance of defining various survey levels and categorizing them properly to enhance the effectiveness of research efforts. As we proceed, we shall unpack the intricacies involved in different survey classifications, methodologies, and their respective applications in real-world scenarios.
Foreword to Survey Levels
Understanding survey levels is essential to the field of research as it frames the way data is collected and interpreted. Each level of measurement offers different insights, influencing the types of analyses researchers employ. Recognizing these levels enables better communication of findings and a more profound appreciation of the nuances within the data.
Survey levels categorize data based on attributes and represent a hierarchy ranging from nominal to ratio. This classification system impacts methodologies used in both data collection and analysis, affecting outcomes, conclusions, and later applications of the gathered information. Each level provides distinct characteristics and allows for varied statistical techniques, which are crucial in shaping research designs and implementation strategies.
Understanding Survey Levels
Survey levels generally encompass four classifications: nominal, ordinal, interval, and ratio.
- Nominal level refers to basic categorization, where data is grouped without any quantitative value.
- Ordinal level addresses categories with a known order but not equal distances between them.
- Interval level allows for meaningful differences but lacks a true zero point.
- Ratio level has meaningful differences and a true zero, leading to the most comprehensive analysis capabilities.
Each level has its own set of rules for data interpretation, making it critical to select the correct level during survey design.
Importance of Survey Levels in Research
Survey levels are pivotal in research for several reasons.
- Precision in Data Collection: Choosing the correct level streamlines the collection process and enhances the data's accuracy.
- Influence on Statistical Analysis: The selected level of measurement dictates which statistical methods can be applied, influencing the validity of the results.
- Interpretability of Findings: Understanding the level helps in accurately interpreting findings and conveying research outcomes.
- Guiding Future Research: These classifications can inform subsequent studies, helping researchers build upon existing knowledge effectively.
Thus, survey levels serve as a backbone in research methodology, directly impacting the quality and clarity of data analysis.
Types of Survey Levels
Understanding the types of survey levels is fundamental for effective data collection and analysis. Each level allows researchers to gather, quantify, and interpret data in different ways. The survey level selected will influence every aspect of the research, from the design of the questions to the methods used for analysis. Thus, making an informed choice about which survey level to use can enhance the clarity and relevance of the data obtained.
Nominal Level Surveys
Definition and Characteristics
Nominal level surveys categorize data without any meaningful order. This level is primarily used to label variables for easy identification. For example, survey questions may ask respondents about their favorite color, where answers like "red," "blue," or "green" don't have any intrinsic hierarchy. The key characteristic of nominal data is its inability to indicate how much more one category possesses than another. This makes it a popular choice in qualitative research, where such distinctions help in identifying trends and patterns among various groups.
Examples in Research
Nominal level surveys are frequently employed across diverse fields. One common example is in demographic studies, where researchers may categorize respondents by gender or ethnicity. Such classifications allow for effective analysis of differences between groups. The unique feature of nominal surveys is that they facilitate straightforward statistical analysis, typically using frequency counts. However, their limitation lies in the inability to derive any measure of central tendency, such as an average.
Ordinal Level Surveys
Definition and Characteristics
Ordinal level surveys offer a rank-ordering of variables, presenting a clearer picture than nominal levels. For instance, a question that asks respondents to rate their satisfaction on a scale from "very unsatisfied" to "very satisfied" uses ordinal data. Here, the key characteristic is the rank ordering of responses. This method is beneficial because it provides insights into preferences or attitudes that can be analyzed comparatively. Yet, it is essential to note that while ordinal levels concisely represent order, they do not quantify the distance between ranks.
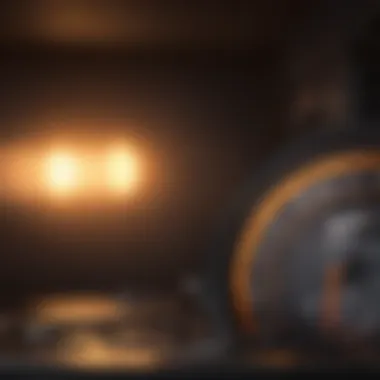
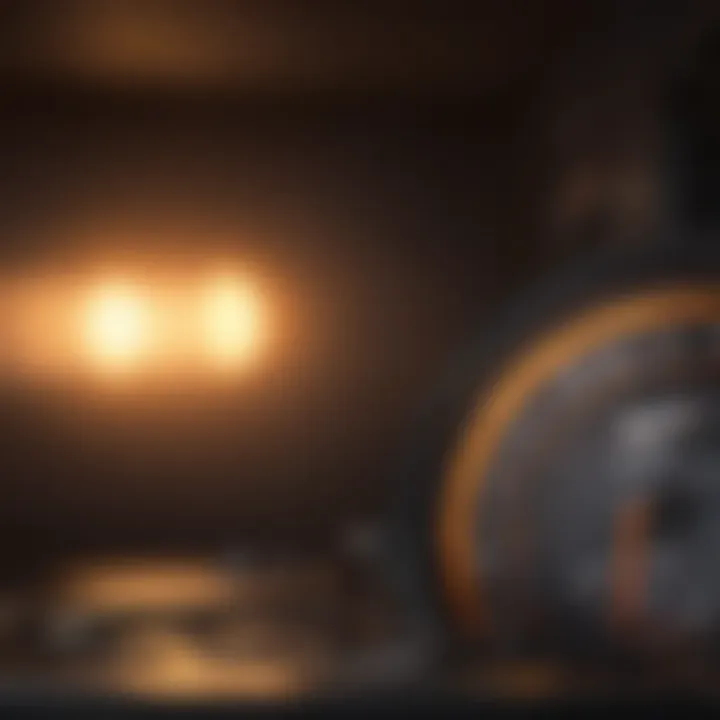
Examples in Research
Ordinal level surveys can be seen in customer satisfaction research. Businesses often use scales to gauge how satisfied their customers are with products or services. Such data can be very useful in making informed decisions about service improvements. The unique advantage of ordinal surveys is their simplicity in gathering preferences. However, they may suffer if exact differences between ranks are assumed, which can lead to misinterpretation of data.
Interval Level Surveys
Definition and Characteristics
Interval level surveys represent data with equal intervals between points, allowing for a meaningful calculation of differences. An example can be temperature measured in Celsius. The fundamental characteristic here is the defined distance between the values, which empowers researchers to conduct statistical operations. This level is particularly useful for scientific data analysis, permitting thorough interpretations. However, interval data lacks a true zero point, rendering relative comparisons somewhat limited.
Examples in Research
Interval surveys are often utilized in studies related to psychology where researchers may use scales like the Likert scale, which equates to equidistant points. This feature empowers comprehensive analysis of attitudes or perceptions. Despite its advantages in providing detailed insights, the lack of a true zero can complicate some aspects of statistical analysis.
Ratio Level Surveys
Definition and Characteristics
Ratio level surveys are the most comprehensive, incorporating all characteristics from previous levels while possessing a true zero. This enables a full range of statistical analyses, making it a highly versatile choice for many research scenarios. For instance, weight or height data collected through surveys exhibits a clear zero point, establishing a numerical basis for comparison and analysis. The key characteristic is its ability to support a wide array of mathematical operations, enhancing the depth of insights obtainable from the data.
Examples in Research
In fields like economics or health research, ratio level data often appears in studies measuring income or body mass index. The unique feature is the complete set of possible mathematical operations it permits, offering valuable insights for researchers. The primary challenge, however, lies in collecting ratio data accurately, as this often requires specific measurement tools or techniques.
Choosing the Right Survey Level
Selecting the appropriate survey level is crucial for effective data collection and analysis. The decision influences how information is interpreted and ultimately shapes research outcomes. Choosing the right survey level ensures that the research questions align with the data analysis techniques and ultimately leads to meaningful insights. This section discusses specific elements that impact this choice, as well as the benefits and considerations to keep in mind.
Factors Influencing the Choice of Survey Level
Several factors play a role in determining the suitable level for a survey. These include:
- Research Objectives: Understanding what you intend to achieve with your research is vital. Clear goals dictate the types of data needed and subsequently, the survey levels that are appropriate.
- Nature of Variables: The characteristics of the variables being measured can dictate the survey level. For instance, nominal variables require nominal levels, while age can be measured at the ratio level.
- Target Population: The demographics of your respondents also matter. Certain populations might prefer or better understand specific types of questions.
- Analytical Techniques: The selected analytical methods must correspond with the survey level. Different statistical techniques apply to different data types; for example, chi-square tests are suitable for nominal data.
- Context of the Study: The setting in which data will be collected may influence the choice. For example, academic settings might allow for more complex surveys compared to community-based settings where simplicity may be crucial.
By carefully considering these factors, researchers can make informed decisions that enhance the reliability and validity of their findings.
Consequences of Survey Level Selection
The selection of a survey level carries significant consequences that can affect both the research process and the interpretation of results. Some key consequences include:
- Data Quality: Choosing an inappropriate level may lead to issues such as insensitivity in data capturing or misrepresentation of respondents’ opinions. For instance, using ordinal measures for what should be nominal data can obscure meaningful differences.
- Analysis Complexity: If a survey level is misapplied, the analysis may become overly complicated or yield incorrect results, ultimately hindering decision-making.
- Generalizability: The choice can affect how broadly findings can be applied to other populations. For instance, nominal level surveys might be simpler but may not capture the nuances found in interval or ratio level surveys.
- Resource Implications: Developing appropriate surveys can require significant time and financial resources. Incorrect selection can lead to wasted efforts in designing surveys that ultimately do not meet the research objectives.
It’s vital for researchers to engage in thorough planning and consultation when deciding on survey levels, as these choices have far-reaching implications for the integrity of their research.
By ensuring that the right survey level is chosen, researchers can enhance their methodology, improve data quality, and ultimately provide more accurate and actionable insights.
Designing Surveys Based on Levels
Designing a survey is not just about asking questions; it is about understanding how to frame those questions based on the survey level. Each survey level—nominal, ordinal, interval, and ratio—demands specific considerations in the way questions are structured. This section will outline the importance of designing surveys according to these levels and how it can significantly impact data quality and research outcomes.
Constructing Survey Questions for Different Levels
When constructing survey questions, the level of measurement directly influences the type of questions that can be asked. At the nominal level, questions should be categorical. For instance, asking respondents to select their gender or favorite color is suitable here. The responses will categorize data without any inherent sequence.
In contrast, ordinal level surveys involve questions that reflect ranked data. An example could be asking respondents to rate a service on a scale from poor to excellent. This setup allows for comparison based on order, but not on the magnitude of difference.
At the interval level, questions like temperature in degrees Celsius or satisfaction ratings from 1 to 10 offer precise differences between the values. Every interval is consistent, enabling more sophisticated analysis.
Lastly, ratio level questions must include absolute zero. An example of this might be questions involving age or income, where zero indicates a total absence of the quantity measured. Choosing the right question type for the appropriate survey level will enhance clarity and validity of the data collected.
Pilot Testing and Validation
Pilot testing is an essential step in the survey design process. It allows researchers to look for potential issues in question clarity and respondent understanding before carrying out the main survey. During this phase, a small group of individuals will take the survey as if it were the final product. This stage is crucial because it can uncover misinterpretations, ambiguous questions, or technical issues that could distort data.
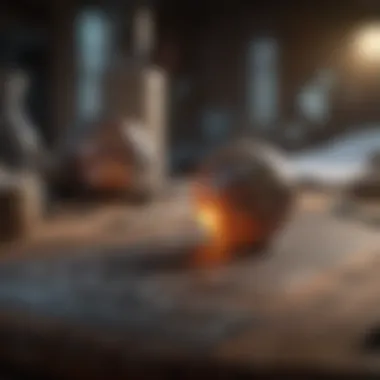
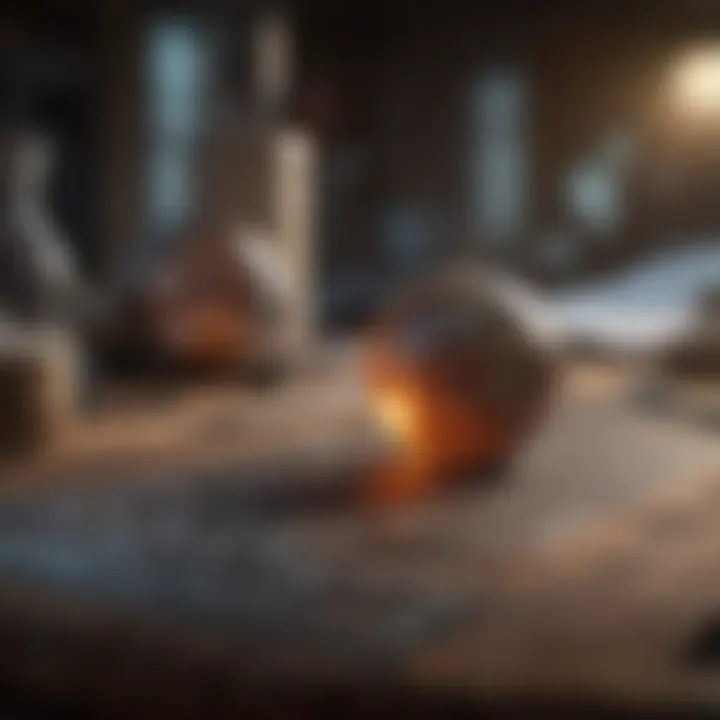
Validation measures how well the survey tool measures what it intends to measure. It ensures that the results are credible and can influence effective decision-making. For example, if a survey designed to assess customer satisfaction does not accurately capture the dimensions of satisfaction, the results will mislead interpretations and policy adjustments.
In summary, careful construction of survey questions and thorough pilot testing and validation are pivotal for effective research. They ensure that the survey levels are accurately represented and that the data collected can be reliably analyzed.
Data Analysis Techniques by Survey Level
In the realm of survey research, effective data analysis techniques are integral to extracting valid insights from collected information. Understanding the appropriate statistical methods to apply at each survey level ensures accurate interpretations and facilitates better decision-making. This section focuses on data analysis techniques categorized by survey levels. By appreciating these methods, researchers can choose the right tools to analyze their data correctly, leading to robust findings.
Statistical Methods for Nominal Data
Nominal data refers to categories without any intrinsic ordering. Common statistical methods for analyzing nominal data include:
- Chi-Square Test: This test checks if there is a significant association between two categorical variables. It helps to identify relationships in survey responses, useful in market research and demographic studies.
- Frequency Distribution: This method organizes and summarizes nominal data, which provides a clear picture of how responses are distributed across categories. It can display counts or percentages.
When working with nominal data, researchers should ensure that the data is accurately categorized to avoid erroneous conclusions.
"Statistical methods for nominal data allow researchers to discern patterns in categories without implying any order."
Statistical Methods for Ordinal Data
Ordinal data reflects ranking but does not specify the magnitude of difference between ranks. Appropriate methods for analyzing ordinal data include:
- Mann-Whitney U Test: This non-parametric test compares two independent groups' differences, helping determine if one group tends to have higher ranks than the other.
- Kendall's Tau: This statistic measures the strength and direction of association between two ranked variables. Utilizing it aids in understanding the relationships among ranked responses.
- Ordinal Logistic Regression: Ideal for predicting an ordinal dependent variable, this regression analysis models the relationship between ordinal data and independent variables.
By selecting methods tailored to ordinal data, researchers can quantitatively evaluate perceptions or preferences.
Statistical Methods for Interval Data
Interval data contains ordered values with meaningful differences but lacks a true zero point. For this type of data, several statistical methods can be employed:
- T-Tests: These tests compare the means of two groups to see if they are significantly different from each other. They can be valuable in evaluating responses on interval scales.
- ANOVA (Analysis of Variance): ANOVA checks for differences among three or more groups simultaneously. This method helps discern variances across multiple surveys or conditions.
- Correlation and Regression Analysis: Assessing relationships and predicting outcomes using interval variables can provide deeper insights. These tools help understand how one variable might predict another.
Interval data analysis can yield rich insights when methodologies align with the dataset's characteristics.
Statistical Methods for Ratio Data
Ratio data possesses all the qualities of interval data, with the addition of a meaningful zero point. Common methods for analyzing ratio data include:
- Descriptive Statistics: Measures like mean, median, and mode can describe ratio data's central tendency and dispersion. These methods offer a comprehensive summary of the dataset's key characteristics.
- Regression Analysis: This analysis can help to establish relationships between ratio variables, offering the ability to predict outcomes based on continuous data.
- Multivariate Analysis: Techniques such as multiple regression or factor analysis allow researchers to examine multiple variables simultaneously, making it possible to uncover complex relationships.
Incorporating appropriate statistical methods tailored to ratio data can comprehensively evaluate relationships in complex datasets, leading to informed conclusions.
Challenges in Survey Levels
Understanding challenges in survey levels is crucial for ensuring the effectiveness of research. Survey levels determine how data is collected, analyzed, and interpreted. Mistakes in this area can yield misleading results, ultimately affecting decision-making. Challenges can arise from various corners: from design flaws to the misreading of data outcomes. Each type of survey level presents unique challenges that can impede the research objectives if not recognized and managed appropriately.
Common Pitfalls in Survey Design
When designing surveys, researchers often fall into certain pitfalls. One primary issue is ambiguity in questions. Questions that are unclear may lead to inconsistent responses, which hinders the quality of the data. Additionally, leading questions may influence respondents' answers, thereby skewing the results.
Another significant challenge is failure to pre-test the survey. Without a pilot test, flaws in the survey might go unnoticed until it is too late, leading to unreliable data collection. This highlights the importance of a thorough review prior to the actual survey deployment. Moreover, not considering the target audience when formulating questions can lead to misunderstandings and, ultimately, incomplete or erroneous datasets.
Other common pitfalls include:
- Overloading with too many questions: This can cause fatigue among respondents.
- Neglecting response options: Missing options or limited alternatives can force respondents to select answers that do not represent their true views.
- Ignoring data privacy: Not addressing concerns about confidentiality can deter participation.
Misinterpretation of Results
Interpreting survey data incorrectly is another significant challenge. Misinterpretation stems from various factors, including lack of statistical knowledge, biases, and a poor understanding of survey levels. For instance, using the wrong statistical methods for different survey levels can lead to incorrect conclusions.
A misstep can turn meaningful results into confusion. Consider the difference between nominal and ordinal data. Assuming that ordinal data can be treated like nominal data can skew analyses, leading to conclusions that do not accurately reflect reality.
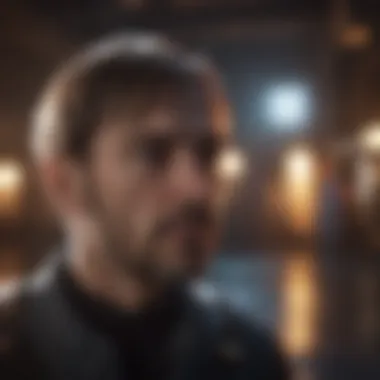
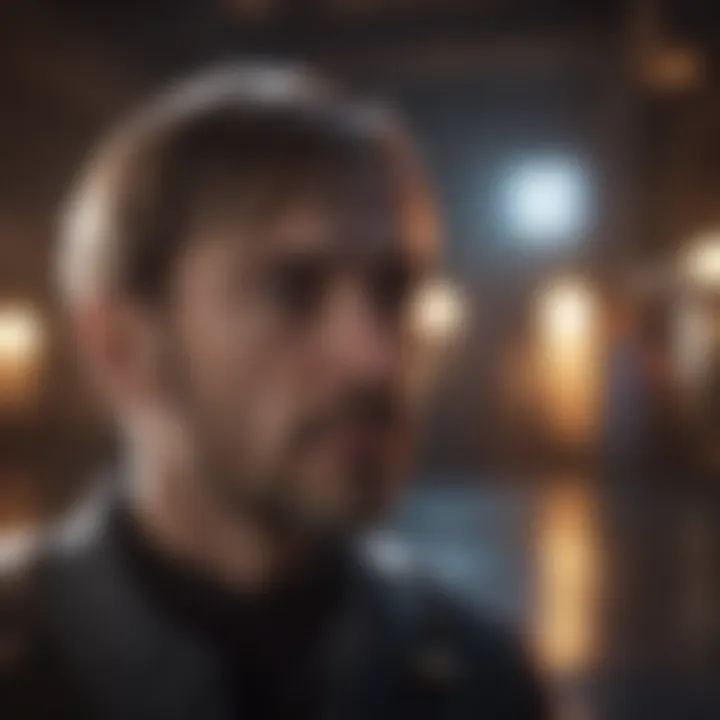
Moreover, confirmation bias may play a role where researchers only interpret results that align with their expectations. This selective interpretation can further distort findings and mislead stakeholders.
To minimize misinterpretations, researchers should:
- Employ appropriate statistical methods according to the survey level.
- Seek peer reviews to validate interpretations.
- Diversify interpretation perspectives to reduce bias.
"The integrity of survey research lies in proper survey design and accurate interpretation of data. Ignoring these aspects can lead to significant errors in research outcomes."
Addressing these challenges in survey levels is not just about refining the survey process. It is about enhancing the overall value of research output, ensuring more reliable and actionable insights emerge from well-conceived and executed surveys.
Case Studies in Survey Levels
Case studies in survey levels provide crucial insights into practical applications, demonstrating how theoretical concepts translate into real-world settings. They serve as exemplars of effective survey design and highlight the effectiveness of various survey levels in addressing specific research questions. In this context, analyzing case studies fosters a deeper understanding of the impacts that survey levels can have on data collection and the validity of findings. Such analyses reveal tangible benefits that arise when researchers thoughtfully select the appropriate survey levels.
Application in Social Science Research
In social science research, survey levels are instrumental for categorizing responses and analyzing social behaviors. Researchers often utilize nominal and ordinal levels to assess public opinion, social trends, and demographic characteristics. For example, a study might categorize respondents based on their political affiliation, using a nominal level to distinguish between parties such as Democratic, Republican, or Independent.
When investigating social phenomena, researchers might also employ ordinal scales to measure attitudes or perceptions. Surveys measuring satisfaction with community services might use a Likert scale (e.g., from "very satisfied" to "very dissatisfied") to capture a spectrum of feelings. By applying these survey levels, social scientists can uncover correlations and draw meaningful conclusions about societal issues.
In one notable case study, a survey was conducted to assess the impact of educational programs on community engagement. The researchers used both nominal and ordinal survey levels to categorize participants' levels of involvement before and after the program. Through data analysis, they identified a significant increase in participation, demonstrating how survey levels can illuminate relationships between interventions and social behavior.
Application in Health Research
In the realm of health research, case studies exemplify how different survey levels facilitate the understanding of health-related behaviors and outcomes. Health researchers leverage interval and ratio levels for rigorous analysis of numeric data, such as blood pressure readings or body mass index measurements. These levels allow for a precise assessment of health metrics and can reveal important trends in patient populations.
For instance, a health study might involve administering a survey that quantifies patient satisfaction with healthcare services. Researchers could use an interval scale to measure satisfaction on a scale from 1 to 10, allowing for nuanced comparisons between diverse patient groups. This data can inform healthcare providers about areas needing improvement.
In one impactful case study, a survey evaluated the effectiveness of a new medication among different demographic groups. Researchers deployed ratio levels to measure dosage and outcomes like cholesterol levels. This systematic approach provided clear insights, illustrating the medication's efficacy across varying factors.
"Case studies in survey levels not only exemplify methodologies but also validate the appropriateness of survey choices in specific research contexts."
Analyzing these case studies equips future researchers with practical examples, encouraging thoughtful selection of survey levels that align with their research goals. By understanding these applications, students and professionals can enhance the reliability of their own study outputs.
Future Directions in Survey Research
The future of survey research is shaped by ongoing developments in methodology and technology. As researchers strive for more accurate and reliable data, understanding these changes is crucial. Emerging trends in survey research reflect evolving attitudes towards data collection and the integration of advanced tools and techniques. Being aware of these trends allows scholars and practitioners to adapt their approaches, ensuring their research is contemporary and relevant.
Emerging Trends in Survey Methodology
Recent years have brought several innovations in survey methodology. These trends focus on enhancing the data collection process and improving the quality of responses.
- Mixed-Methods Approaches: Combining qualitative and quantitative methods offers richer insights. By using interviews alongside traditional surveys, researchers can delve deeper into participants' perspectives.
- Adaptive Survey Designs: Surveys that adapt in real-time to respondent answers can lead to more relevant questioning. This flexibility can improve response rates and data accuracy.
- Engagement Strategies: New methodologies prioritize respondent engagement to reduce fatigue. Techniques include shorter surveys, interactive formats, and incentives to encourage participation.
- Cultural Sensitivity in Surveys: A rising focus on cultural context improves understanding and relevance. Recognizing diverse backgrounds can enhance the validity of survey results.
Understanding these trends is vital for any research aiming to stay relevant. It is essential to be aware of these changes to leverage them effectively in one's own research.
The Role of Technology in Survey Levels
Technology influences every aspect of survey research today. This integration enhances not only data collection but also analysis and feedback processes. Some critical advancements include:
- Online Survey Platforms: Tools like SurveyMonkey and Google Forms allow for quick distribution and real-time results collection. These platforms often feature customizable templates, making it easier to tailor questions to various survey levels.
- Mobile Technology: With smartphones, data collection has become more accessible. Surveys can be conducted via mobile apps, increasing participant reach, especially in younger demographics.
- Machine Learning Algorithms: These algorithms can analyze large datasets to find patterns or predict trends. Their use improves survey analytics, providing deeper insights into respondent behavior.
- Data Security: Enhancements in data protection ensure respondent privacy. Implementing secure data collection methods promotes trust, which is essential for obtaining genuine responses.
"The integration of technology in survey research not only streamlines processes but also expands possibilities for data interpretation."
In summary, the future of survey research holds promise for increased sophistication and precision. By embracing emerging trends and understanding the role of technology, researchers can ensure their methodologies remain robust and impactful.
The End
The conclusion serves as a pivotal section of this article, synthesizing the core concepts discussed regarding survey levels. It encapsulates the main ideas, reinforcing the importance of understanding survey levels in data collection and analysis. Survey levels offer a structured approach that aids researchers and practitioners in selecting the appropriate methodologies for their studies.
Key Takeaways on Survey Levels
In considering the key takeaways, several points emerge that underline the significance of this topic:
- Diverse Types: There are four main types of survey levels: nominal, ordinal, interval, and ratio. Each type has its unique characteristics that influence the type of data collected and the analysis methods used.
- Importance in Research: The correct identification of survey levels affects the quality of research findings. It informs the design, aids in the interpretation of results, and guides the choice of appropriate statistical methods.
- Informed Decision-Making: Researchers who are knowledgeable about survey levels can make informed decisions that enhance the integrity and applicability of their research outcomes.
- Future Implications: As technology evolves, the methodologies surrounding survey levels continue to adapt. Researchers must stay informed about emerging trends and innovative techniques in survey design.
"Understanding survey levels not only facilitates effective data collection but also enhances the legitimacy of research findings, making this knowledge indispensable for researchers in any field."
In summary, recognizing the nuances of different survey levels equips students, researchers, educators, and professionals with the insights necessary for conducting meaningful research. It fosters a deeper understanding of data interpretation and its implications in various fields, ultimately elevating the standard of research quality.