Leading Figures in Artificial Intelligence Research
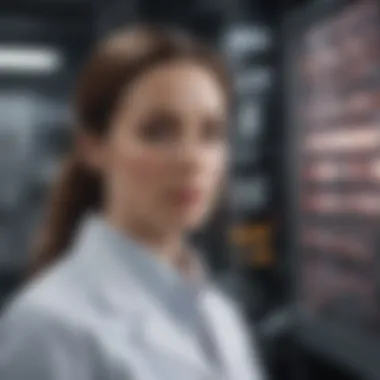
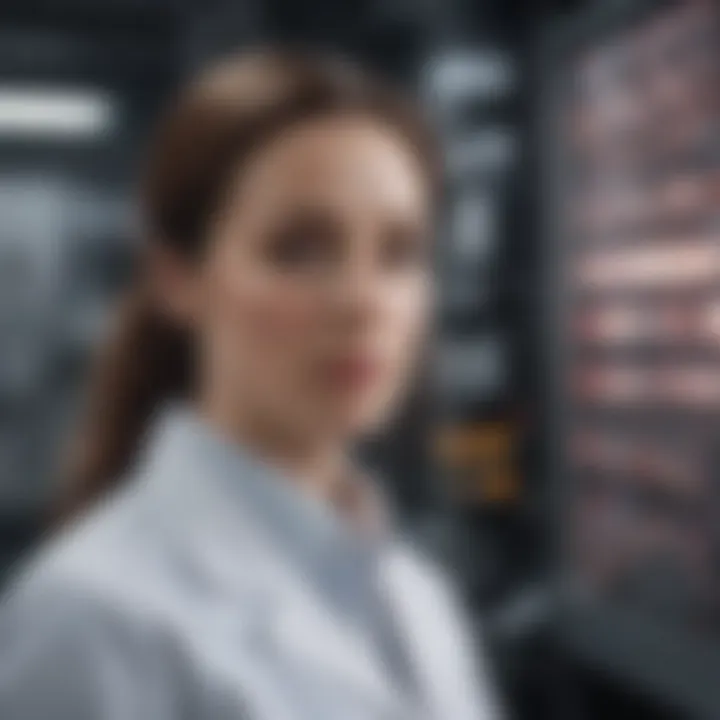
Intro
Artificial intelligence has become a significant area of research, driving innovation and advancement in multiple fields. Understanding the key figures who contribute to this discipline provides insight into the methodologies and ideologies that shape AI today. These researchers not only pave the way for breakthroughs but also influence the ethical considerations surrounding AI applications. Beyond their individual contributions, their collective impact leads to societal shifts, as AI technology advances swiftly. Through this article, we will explore the prominent researchers, the institutions they are associated with, and the trajectory of their research, offering a clear picture of the present and future landscape of artificial intelligence.
Research Highlights
Overview of Key Findings
The contributions made by influential figures in artificial intelligence are profound. Some focus on machine learning, while others delve into natural language processing or robotics. For instance, Fei-Fei Li has made significant strides in computer vision through her leadership in the ImageNet project. Her work helped reshape how machines perceive and interpret visual data. Similarly, Geoffrey Hinton has been pivotal in deep learning, which has vastly improved AI's capabilities in tasks previously seen as insurmountable.
Significance of the Research
The research conducted by these leading figures is not only significant in academic circles but also resonates strongly within industry applications. The findings enable advancements in voice recognition systems, self-driving cars, and medical diagnostics. Moreover, their work prompts discussions about ethical AI usage, particularly concerning privacy and bias in algorithms. These issues require careful consideration, as they affect how society interacts with technology.
"The best way to predict the future is to invent it." - Alan Kay
Original Research Articles
Summary of the Article
Many original research articles from these leading figures showcase their methodologies. They present innovative approaches that have been adopted by other researchers, fostering a collaborative spirit in the AI community. For example, a notable article by Yann LeCun details advancements in convolutional networks, laying the groundwork for facial recognition technologies.
Author Contributions
Each of these researchers contributes unique perspectives and expertise. From Andrew Ng's work on MOOCs promoting AI education to Yann LeCun's pioneering efforts in deep learning, their publications serve as benchmarks in the field. Furthermore, these contributions educate newcomers and inspire ongoing exploration into the potential of artificial intelligence. Understanding their findings is essential for anyone keen on delving into AI research or applications.
Overview of Artificial Intelligence Research
The exploration of artificial intelligence research is vital for understanding how this technology has evolved and shaped our world. This section highlights the key elements and benefits of AI research, acknowledging its wide-ranging implications across various sectors. The rapid advancements in AI technologies have made it essential to grasp both the definitions and the historical contexts that underpin current methodologies. A strong foundation in the overview of AI research enables a deeper appreciation of the intricacies involved in developing intelligent systems.
Definition and Scope
Artificial intelligence encompasses a broad spectrum of techniques aimed at simulating human-like cognitive functions. These functions include learning, reasoning, problem-solving, perception, and language understanding. AI can be categorized into two primary types:
- Narrow AI, which specializes in specific tasks, such as image recognition or voice assistants.
- General AI, which aims to perform any intellectual task that a human can do.
The scope of AI research extends beyond computer science and delves into areas such as neuroscience, cognitive psychology, and data analytics. Researchers prioritize solving complex problems and enhancing automation, with implications that resonate in domains like healthcare, transportation, finance, and education.
Historical Context
The genesis of artificial intelligence can be traced back to the mid-20th century when pioneers began exploring the concept of machines capable of simulating intelligence. Early work by individuals like Alan Turing laid the groundwork for AI research, introducing the Turing Test as a measure of machine intelligence.
In the subsequent decades, significant milestones emerged, including the development of algorithms that enabled machines to play chess and solve mathematical problems. However, the field faced periods of stagnation, referred to as "AI winters," due to unmet expectations and limited technological resources.
The resurgence of AI research in recent years can largely be attributed to improvements in computational power, increased availability of data, and advancements in machine learning techniques. Such developments have shifted the focus toward deep learning and neural networks, propelling AI technologies into practical applications.
Overall, understanding the history of artificial intelligence research provides essential insights into its current landscape and future trajectory.
Factors Influencing AI Research
The landscape of Artificial Intelligence (AI) research is complex and multifaceted. Various elements drive progress in this field, each contributing uniquely to shaping its future. Understanding these factors is essential for contextualizing the contributions of leading figures in AI.
Technological Advancements
Technology serves as the backbone of AI research. The evolution of computational power is one major advancement. Graphics Processing Units (GPUs) have significantly increased speed and efficiency in processing vast datasets. With improved hardware, researchers can explore deeper neural networks, leading to better performance in tasks like image and speech recognition.
Another important technological leap is the emergence of cloud computing. It allows researchers to access resources on-demand, enabling large-scale experiments without substantial investment in local infrastructure. This democratization of technology has opened doors for many researchers, facilitating collaboration and enhancing the overall pace of innovation.
Furthermore, advancements in algorithms play a vital role. Breakthroughs such as reinforcement learning and transformer models have transformed how machines learn from data. These new methods allow AI systems to adapt and make decisions based on context, moving beyond traditional approaches which often rely on static rules.
Interdisciplinary Collaboration
The role of interdisciplinary collaboration in AI research cannot be overemphasized. AI's application spans multiple domains like healthcare, finance, transportation, and more. Collaborating across these fields brings together diverse perspectives and expertise, enhancing research quality and applicability.
For instance, partnerships between technologists and medical professionals have led to remarkable advancements in predictive analytics for patient care. AI algorithms can analyze medical records and predict health outcomes, improving diagnostics and treatment plans.
Additionally, interdisciplinary teams are often more effective at addressing ethical considerations that arise in AI. By involving ethicists and social scientists, researchers can better understand the societal implications of their work and create more responsible technologies.
Ultimately, the synergy between technology and collaboration fosters an environment that promotes innovation and responsible research practices in AI.
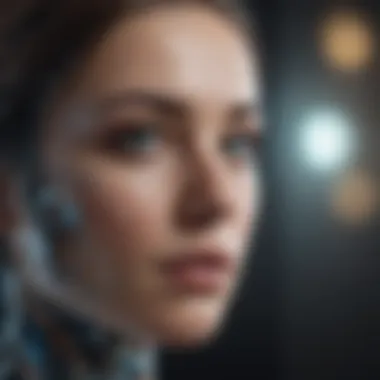
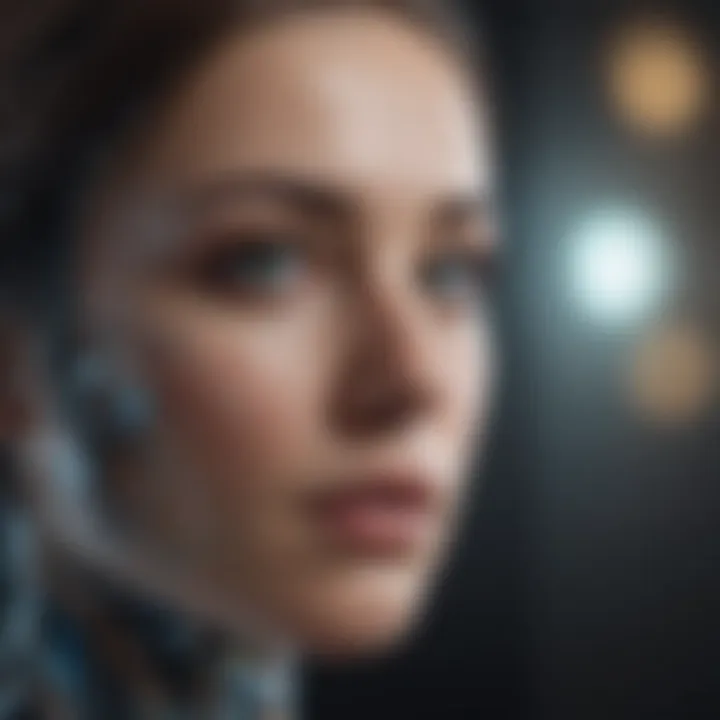
"The future of AI will be shaped by those who understand and merge multiple disciplines."
Through understanding these factors, a clearer picture emerges of the evolving nature of AI research and its leading figures. These elements not only influence the current state of AI but also hint at potential future advancements.
Prominent AI Researchers
Understanding the contributions of prominent AI researchers is essential in grasping the evolution of artificial intelligence. These individuals have not only influenced the trajectory of AI research and development but have also shaped the theoretical frameworks and practical applications that define the field today. Their pioneering work has led to advancements in various AI disciplines, including machine learning, robotics, and natural language processing. Additionally, they play a crucial role in inspiring the next generation of researchers and practitioners. In this section, we will explore two categories of these influential figures: pioneers and modern influencers.
Pioneers in AI
The pioneers of AI laid the groundwork for many of the concepts we now take for granted. Figures like Alan Turing, John McCarthy, and Marvin Minsky contributed foundational ideas that still resonate today. Alan Turing, often called the father of computer science, introduced the concept of the Turing machine. His work on algorithm design and computational theory was revolutionary. John McCarthy is credited with coining the term "artificial intelligence" in 1956 and he also developed the Lisp programming language, which became vital for AI programming.
Marvin Minsky, a co-founder of the MIT AI Lab, was instrumental in exploring neural networks and theories related to perception. These pioneers faced skepticism and low funding in their early years. Yet, they persevered and established AI as a legitimate field of research. Their vision has continually paved the way for future researchers, anchoring ambitious projects that drive AI today.
Modern Influencers
The landscape of AI research has greatly shifted with the emergence of modern influencers. These are researchers who leverage technological advancements and harness interdisciplinary approaches to push the boundaries of AI further. For instance, Geoffrey Hinton has been a prominent figure in deep learning, significantly contributing to the resurgence of neural networks over the past decade. His work on backpropagation is critical for training deep neural networks, resulting in improved performance for tasks such as image recognition and natural language understanding.
Another key modern influencer is Yann LeCun, who has focused on convolutional neural networks. His contributions have been particularly influential in fields like computer vision. LeCun's collaboration between academia and industry showcases how applied research can lead to tangible technological advancement.
In addition, Timnit Gebru stands out for her focus on ethics in AI, emphasizing the societal implications related to biases in algorithms. Her work calls for a more responsible approach to AI development. Together, these modern influencers exemplify how current research not only builds upon foundational theories but also addresses the pressing challenges of today.
"AI is not just about algorithms; it’s about people, ethics, and the very way technology interacts with our lives."
Through their combined efforts, prominent AI researchers continue to illuminate the path forward for AI, ensuring a balance between innovation and responsibility.
Key Contributions to AI
Algorithm Development
Algorithm development serves as the backbone of artificial intelligence. Researchers create new algorithms to enhance the performance of AI systems. Notably, advancements in machine learning algorithms have led to significant improvements in pattern recognition and decision-making capabilities. For instance, the introduction of deep learning by researchers such as Yann LeCun and Geoffrey Hinton has changed how we approach complex datasets.
The contributions are diverse.
- Neural Networks: These algorithms mimic human brain functions, allowing systems to learn from large amounts of data.
- Reinforcement Learning: This approach enables AI to learn optimal actions through trial and error, a method seen in games like chess and Go.
- Generative Adversarial Networks (GANs): Proposed by Ian Goodfellow, GANs are a game-changing innovation in data generation, seen in various applications from art to medicine.
These developments show the potential of AI algorithms in advancing not just technology, but improving productivity across numerous sectors.
Theoretical Foundations
The theoretical foundations of AI are crucial for creating robust systems. Researchers articulate the principles that underpin how AI operates. These principles include theories from computer science, cognitive science, and statistics. Foundations set the stage for innovative methods and improvements.
Key areas of development include:
- Computational Learning Theory: It discusses how algorithms learn from data, influencing the design of more effective systems.
- Probabilistic Reasoning: This aspect allows AI systems to handle uncertainty, forming the basis for decision-making under risk.
- Logic and Knowledge Representation: Understanding logical structures has implications for natural language processing and the development of intelligent agents.
Theoretical advancements ensure that AI progresses in a meaningful and scientifically sound way, illuminating paths for future research and innovation.
Real-World Applications
Real-world applications of AI illustrate how abstract concepts translate to practical solutions. Contributions from leading figures have led to breakthroughs across various fields, demonstrating the societal implications of their work.
Prominent applications include:
- Healthcare: AI aids in diagnostics, personalized medicine, and patient management systems, fundamentally changing how we approach health.
- Finance: Innovations enhance fraud detection, algorithmic trading, and risk management, leading to improved financial security and efficiency.
- Transportation: The development of autonomous vehicles showcases AI's capabilities in navigation and decision-making, presenting new possibilities for urban mobility.
"AI is not just a tool; it is an integral part of human progress."
The applications highlight the far-reaching influence of AI and underscore the importance of ongoing research to address challenges presented by these technologies. Every aspect of AI research contributes to a comprehensive understanding, paving the way for advancements that can address future global challenges.
Challenges Faced by AI Researchers
The pursuit of advancements in artificial intelligence (AI) is often met with a variety of challenges. Navigating these obstacles is crucial for researchers aiming to make significant contributions to the field. Understanding these challenges enhances the discourse on how AI can evolve responsibly and effectively. Ethical considerations and data privacy issues stand out as particularly important factors in this context.
AI research is not just about the technology; it fundamentally intersects with societal values and the implications of machine intelligence on everyday life.
Ethical Considerations
Ethics in AI is a critical area requiring attention. As AI systems become more integrated into various aspects of life, it is essential to address questions around bias, fairness, and accountability. AI has the potential to replicate or even exacerbate societal biases that exist in the data from which algorithms learn. For example, if facial recognition technology is trained on datasets that lack diversity, the resulting AI may perform poorly on underrepresented groups.
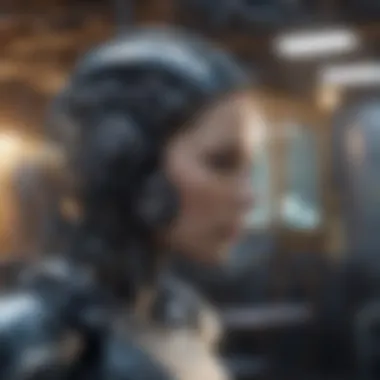
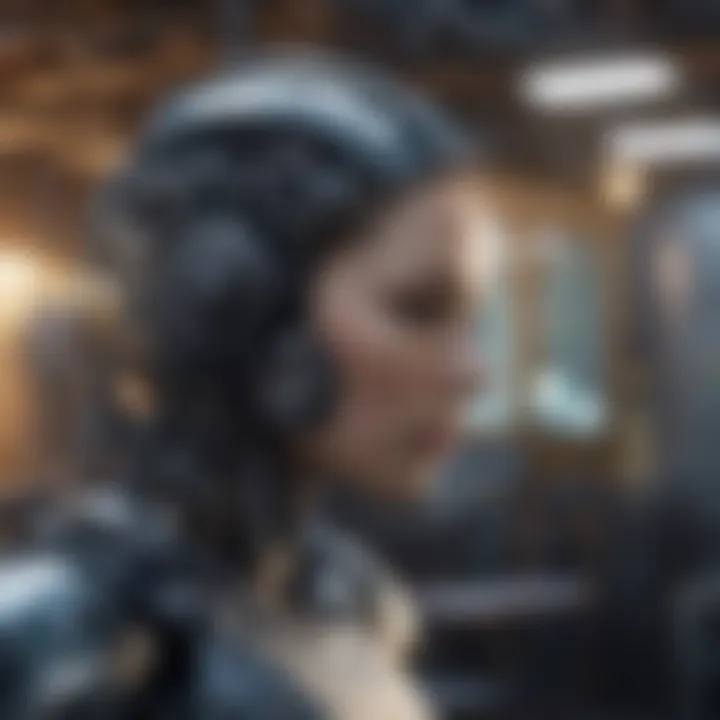
Researchers must work to ensure that their algorithms are not only effective but also equitable. This often involves:
- Bias Detection: Continuously identifying and correcting for biases in training data.
- Transparency: Making AI systems more understandable to users, thereby increasing trust.
- Inclusive Design: Engaging diverse groups in the development of AI technologies.
By actively addressing these ethical challenges, researchers contribute to the creation of a more inclusive AI landscape that prioritizes human values.
"The future of AI depends not only on technological innovation but also on our ability to guide it ethically."
Data Privacy Issues
Data privacy also represents a significant hurdle in AI research. As AI systems rely heavily on vast amounts of data, concerns arise regarding how this data is collected, stored, and used. There is a fine line between leveraging data to improve AI models and infringing on individuals' privacy.
Key elements to consider include:
- Data Ownership: Who owns the data used in training AI models? This question can lead to complex legal and ethical situations.
- Regulations: Compliance with laws such as GDPR is essential for researchers to avoid legal repercussions and ensure ethical use of data.
- User Consent: Effectively obtaining and managing consent from data subjects is critical to maintaining trust.
Balancing the need for data with the imperative for privacy protection is an ongoing challenge for AI researchers. It demands careful consideration and proactive strategies to mitigate risks associated with data handling.
Addressing these challenges is not merely an academic concern; it shapes the trajectory of future AI development and its role in society.
Future Directions in AI Research
The future of artificial intelligence research presents a landscape full of potential and pivot points that could alter our technological and social fabric. Understanding these future directions is essential for grasping how AI will evolve and influence various domains. From advancements in algorithm complexity to the ethical implementation of AI in society, the discussions about future directions encapsulate both benefits and challenges.
Emerging Trends
In the realm of AI research, several emerging trends are gaining traction. One significant trend involves the integration of AI with other advanced technologies such as quantum computing. Quantum computing possesses the capability to handle data at unprecedented speeds, thus potentially revolutionizing AI computations and expanding their applications.
Additionally, a growing focus is on explainable AI (XAI). This trend addresses the need to demystify AI decision-making processes. As AI systems are increasingly utilized in sectors like healthcare and finance, transparency becomes vital to ensure accountability and trust in these systems. Researchers are working to create models that can provide clear reasoning behind their conclusions.
Other notable trends include the expansion of AI ethics as a formal research branch. Given the ethical implications surrounding AI, including bias and privacy concerns, there is a pressing need for frameworks that ensure responsible use of AI technologies.
Potential Breakthroughs
The quest for breakthroughs in AI research is relentless. One potential area is the development of generalized AI, also known as Artificial General Intelligence (AGI). This would represent a significant leap from current AI capabilities, allowing systems to perform any intellectual task a human can do. While the journey to AGI is complex and fraught with challenges, its realization could unlock limitless possibilities across industries.
Another potential breakthrough lies in advancements of neural networks. Researchers aim to create more sophisticated architectures that can better mimic human brain functions. Innovations like capsule networks or those utilizing attention mechanisms are examples of routes being explored to achieve higher efficiency and accuracy in learning patterns.
Furthermore, harnessing large sets of unstructured data stands as a frontier. Techniques in unsupervised and semi-supervised learning are evolving. By improving our ability to extract insights from vast, unlabeled datasets, researchers can enhance machine intelligence and create more adaptable systems.
"The future of AI lies not merely in better algorithms but also in a combined understanding of ethics, technology, and societal impact."
The transition into these future pathways requires collaboration across disciplines. It is through a multifaceted approach that we can address technical challenges while ensuring that AI tools are safe and beneficial for society.
The Role of Education in Advancing AI
Education plays a critical role in the progress of artificial intelligence. As the field of AI evolves, so does the need for a well-structured curriculum that reflects current trends and technologies. The depth of knowledge acquired through education can shape the next generation of AI researchers and practitioners, equipping them with the tools to tackle challenges in this rapidly advancing domain.
Moreover, the importance of fostering an academic environment that encourages innovation cannot be overstated. By understanding the underpinning theories and methodologies in AI, students and researchers can contribute to groundbreaking advancements. Educational institutions also serve as a breeding ground for interdisciplinary collaboration, which is vital in solving complex issues faced by the AI community.
Curriculum Development
The development of a robust curriculum dedicated to AI is essential for preparing students for careers in this field. A well-rounded syllabus should encompass computer science fundamentals along with specialized AI topics, such as machine learning, neural networks, and natural language processing. The integration of practical projects is equally important, enabling students to apply theoretical concepts to real-world scenarios.
Some key considerations for curriculum development include:
- Industry Relevance: Collaborating with industry experts can help ensure that the curriculum remains aligned with current trends and skills demanded in the workforce.
- Core Competencies: The focus should revolve around essential topics, including algorithm design, data analysis, and ethical implications of AI.
- Interdisciplinary Approach: Encouraging students to take courses in related fields such as psychology, philosophy, and statistics will enhance their understanding of AI from diverse perspectives.
Incorporating these elements into the educational framework will cultivate a skilled workforce ready to contribute to the advances in AI.
Importance of Research Funding
Research funding is undoubtedly a vital element in advancing AI. Financial support can facilitate exploratory projects, allowing researchers to delve into innovative ideas that may lead to significant discoveries. High-quality research requires resources for both human capital and infrastructure.
Considerations regarding research funding include:
- Government Support: Public funding is often crucial for basic research that might not have immediate commercial applications.
- Private Investments: Tech companies frequently fund research initiatives to drive innovations that align with their strategic goals.
- Grants and Scholarships: Opportunities provided by various organizations can empower both established researchers and students to focus on AI advancement without financial burdens.
Having sufficient funding can greatly enhance the capability of researchers to explore ambitious projects, thus pushing the limits of what AI can achieve.
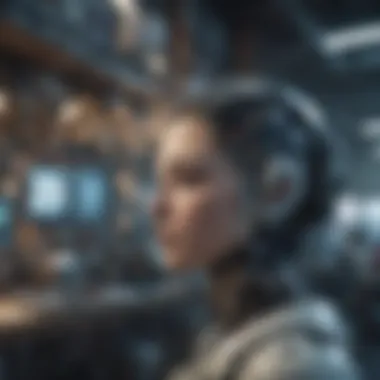
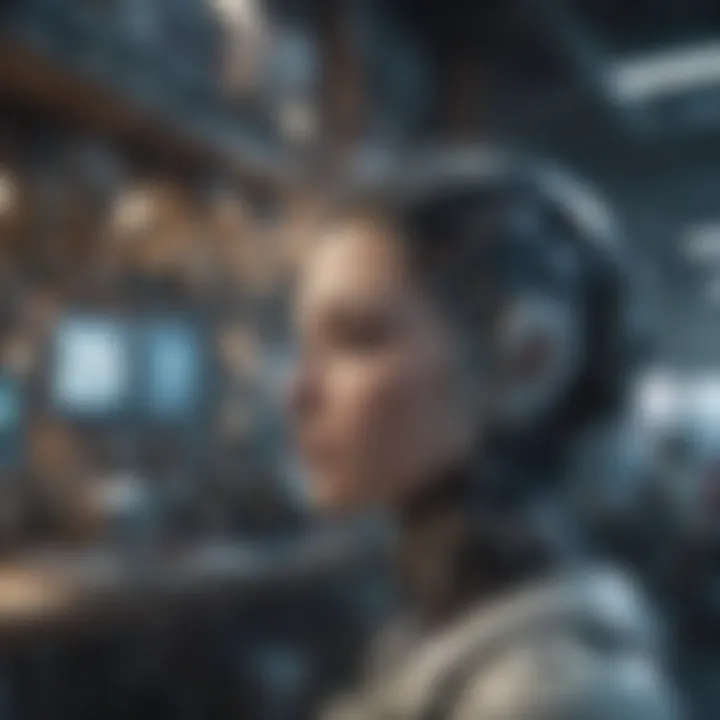
AI Research and Global Challenges
The convergence of artificial intelligence and global challenges is an increasingly crucial topic. As AI capabilities expand, their potential to address pressing issues like climate change and healthcare innovations becomes more apparent. Researchers and practitioners are recognizing that AI can play a vital role in devising solutions that meet humanitarian and environmental demands.
AI methodologies can optimize responses to crises, enhance predictive analytics, and streamline decision-making processes. This article highlights the significance of exploring how AI research can contribute to solutions for these global challenges, focusing on concrete examples of successful applications.
Moreover, understanding the implications of AI in these areas not only provides insights into future directions but also raises ethical considerations and highlights the importance of interdisciplinary collaboration. The interaction between AI technology and global issues fosters a sense of responsibility among researchers to ensure their innovations benefit society at large.
Addressing Climate Change
AI has emerged as a critical tool in the fight against climate change. Machine learning algorithms analyze vast amounts of environmental data, identifying patterns that inform mitigation strategies. For instance, AI models can predict greenhouse gas emissions and track their sources. These insights enable policymakers to implement more effective strategies for reduction.
Some significant areas include:
- Resource Optimization: AI can optimize energy consumption in buildings or transportation systems, thus reducing carbon footprints.
- Climate Modeling: Advanced AI techniques improve the accuracy of climate models, allowing scientists to simulate various scenarios of climate impact.
- Sustainable Agriculture: AI-driven analytics can assist farmers in making data-informed decisions that lead to increased crop yields while minimizing resource use.
Globally, institutions are harnessing AI for climate research. Teams at NASA and other organizations are using machine learning to analyze satellite data, identifying changes in land use and carbon levels. This cooperation signifies a broader acknowledgment of AI's potential in environmental stewardship.
Healthcare Innovations
The healthcare field is another domain where AI is making significant strides. AI technologies are revolutionizing patient care, diagnostics, and operational efficiencies. Machine learning algorithms can analyze medical images more accurately than human counterparts, resulting in early detection of conditions like cancer.
Some innovative applications of AI in healthcare include:
- Predictive Analytics: AI systems can analyze patient data to foresee health complications, allowing for preventive measures.
- Telemedicine: AI-powered chatbots and virtual assistants enhance patient engagement and streamline administrative tasks.
- Drug Discovery: AI accelerates the process of discovering new medications by simulating how different compounds interact with biological systems.
Organizations like Google Health and IBM Watson Health are spearheading efforts in using AI to improve health outcomes and operational efficiencies. The incorporation of AI into healthcare can ultimately lead to increased accessibility and affordability of services.
AI's contributions in these sectors underscore its transformational potential in tackling some of humanity’s most pressing challenges. The importance of AI research cannot be overstated as it positions itself as a powerful ally for future advancements.
Profiles of Key AI Figures
The examination of prominent figures in artificial intelligence is critical for understanding the trajectory of research and its eventual impact on society. Each leading figure contributes unique insights, methodologies, and breakthroughs in their respective fields. By analyzing their profiles, we gain valuable perspective on the driving forces behind modern AI innovations and the ethical implications of their work. This section spotlights the essential roles that these researchers play in shaping AI technologies and the collaborative nature of their research efforts.
Ethics in AI by Timnit Gebru
Timnit Gebru is a significant figure in AI ethics, known for her pioneering work in the realm of algorithmic bias and data privacy. As a co-founder of the Black in AI organization, she advocates for diversity and inclusion within the AI research community. Gebru’s research highlights how biases can manifest in AI systems, often leading to unfair outcomes that disproportionately affect marginalized groups.
Her publication, "Algorithmic Bias Detecting and Mitigation: Best Practices and Policies," emphasizes the need for transparency and accountability in AI development. This work urges researchers to consider ethical implications at every stage of the AI lifecycle. By leading discussions on fairness, Gebru contributes to the essential dialogue around responsible AI deployment, making her a key influencer in fostering a more ethical AI landscape.
Deep Learning Advancements by Yann LeCun
Yann LeCun has been instrumental in the development of deep learning, contributing foundational knowledge and innovative techniques. As Chief AI Scientist at Meta Platforms, LeCun’s research focuses on convolutional neural networks (CNNs) and their applications in image recognition and computer vision. His work paved the way for machines to interpret and classify images with remarkable accuracy.
LeCun's enthusiasm for unsupervised learning also sets him apart. He posits that AI systems should learn similarly to humans, utilizing vast amounts of unlabelled data. His pioneering approaches have influenced both academia and industries, establishing a roadmap for future deep learning advancements.
"The road to AI involves learning from data, but in ways that mimic human thought processes." - Yann LeCun
Natural Language Processing by Geoffrey Hinton
Geoffrey Hinton is a luminary in the field of natural language processing (NLP). Often referred to as one of the "Godfathers of Deep Learning," Hinton’s contributions extend from foundational theories to practical applications in understanding human language. His early work on backpropagation laid the groundwork for many state-of-the-art algorithms used in NLP today.
At Google, Hinton has worked on integrating neural networks with large datasets to enhance language understanding and generation capabilities. His research led to significant improvements in machine translation and conversational agents, impacting how humans and machines interact. Hinton advocates for a research culture that emphasizes collaboration and open sharing of ideas, thus advancing the entire field.
In summary, the profiles of these key figures in AI research illustrate the profound impact of their individual contributions. Their efforts not only propel technological advancements but also encourage ethical and responsible practices within the AI community. Evaluating their work provides a framework for navigating the complexities of AI technologies and their implications for society.
The End and Reflections on AI Research
The conclusion of this article serves as a pivotal moment to reflect on the vast and intricate landscape of artificial intelligence research. AI is not just about technology; it encompasses a deep understanding of the ethics, applications, and future potential of this rapidly evolving field. The contributions of leading figures in AI significantly shape both the theoretical frameworks and practical innovations that drive advancements today.
Understanding the profiles and work of prominent researchers allows us to grasp not only their impact within the scientific community but also their influence on society at large. This insight reveals the interconnectedness of their findings with pressing global challenges, thereby emphasizing the relevance of AI across various sectors.
Summary of Key Insights
Throughout this exploration, it becomes evident that:
- Diverse Expertise: AI research thrives on the collaboration of various disciplines, ranging from computer science and mathematics to psychology and philosophy.
- Ethical Imperatives: There is a growing consensus on the importance of integrating ethical considerations in AI development, highlighting the work of scholars like Timnit Gebru.
- Innovative Breakthroughs: Pioneering work, such as that by Geoffrey Hinton in natural language processing, illustrates how foundational research leads to transformative applications.
- Social Relevance: The potential of AI to affect issues like healthcare and climate change underscores the necessity of responsible innovation in the field.
"AI research is not merely a technological pursuit; it is a comprehensive dialogue about the future of humanity in relation to intelligent systems."
—Insights from leading AI researchers.
The Future Landscape of AI
Looking forward, the landscape of AI is poised for significant transformations. Several emerging trends stand out:
- Decentralized AI: Technologies like blockchain may enable more transparent and secure AI models.
- Increased Personalization: As algorithms evolve, we expect AI to provide increasingly tailored solutions for users, impacting sectors from entertainment to education.
- Human-Centric Design: Future AI systems are likely to prioritize user experience and ethical standards, addressing issues raised by critics of current AI practices.
- Cross-Disciplinary Collaboration: Innovations are likely to stem from cooperative efforts between computer scientists, social scientists, and ethicists, creating multifaceted solutions.
In summary, the reflections on AI research highlight the essential role of influential figures in shaping the present and future of AI. Understanding their contributions offers not only an appreciation of their work but also a lens through which we can examine the implications for society as a whole. As AI continues to evolve, staying informed about the influences, challenges, and potential of this technology will be crucial for anyone involved in or impacted by the field.